Relational Abstractions for Generalized Reinforcement Learning on Symbolic Problems
European Conference on Artificial Intelligence(2022)
摘要
Reinforcement learning in problems with symbolic state spaces is challenging due to the need for reasoning over long horizons. This paper presents a new approach that utilizes relational abstractions in conjunction with deep learning to learn a generalizable Q-function for such problems. The learned Q-function can be efficiently transferred to related problems that have different object names and object quantities, and thus, entirely different state spaces. We show that the learned generalized Q-function can be utilized for zero-shot transfer to related problems without an explicit, hand-coded curriculum. Empirical evaluations on a range of problems show that our method facilitates efficient zero-shot transfer of learned knowledge to much larger problem instances containing many objects.
更多查看译文
关键词
Machine Learning: Reinforcement Learning,Machine Learning: Deep Reinforcement Learning,Planning and Scheduling: Learning in Planning and Scheduling,Uncertainty in AI: Sequential Decision Making
AI 理解论文
溯源树
样例
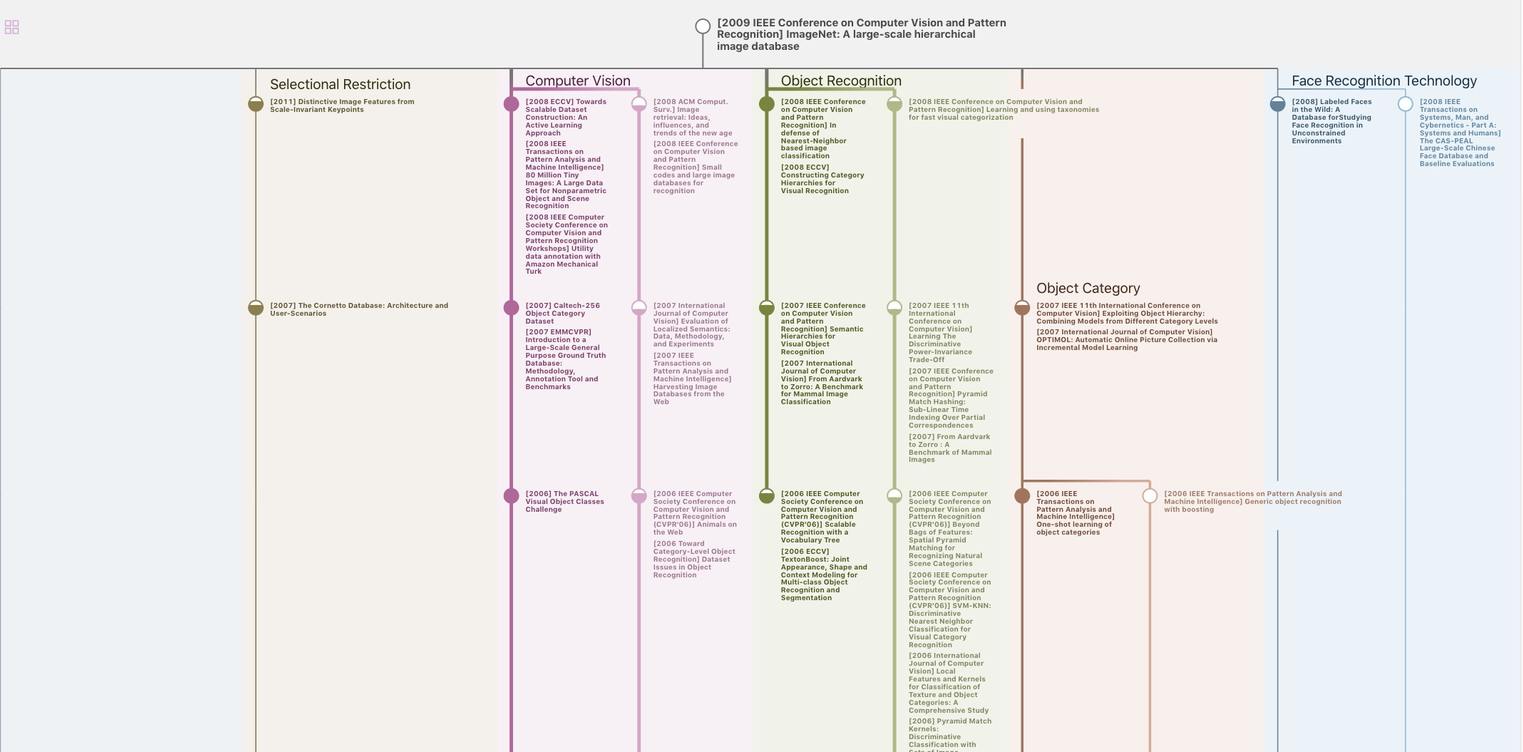
生成溯源树,研究论文发展脉络
Chat Paper
正在生成论文摘要