Phenomenology at the large hadron collider with deep learning: the case of vector-like quarks decaying to light jets
The European Physical Journal C(2022)
摘要
In this work, we continue our exploration of TeV-scale vector-like fermion signatures inspired by a Grand Unification scenario based on the trinification gauge group. A particular focus is given to pair-production topologies of vector-like quarks (VLQs) at the LHC, in a multi-jet plus a charged lepton and a missing energy signature. We employ Deep Learning methods and techniques based in evolutive algorithms that optimize hyper-parameters in the neural network construction, whose objective is to maximise the Asimov estimate for distinct VLQ masses. In this article, we consider the implications of an innovative approach by simultaneously combining detector images (also known as jet images) and tabular data containing kinematic information from the final states. With this technique we are able to exclude VLQs, that are specific for the considered model, up to a mass of 800 GeV in both the high-luminosity the Run-III phases of the LHC programme.
更多查看译文
关键词
quarks,large hadron collider,deep learning,vector-like
AI 理解论文
溯源树
样例
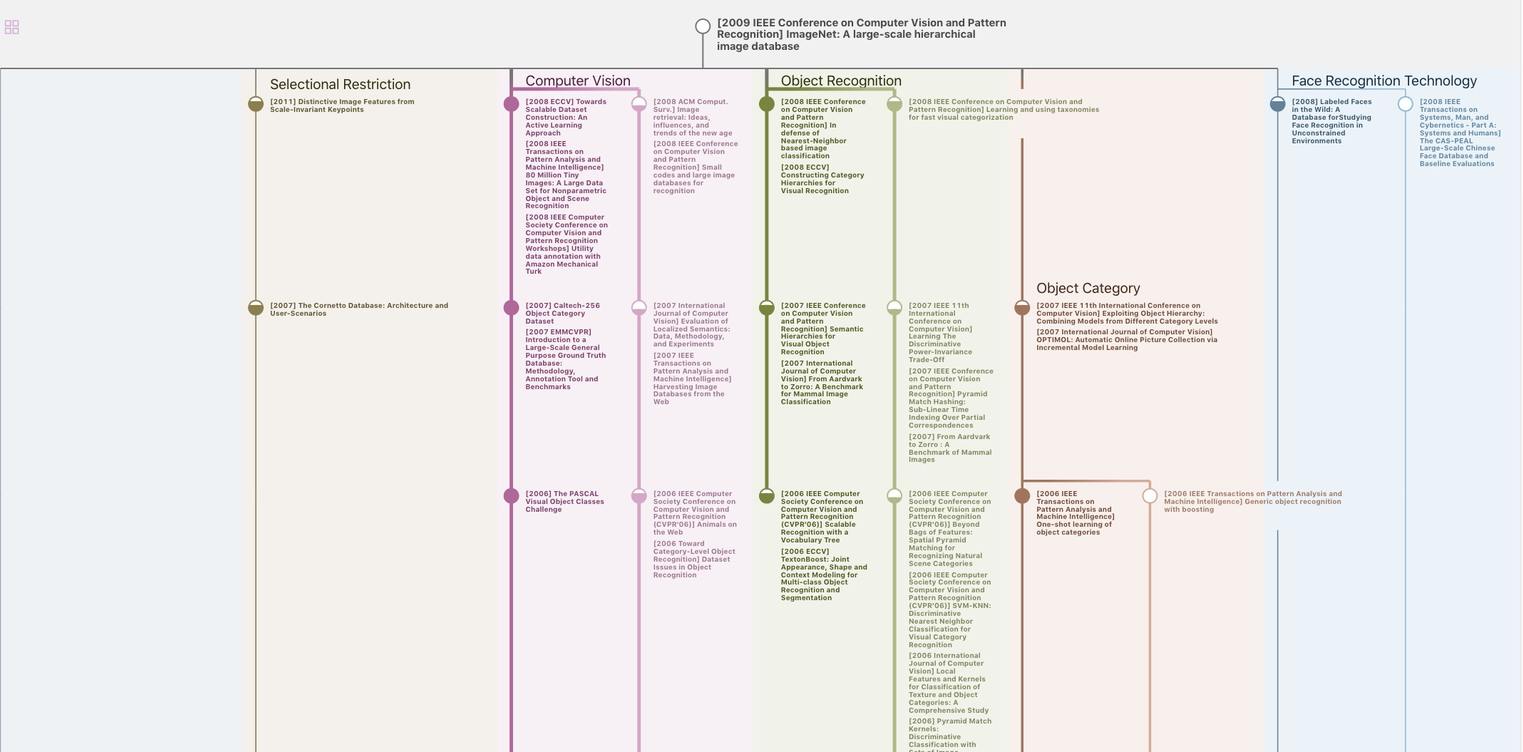
生成溯源树,研究论文发展脉络
Chat Paper
正在生成论文摘要