Contrastive Language-Action Pre-training for Temporal Localization
arxiv(2022)
摘要
Long-form video understanding requires designing approaches that are able to temporally localize activities or language. End-to-end training for such tasks is limited by the compute device memory constraints and lack of temporal annotations at large-scale. These limitations can be addressed by pre-training on large datasets of temporally trimmed videos supervised by class annotations. Once the video encoder is pre-trained, it is common practice to freeze it during fine-tuning. Therefore, the video encoder does not learn temporal boundaries and unseen classes, causing a domain gap with respect to the downstream tasks. Moreover, using temporally trimmed videos prevents to capture the relations between different action categories and the background context in a video clip which results in limited generalization capacity. To address these limitations, we propose a novel post-pre-training approach without freezing the video encoder which leverages language. We introduce a masked contrastive learning loss to capture visio-linguistic relations between activities, background video clips and language in the form of captions. Our experiments show that the proposed approach improves the state-of-the-art on temporal action localization, few-shot temporal action localization, and video language grounding tasks.
更多查看译文
关键词
localization,language-action,pre-training
AI 理解论文
溯源树
样例
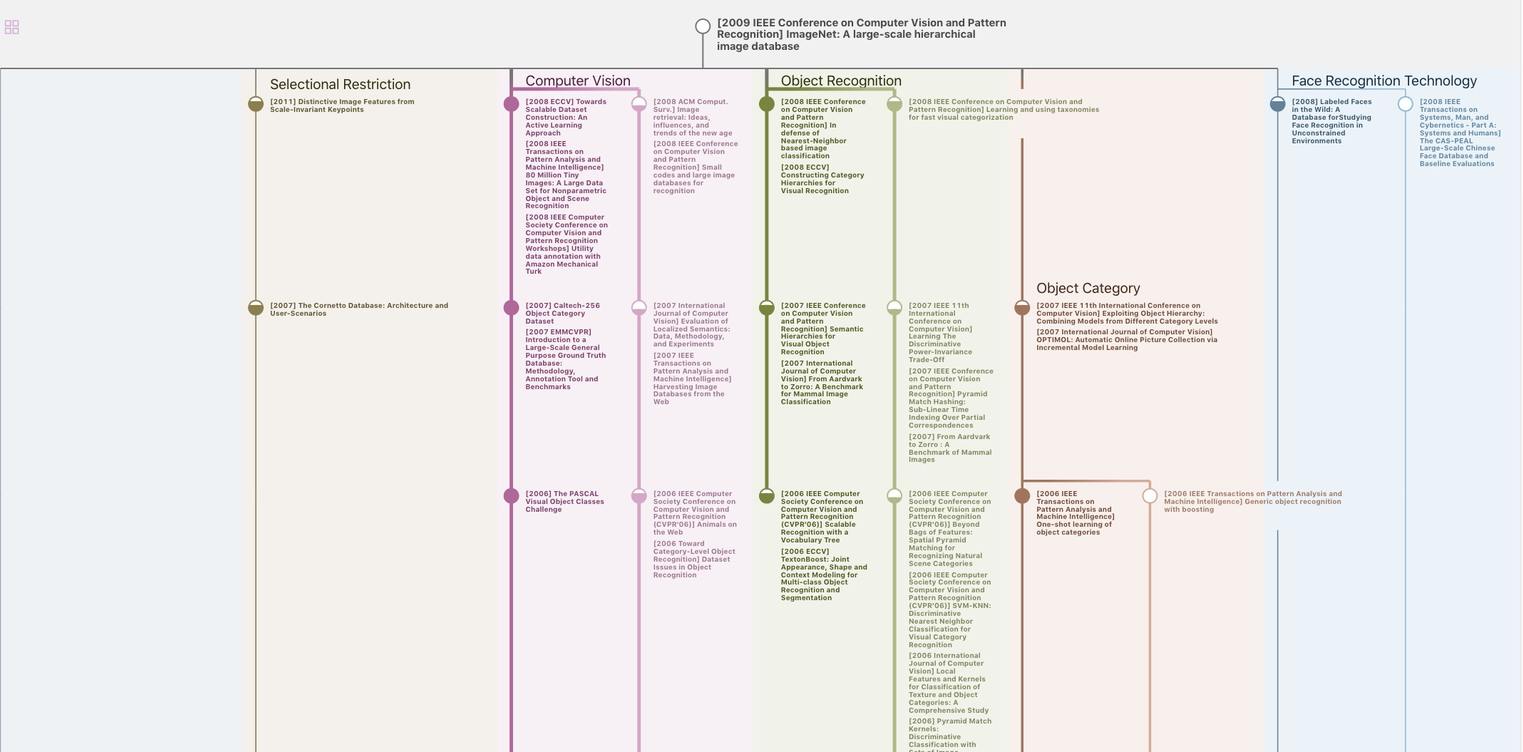
生成溯源树,研究论文发展脉络
Chat Paper
正在生成论文摘要