Information Fusion: Scaling Subspace-Driven Approaches
arxiv(2022)
摘要
In this work, we seek to exploit the deep structure of multi-modal data to robustly exploit the group subspace distribution of the information using the Convolutional Neural Network (CNN) formalism. Upon unfolding the set of subspaces constituting each data modality, and learning their corresponding encoders, an optimized integration of the generated inherent information is carried out to yield a characterization of various classes. Referred to as deep Multimodal Robust Group Subspace Clustering (DRoGSuRe), this approach is compared against the independently developed state-of-the-art approach named Deep Multimodal Subspace Clustering (DMSC). Experiments on different multimodal datasets show that our approach is competitive and more robust in the presence of noise.
更多查看译文
关键词
fusion,scaling,information,subspace-driven
AI 理解论文
溯源树
样例
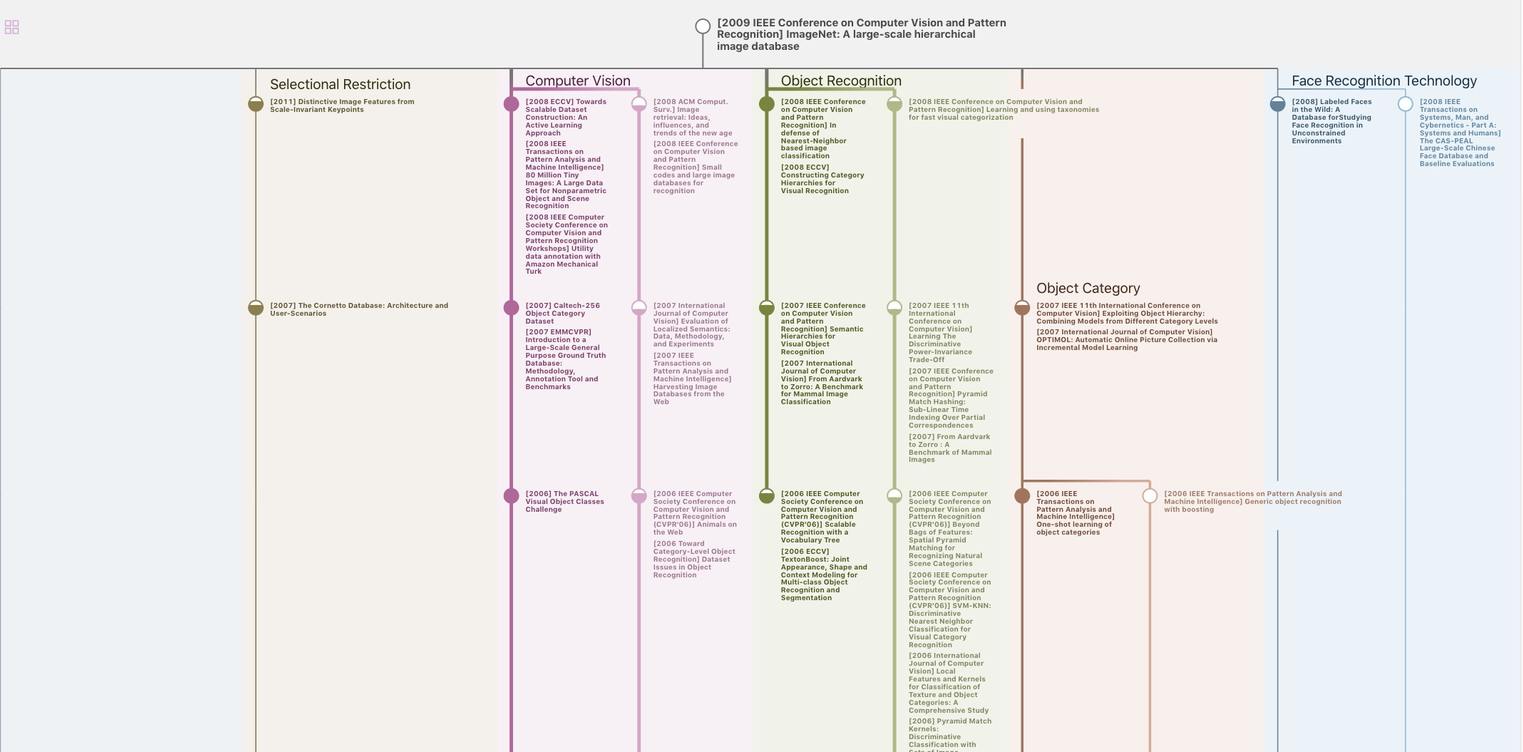
生成溯源树,研究论文发展脉络
Chat Paper
正在生成论文摘要