A Comparative Study of Data-Driven Models for Travel Destination Characterization
FRONTIERS IN BIG DATA(2022)
摘要
Characterizing items for content-based recommender systems is a challenging task in complex domains such as travel and tourism. In the case of destination recommendation, no feature set can be readily used as a similarity ground truth, which makes it hard to evaluate the quality of destination characterization approaches. Furthermore, the process should scale well for many items, be cost-efficient, and most importantly correct. To evaluate which data sources are most suitable, we investigate 18 characterization methods that fall into three categories: venue data, textual data, and factual data. We make these data models comparable using rank agreement metrics and reveal which data sources capture similar underlying concepts. To support choosing more suitable data models, we capture a desired concept using an expert survey and evaluate our characterization methods toward it. We find that the textual models to characterize cities perform best overall, with data models based on factual and venue data being less competitive. However, we show that data models with explicit features can be optimized by learning weights for their features.
更多查看译文
关键词
destination characterization, rank agreement metrics, expert evaluation, data mining, recommender systems, content-based filtering
AI 理解论文
溯源树
样例
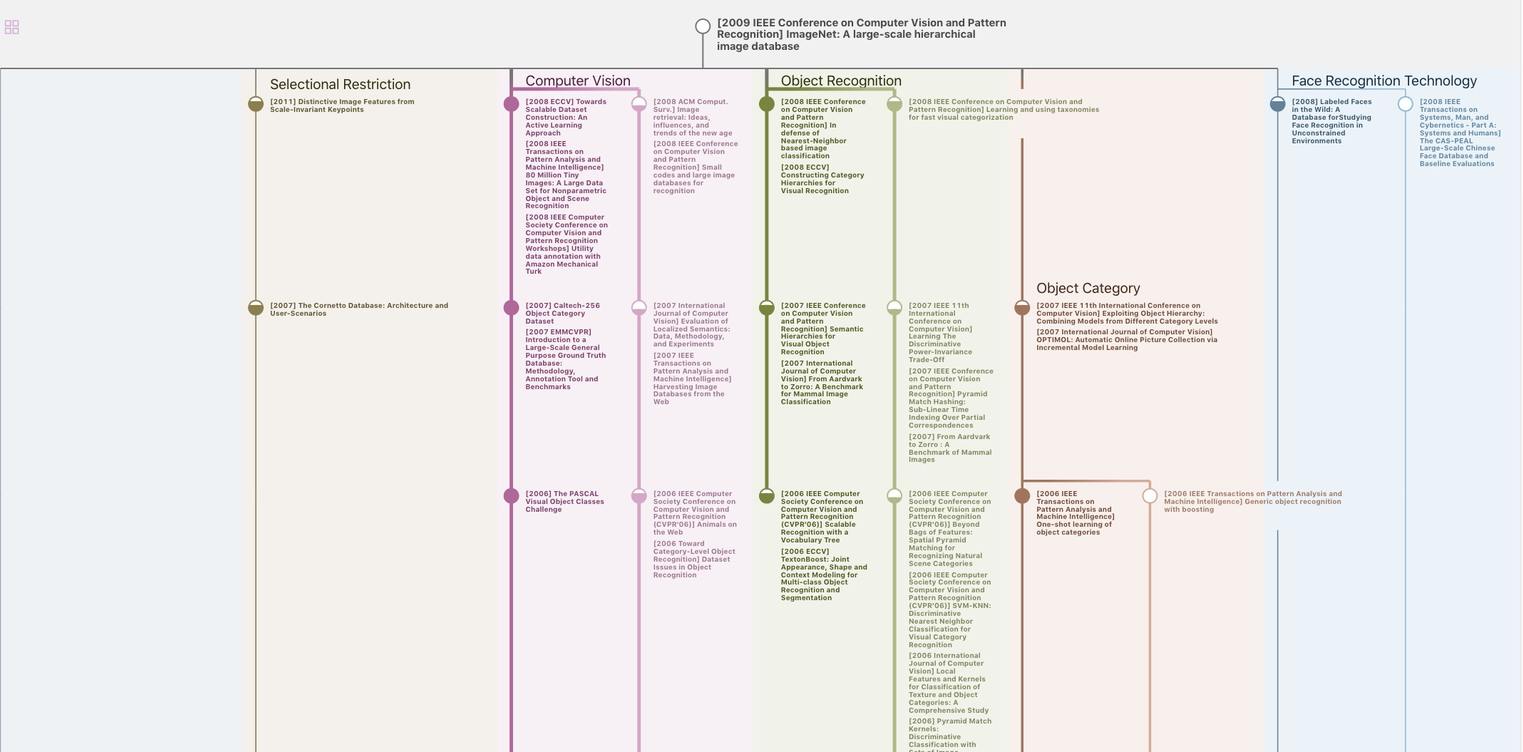
生成溯源树,研究论文发展脉络
Chat Paper
正在生成论文摘要