Conversational Question Answering on Heterogeneous Sources
SIGIR '22: Proceedings of the 45th International ACM SIGIR Conference on Research and Development in Information Retrieval(2022)
摘要
Conversational question answering (ConvQA) tackles sequential information needs where contexts in follow-up questions are left implicit. Current ConvQA systems operate over homogeneous sources of information: either a knowledge base (KB), or a text corpus, or a collection of tables. This paper addresses the novel issue of jointly tapping into all of these together, this way boosting answer coverage and confidence. We present CONVINSE, an end-to-end pipeline for ConvQA over heterogeneous sources, operating in three stages: i) learning an explicit structured representation of an incoming question and its conversational context, ii) harnessing this frame-like representation to uniformly capture relevant evidences from KB, text, and tables, and iii) running a fusion-in-decoder model to generate the answer. We construct and release the first benchmark, ConvMix, for ConvQA over heterogeneous sources, comprising 3000 real-user conversations with 16000 questions, along with entity annotations, completed question utterances, and question paraphrases. Experiments demonstrate the viability and advantages of our method, compared to state-of-the-art baselines.
更多查看译文
关键词
Conversations, Question Answering, Explainability
AI 理解论文
溯源树
样例
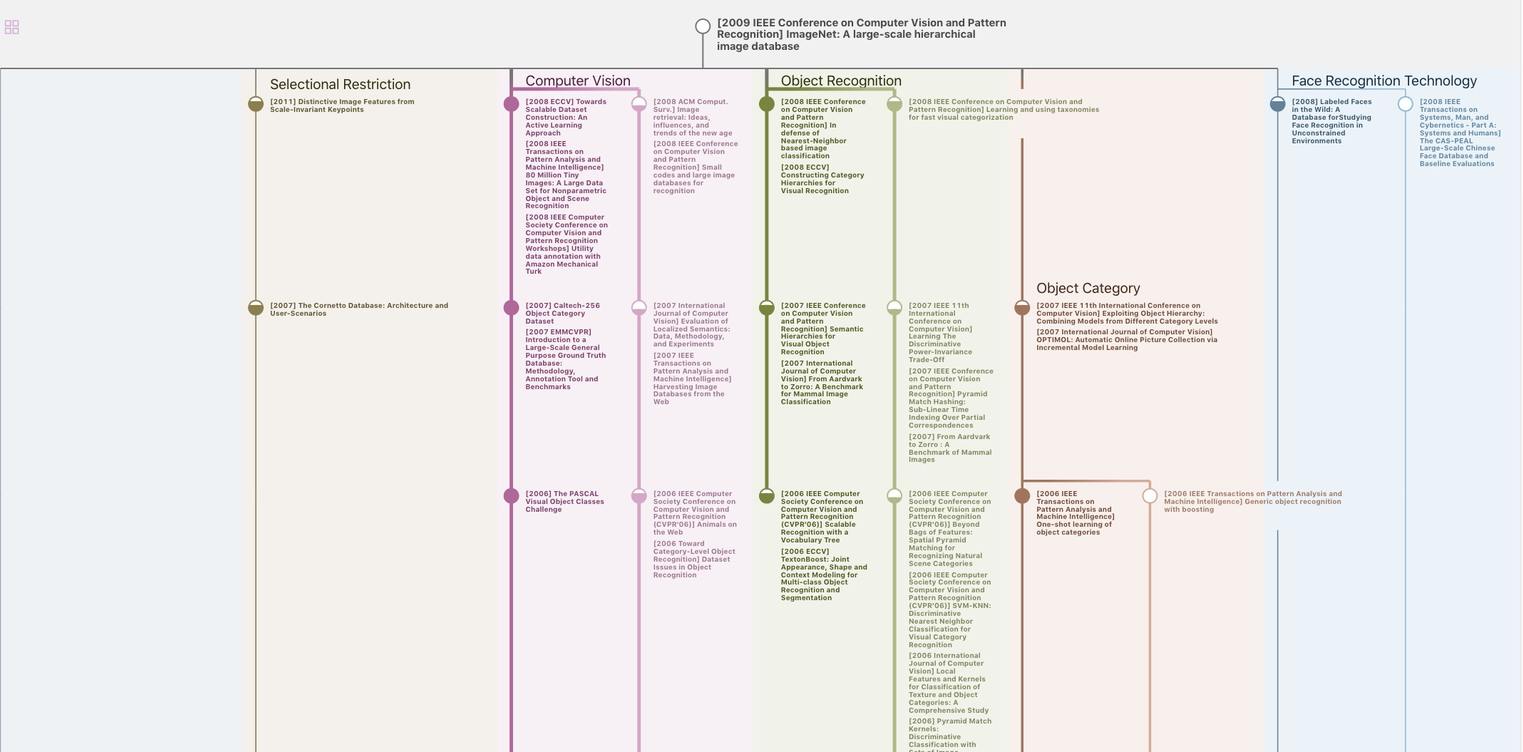
生成溯源树,研究论文发展脉络
Chat Paper
正在生成论文摘要