MCSE: Multimodal Contrastive Learning of Sentence Embeddings
North American Chapter of the Association for Computational Linguistics (NAACL)(2022)
摘要
Learning semantically meaningful sentence embeddings is an open problem in natural language processing. In this work, we propose a sentence embedding learning approach that exploits both visual and textual information via a multimodal contrastive objective. Through experiments on a variety of semantic textual similarity tasks, we demonstrate that our approach consistently improves the performance across various datasets and pre-trained encoders. In particular, combining a small amount of multimodal data with a large text-only corpus, we improve the state-of-the-art average Spearman's correlation by 1.7%. By analyzing the properties of the textual embedding space, we show that our model excels in aligning semantically similar sentences, providing an explanation for its improved performance.
更多查看译文
关键词
multimodal contrastive learning
AI 理解论文
溯源树
样例
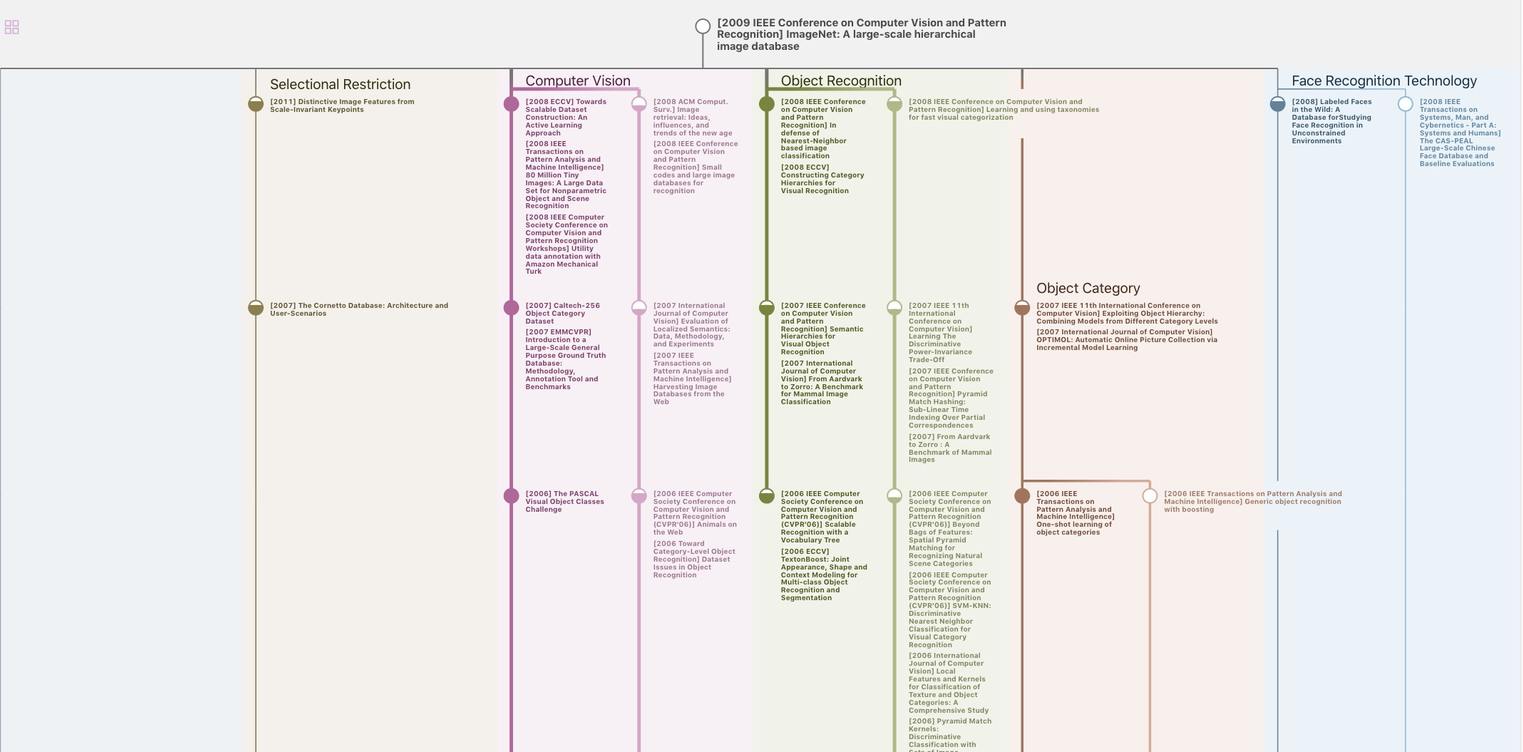
生成溯源树,研究论文发展脉络
Chat Paper
正在生成论文摘要