Locally Aggregated Feature Attribution on Natural Language Model Understanding
North American Chapter of the Association for Computational Linguistics (NAACL)(2022)
摘要
With the growing popularity of deep-learning models, model understanding becomes more important. Much effort has been devoted to demystify deep neural networks for better interpretability. Some feature attribution methods have shown promising results in computer vision, especially the gradient-based methods where effectively smoothing the gradients with reference data is key to a robust and faithful result. However, direct application of these gradient-based methods to NLP tasks is not trivial due to the fact that the input consists of discrete tokens and the "reference" tokens are not explicitly defined. In this work, we propose Locally Aggregated Feature Attribution (LAFA), a novel gradient-based feature attribution method for NLP models. Instead of relying on obscure reference tokens, it smooths gradients by aggregating similar reference texts derived from language model embeddings. For evaluation purpose, we also design experiments on different NLP tasks including Entity Recognition and Sentiment Analysis on public datasets as well as key feature detection on a constructed Amazon catalogue dataset. The superior performance of the proposed method is demonstrated through experiments.
更多查看译文
关键词
aggregated feature attribution,natural language model
AI 理解论文
溯源树
样例
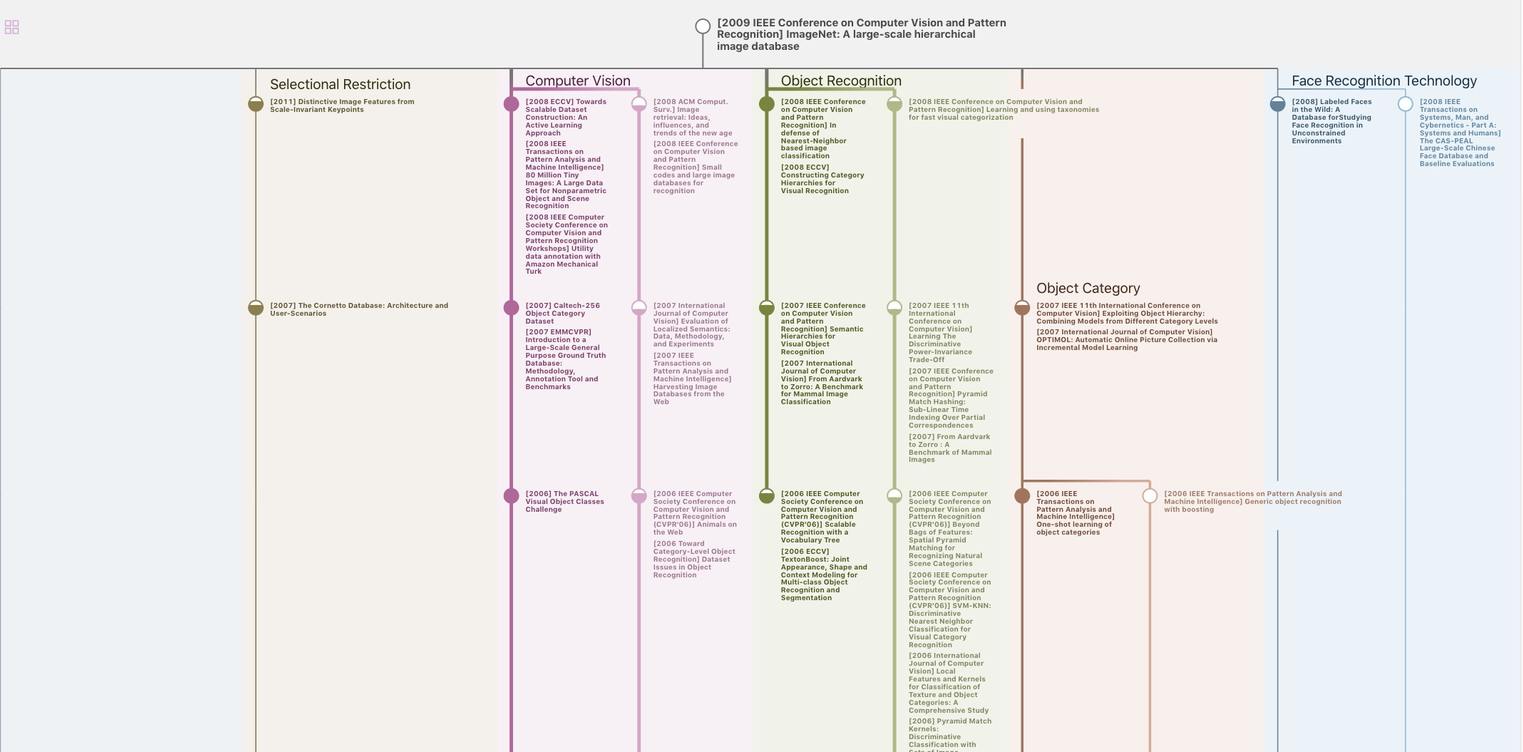
生成溯源树,研究论文发展脉络
Chat Paper
正在生成论文摘要