A Note on the Regularity of Images Generated by Convolutional Neural Networks
arxiv(2023)
摘要
The regularity of images generated by convolutional neural networks, such as the U-net, generative networks, or the deep image prior, is analyzed. In a resolution-independent, infinite dimensional setting, it is shown that such images, represented as functions, are always continuous and, in some circumstances, even continuously differentiable, contradicting the widely accepted modeling of sharp edges in images via jump discontinuities. While such statements require an infinite dimensional setting, the connection to (discretized) neural networks used in practice is made by considering the limit as the resolution approaches infinity. As practical consequence, the results of this paper in particular provide analytical evidence that basic L2 regularization of network weights might lead to over-smoothed outputs.
更多查看译文
关键词
convolutional neural networks, machine learning, functional analysis, mathematical imaging
AI 理解论文
溯源树
样例
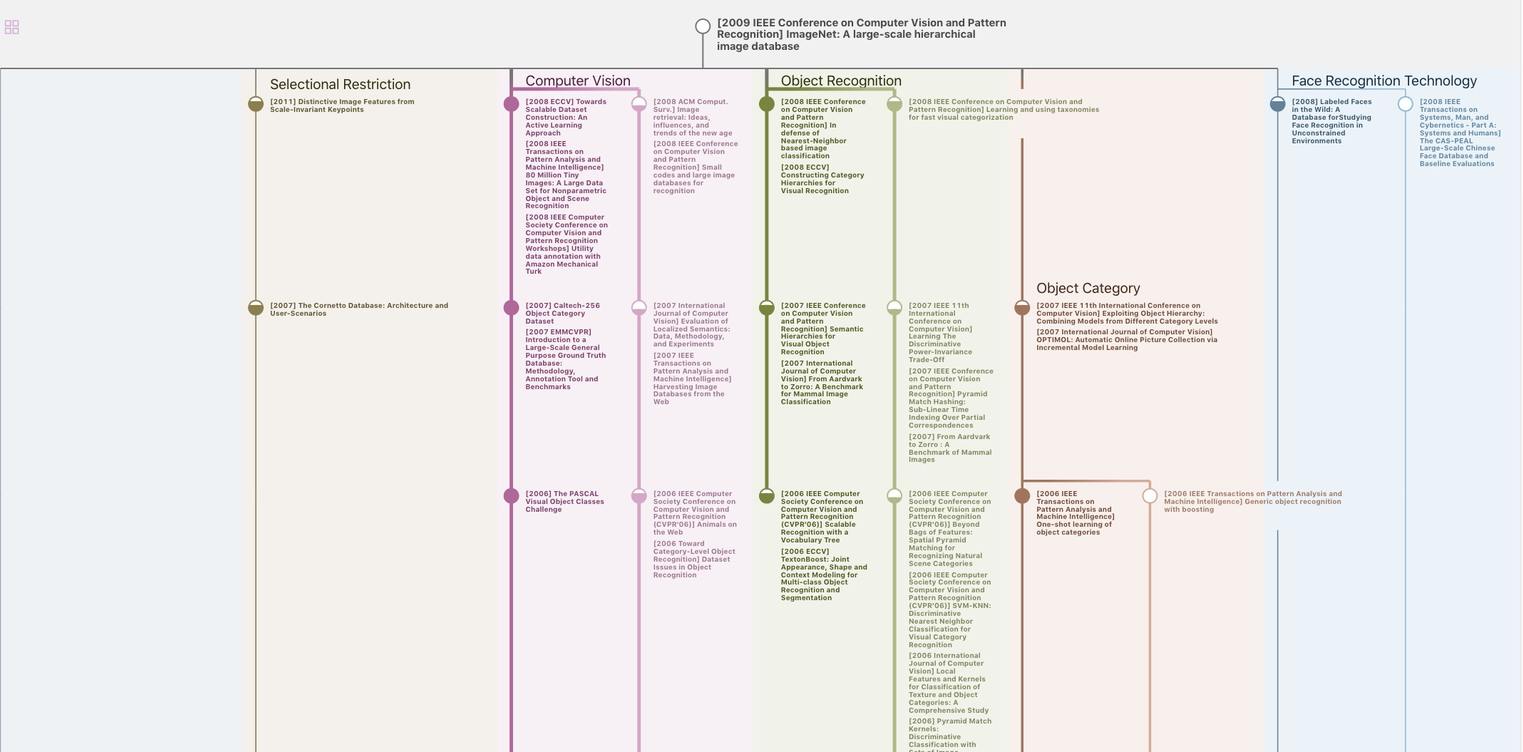
生成溯源树,研究论文发展脉络
Chat Paper
正在生成论文摘要