Learning Dynamic View Synthesis With Few RGBD Cameras
arxiv(2022)
摘要
There have been significant advancements in dynamic novel view synthesis in recent years. However, current deep learning models often require (1) prior models (e.g., SMPL human models), (2) heavy pre-processing, or (3) per-scene optimization. We propose to utilize RGBD cameras to remove these limitations and synthesize free-viewpoint videos of dynamic indoor scenes. We generate feature point clouds from RGBD frames and then render them into free-viewpoint videos via a neural renderer. However, the inaccurate, unstable, and incomplete depth measurements induce severe distortions, flickering, and ghosting artifacts. We enforce spatial-temporal consistency via the proposed Cycle Reconstruction Consistency and Temporal Stabilization module to reduce these artifacts. We introduce a simple Regional Depth-Inpainting module that adaptively inpaints missing depth values to render complete novel views. Additionally, we present a Human-Things Interactions dataset to validate our approach and facilitate future research. The dataset consists of 43 multi-view RGBD video sequences of everyday activities, capturing complex interactions between human subjects and their surroundings. Experiments on the HTI dataset show that our method outperforms the baseline per-frame image fidelity and spatial-temporal consistency. We will release our code, and the dataset on the website soon.
更多查看译文
关键词
dynamic view synthesis,few rgbd cameras,learning
AI 理解论文
溯源树
样例
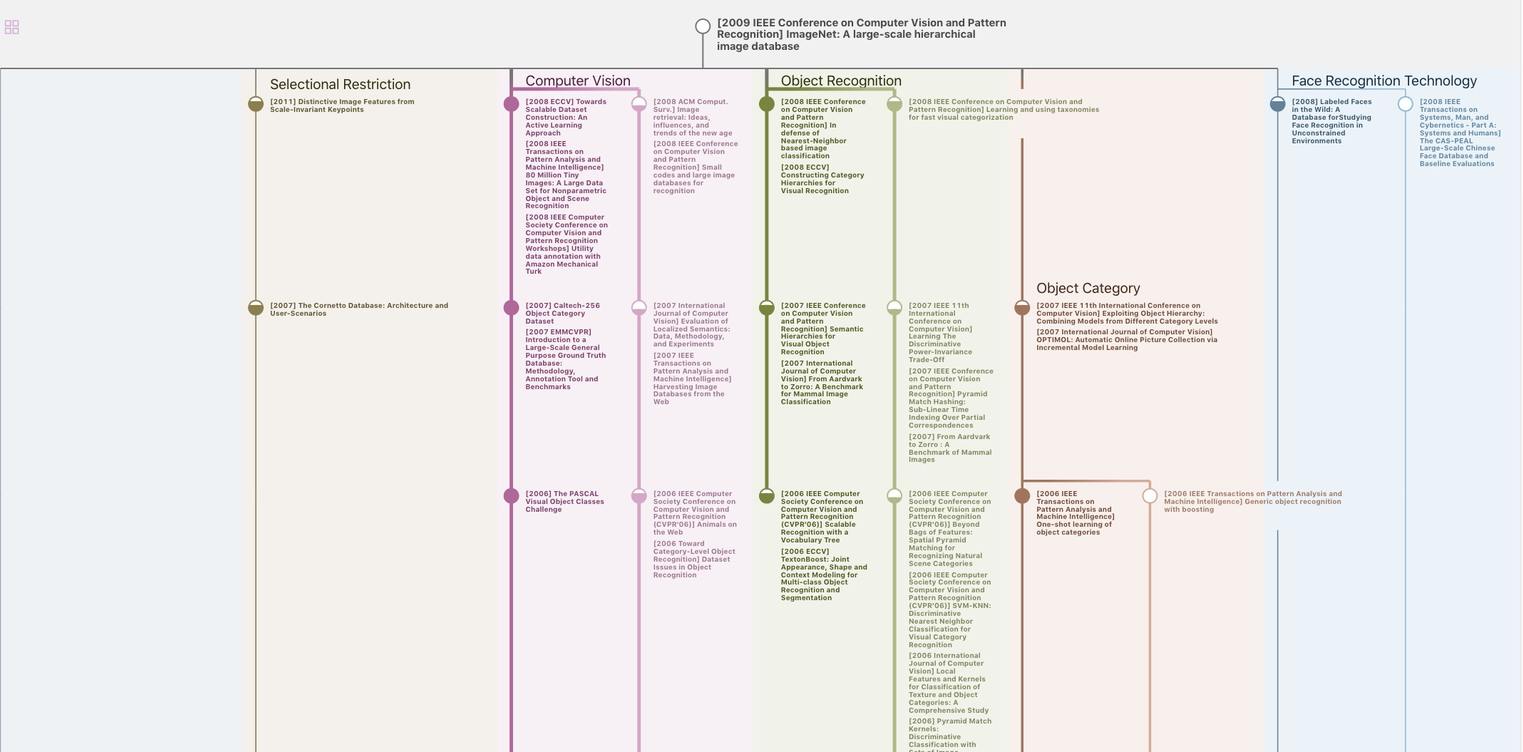
生成溯源树,研究论文发展脉络
Chat Paper
正在生成论文摘要