Improving the Generalizability of Depression Detection by Leveraging Clinical Questionnaires
PROCEEDINGS OF THE 60TH ANNUAL MEETING OF THE ASSOCIATION FOR COMPUTATIONAL LINGUISTICS (ACL 2022), VOL 1: (LONG PAPERS)(2022)
摘要
Automated methods have been widely used to identify and analyze mental health conditions (e.g., depression) from various sources of information, including social media. Yet, deployment of such models in real-world healthcare applications faces challenges including poor out-of-domain generalization and lack of trust in black box models. In this work, we propose approaches for depression detection that are constrained to different degrees by the presence of symptoms described in PHQ9, a questionnaire used by clinicians in the depression screening process. In dataset-transfer experiments on three social media datasets, we find that grounding the model in PHQ9's symptoms substantially improves its ability to generalize to out-of-distribution data compared to a standard BERT-based approach. Furthermore, this approach can still perform competitively on in-domain data. These results and our qualitative analyses suggest that grounding model predictions in clinically-relevant symptoms can improve generalizability while producing a model that is easier to inspect.
更多查看译文
关键词
depression detection
AI 理解论文
溯源树
样例
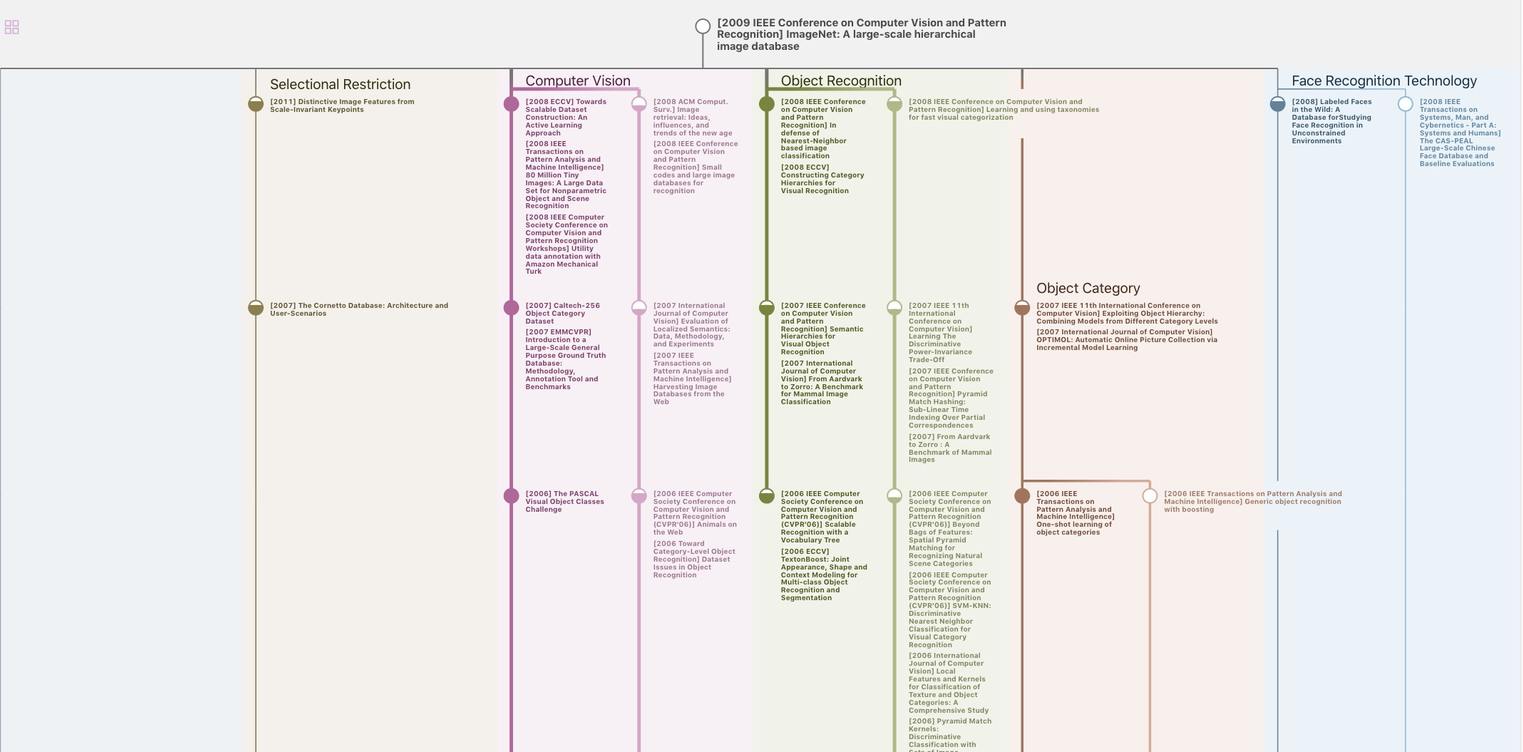
生成溯源树,研究论文发展脉络
Chat Paper
正在生成论文摘要