Implementation of a machine learning application in preoperative risk assessment for hip repair surgery
BMC Anesthesiology(2022)
摘要
Background This study aims to develop a machine learning-based application in a real-world medical domain to assist anesthesiologists in assessing the risk of complications in patients after a hip surgery. Methods Data from adult patients who underwent hip repair surgery at Chi-Mei Medical Center and its 2 branch hospitals from January 1, 2013, to March 31, 2020, were analyzed. Patients with incomplete data were excluded. A total of 22 features were included in the algorithms, including demographics, comorbidities, and major preoperative laboratory data from the database. The primary outcome was a composite of adverse events (in-hospital mortality, acute myocardial infarction, stroke, respiratory, hepatic and renal failure, and sepsis). Secondary outcomes were intensive care unit (ICU) admission and prolonged length of stay (PLOS). The data obtained were imported into 7 machine learning algorithms to predict the risk of adverse outcomes. Seventy percent of the data were randomly selected for training, leaving 30% for testing. The performances of the models were evaluated by the area under the receiver operating characteristic curve (AUROC). The optimal algorithm with the highest AUROC was used to build a web-based application, then integrated into the hospital information system (HIS) for clinical use. Results Data from 4,448 patients were analyzed; 102 (2.3%), 160 (3.6%), and 401 (9.0%) patients had primary composite adverse outcomes, ICU admission, and PLOS, respectively. Our optimal model had a superior performance (AUROC by DeLong test) than that of ASA-PS in predicting the primary composite outcomes (0.810 vs. 0.629, p < 0.01), ICU admission (0.835 vs. 0.692, p < 0.01), and PLOS (0.832 vs. 0.618, p < 0.01). Conclusions The hospital-specific machine learning model outperformed the ASA-PS in risk assessment. This web-based application gained high satisfaction from anesthesiologists after online use.
更多查看译文
关键词
Hip surgery, Risk assessment, Machine learning
AI 理解论文
溯源树
样例
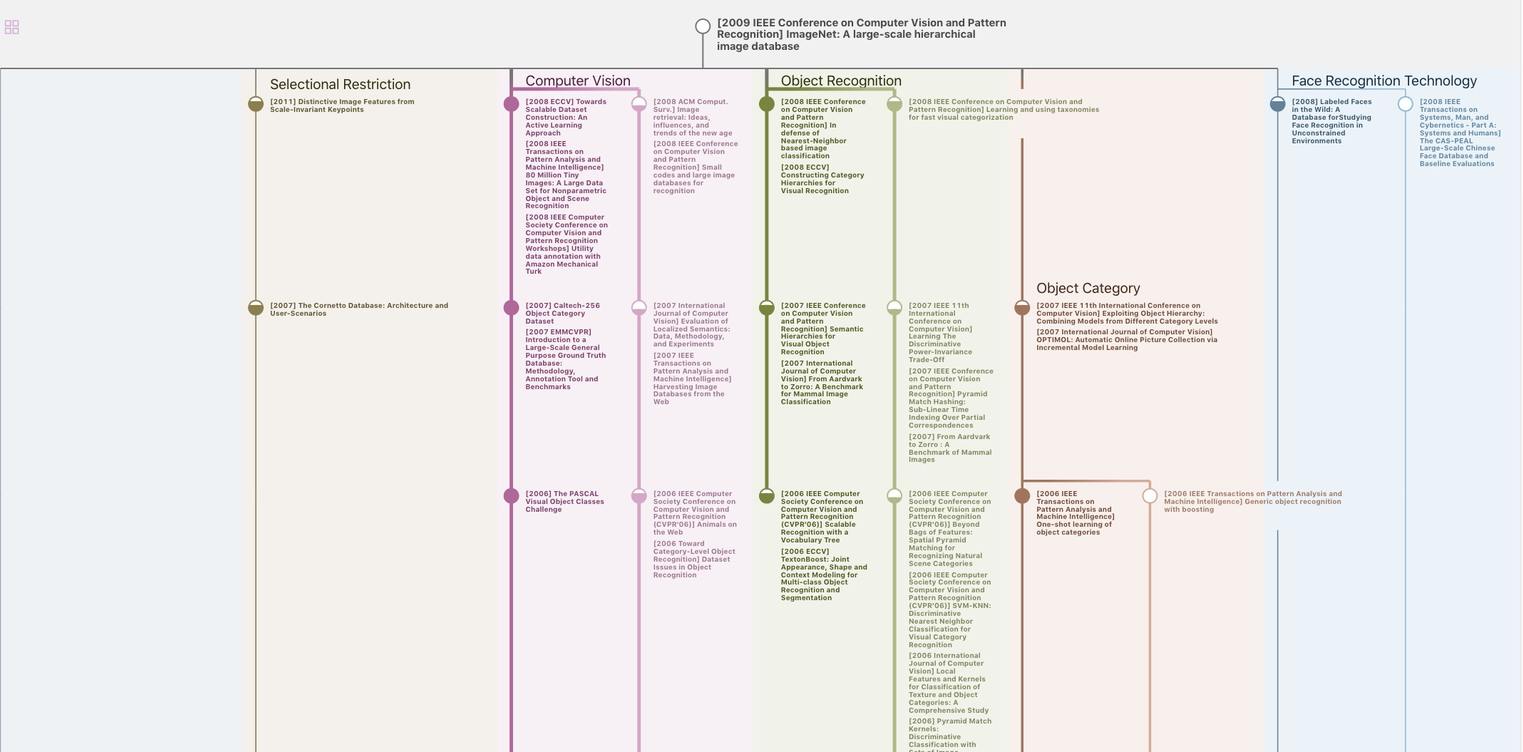
生成溯源树,研究论文发展脉络
Chat Paper
正在生成论文摘要