Decision Theory versus Conventional Statistics for Personalized Therapy of Breast Cancer
JOURNAL OF PERSONALIZED MEDICINE(2022)
摘要
Estrogen and progesterone receptors being present or not represents one of the most important biomarkers for therapy selection in breast cancer patients. Conventional measurement by immunohistochemistry (IHC) involves errors, and numerous attempts have been made to increase precision by additional information from gene expression. This raises the question of how to fuse information, in particular, if there is disagreement. It is the primary domain of Dempster-Shafer decision theory (DST) to deal with contradicting evidence on the same item (here: receptor status), obtained through different techniques. DST is widely used in technical settings, such as self-driving cars and aviation, and is also promising to deliver significant advantages in medicine. Using data from breast cancer patients already presented in previous work, we focus on comparing DST with classical statistics in this work, to pave the way for its application in medicine. First, we explain how DST not only considers probabilities (a single number per sample), but also incorporates uncertainty in a concept of 'evidence' (two numbers per sample). This allows for very powerful displays of patient data in so-called ternary plots, a novel and crucial advantage for medical interpretation. Results are obtained according to conventional statistics (ODDS) and, in parallel, according to DST. Agreement and differences are evaluated, and the particular merits of DST discussed. The presented application demonstrates how decision theory introduces new levels of confidence in diagnoses derived from medical data.
更多查看译文
关键词
biomarkers, decision theory, gene expression, breast cancer, receptor status, precision medicine, personalized medicine, data science, mathematical oncology
AI 理解论文
溯源树
样例
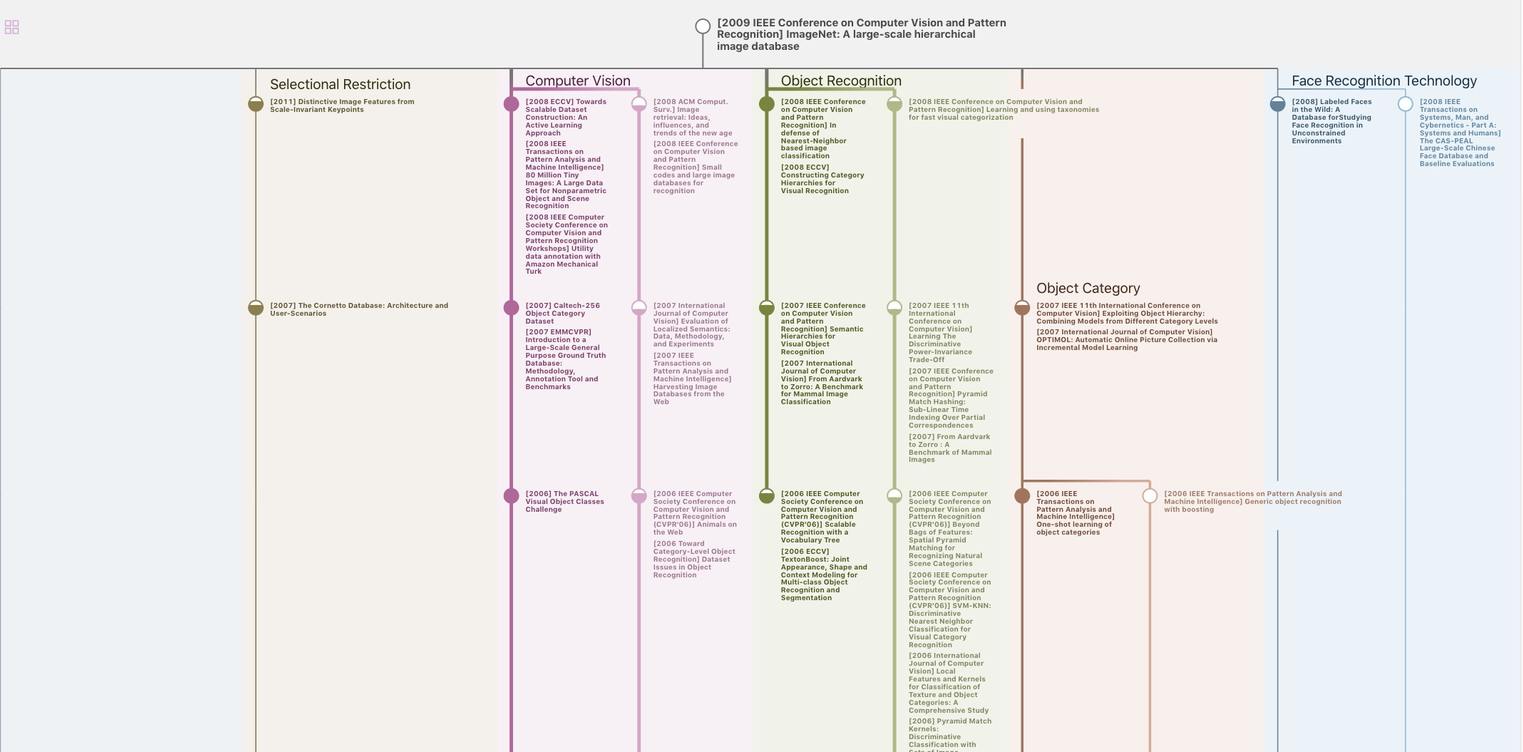
生成溯源树,研究论文发展脉络
Chat Paper
正在生成论文摘要