A Spatial–Temporal Graph Model for Pronunciation Feature Prediction of Chinese Poetry
IEEE transactions on neural networks and learning systems(2023)
摘要
With the development of artificial intelligence, speech recognition and prediction have become one of the important research domains with wild applications, such as intelligent control, education, individual identification, and emotion analysis. Chinese poetry reading contains rich features of continuous pronunciations, such as mood, emotion, rhythm schemes, lyric reading, and artistic expression. Therefore, the prediction of the pronunciation characteristics of a Chinese poetry reading is the significance for the presentation of high-level machine intelligence and has the potential to create a high-level intelligent system for teaching children to read Tang poetry. Mel frequency cepstral coefficient (MFCC) is currently used to present important speech features. Due to the complexity and high degree of nonlinearity in poetry reading, however, there is a tough challenge facing accurate pronunciation feature prediction, that is, how to model complex spatial correlations and time dynamics, such as rhyme schemes. As for many current methods, they ignore the spatial and temporal characteristics in MFCC presentation. In addition, these methods are subjected to certain limitations on prediction for long-term performance. In order to solve these problems, we propose a novel spatial–temporal graph model (STGM-MHA) based on multihead attention for the purpose of pronunciation feature prediction of Chinese poetry. The STGM-MHA is designed using an encoder–decoder structure. The encoder compresses the data into a hidden space representation, while the decoder reconstructs the hidden space representation as output. In the model, a novel gated recurrent unit (GRU) module (AGRU) based on multihead attention is proposed to extract the spatial and temporal features of MFCC data effectively. The evaluation comparison of our proposed model versus state-of-the-art methods in six datasets reveals the clear advantage of the proposed model.
更多查看译文
关键词
Mel frequency cepstral coefficient,Predictive models,Rhythm,Feature extraction,Analytical models,Data models,Computational modeling,AGRU,Chinese poetry,encoder-decoder,graph modeling,Mel frequency cepstral coefficient (MFCC),pronunciation features,spatial-temporal graph model (STGM-MHA)
AI 理解论文
溯源树
样例
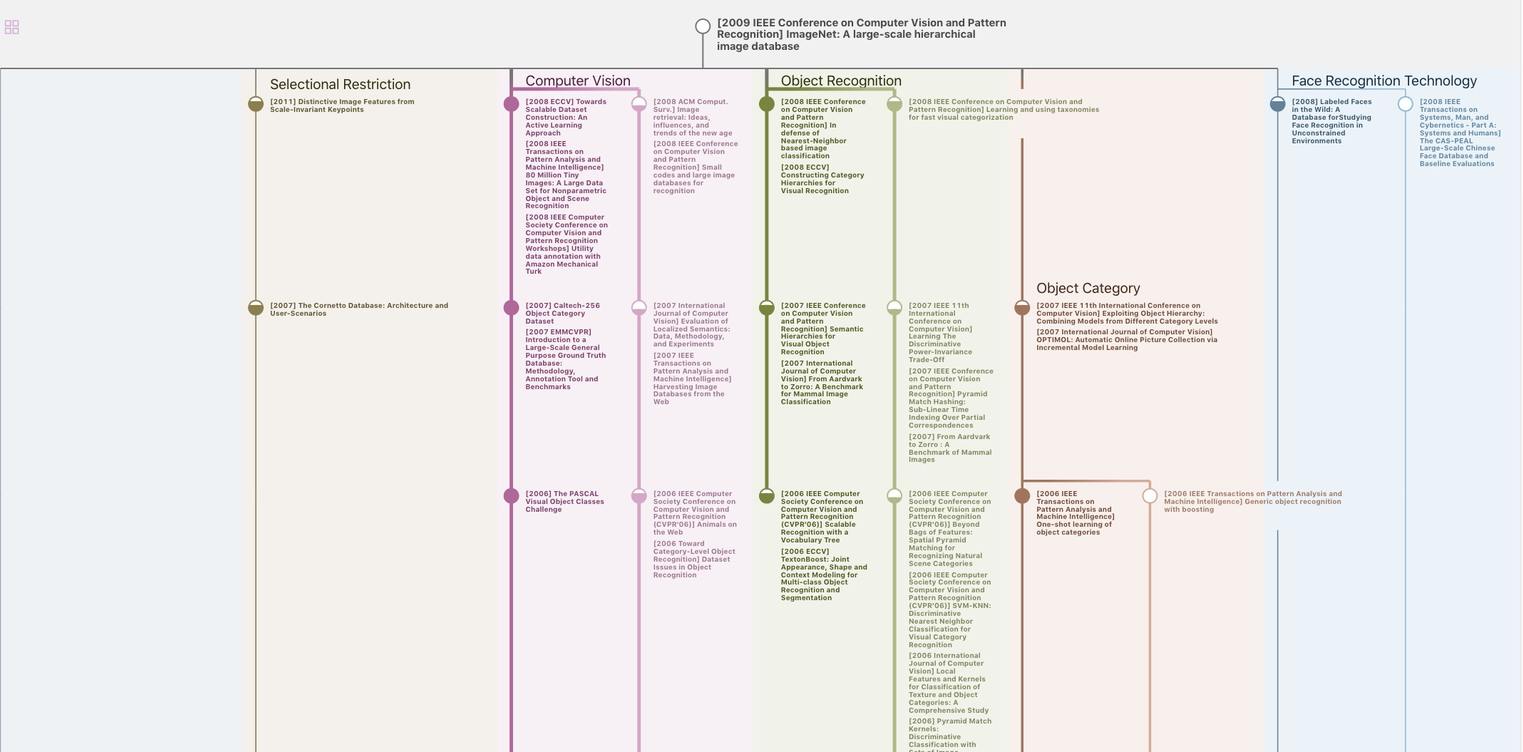
生成溯源树,研究论文发展脉络
Chat Paper
正在生成论文摘要