Adversarial Contrastive Learning by Permuting Cluster Assignments
arxiv(2022)
摘要
Contrastive learning has gained popularity as an effective self-supervised representation learning technique. Several research directions improve traditional contrastive approaches, e.g., prototypical contrastive methods better capture the semantic similarity among instances and reduce the computational burden by considering cluster prototypes or cluster assignments, while adversarial instance-wise contrastive methods improve robustness against a variety of attacks. To the best of our knowledge, no prior work jointly considers robustness, cluster-wise semantic similarity and computational efficiency. In this work, we propose SwARo, an adversarial contrastive framework that incorporates cluster assignment permutations to generate representative adversarial samples. We evaluate SwARo on multiple benchmark datasets and against various white-box and black-box attacks, obtaining consistent improvements over state-of-the-art baselines.
更多查看译文
关键词
adversarial contrastive learning,permuting cluster assignments
AI 理解论文
溯源树
样例
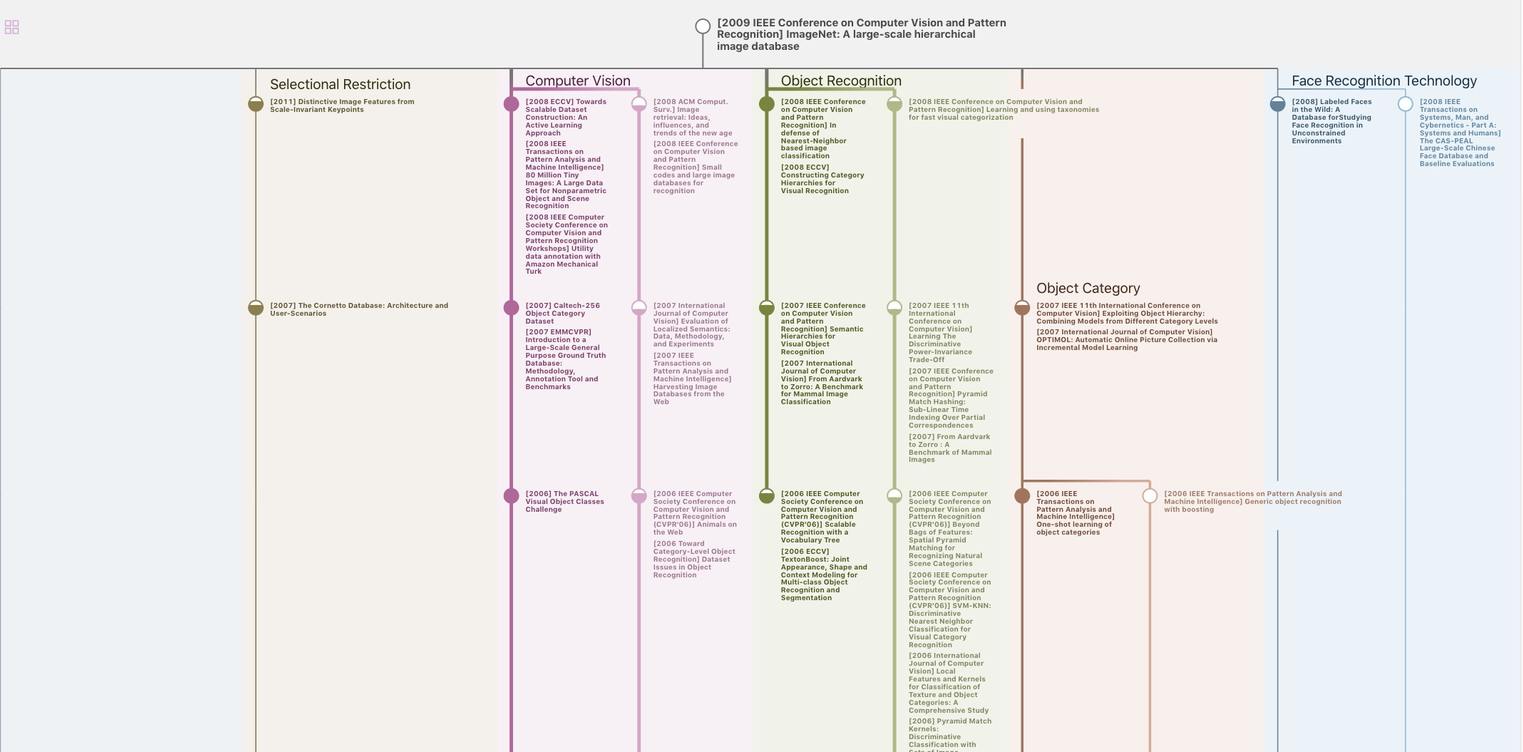
生成溯源树,研究论文发展脉络
Chat Paper
正在生成论文摘要