Scale-Separated Dynamic Mode Decomposition and Ionospheric Forecasting
arxiv(2023)
摘要
We present a method for forecasting the foF2 and hmF2 parameters using modal decompositions from measured ionospheric electron density profiles. Our method is based on Dynamic Mode Decomposition (DMD), which provides a means of determining spatiotemporal modes from measurements alone. Our proposed extensions to DMD use wavelet decompositions that provide separation of a wide range of high-intensity, transient temporal scales in the measured data. This scale separation allows for DMD models to be fit on each scale individually, and we show that together they generate a more accurate forecast of the time-evolution of the F-layer peak. We call this method the Scale-Separated Dynamic Mode Decomposition (SSDMD). The approach is shown to produce stable modes that can be used as a time-stepping model to predict the state of foF2 and hmF2 at a high time resolution. We demonstrate the SSDMD method on data sets covering periods of high and low solar activity as well as low, mid, and high latitude locations.
更多查看译文
关键词
modeling and forecasting,time series analysis,wavelet transform,nonlinear phenomena,signal processing
AI 理解论文
溯源树
样例
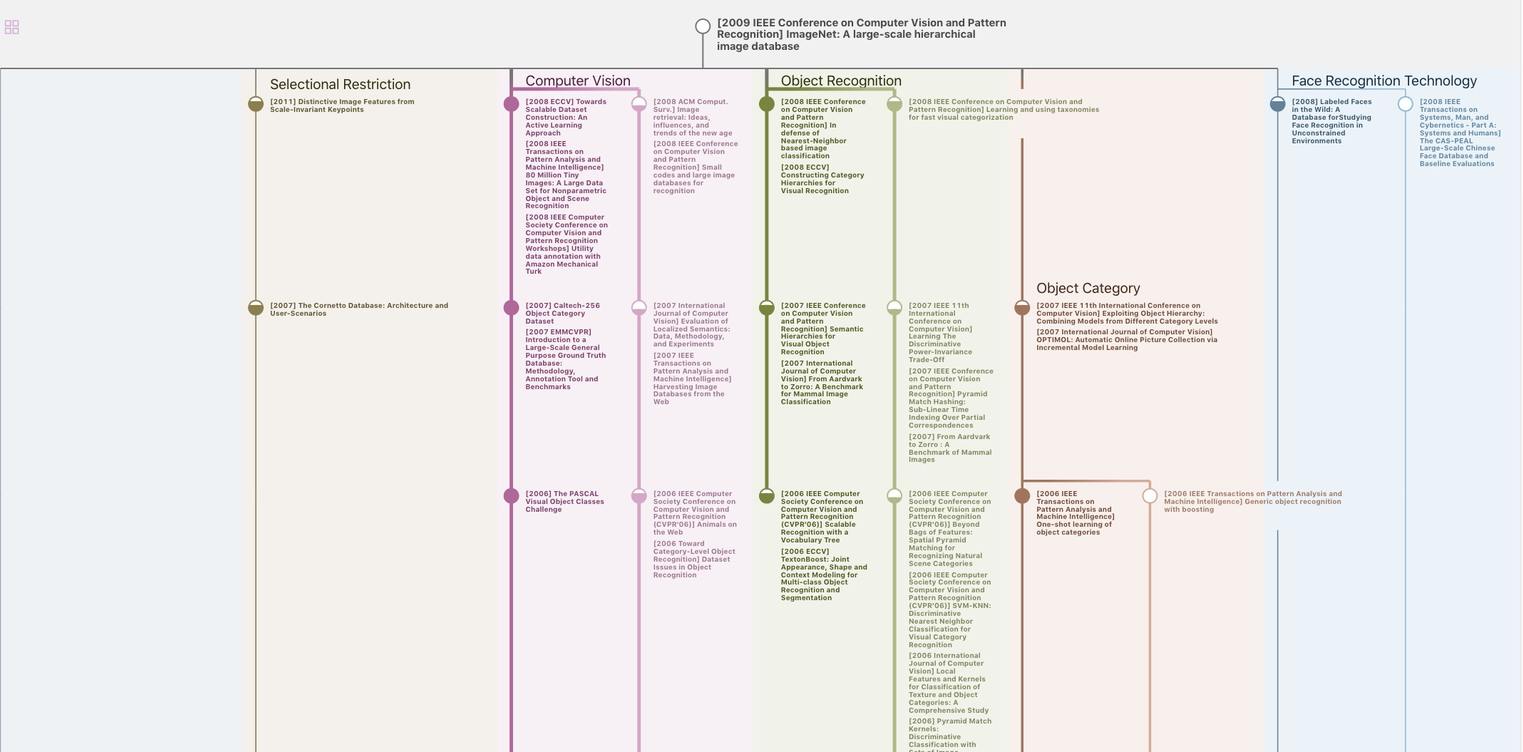
生成溯源树,研究论文发展脉络
Chat Paper
正在生成论文摘要