Semantic Structure Based Query Graph Prediction for Question Answering over Knowledge Graph.
ArXiv(2022)
摘要
Building query graphs from natural language questions is an important step in complex question answering over knowledge graph (Complex KGQA). In general, a question can be correctly answered if its query graph is built correctly and the right answer is then retrieved by issuing the query graph against the KG. Therefore, this paper focuses on query graph generation from natural language questions. Existing approaches for query graph generation ignore the semantic structure of a question, resulting in a large number of noisy query graph candidates that undermine prediction accuracies. In this paper, we define six semantic structures from common questions in KGQA and develop a novel Structure-BERT to predict the semantic structure of a question. By doing so, we can first filter out noisy candidate query graphs by the predicted semantic structures, and then rank the remaining candidates with a BERT-based ranking model. Extensive experiments on two popular benchmarks MetaQA and WebQuestionsSP (WSP) demonstrate the effectiveness of our method as compared to state-of-the-arts.
更多查看译文
关键词
query graph prediction,question answering
AI 理解论文
溯源树
样例
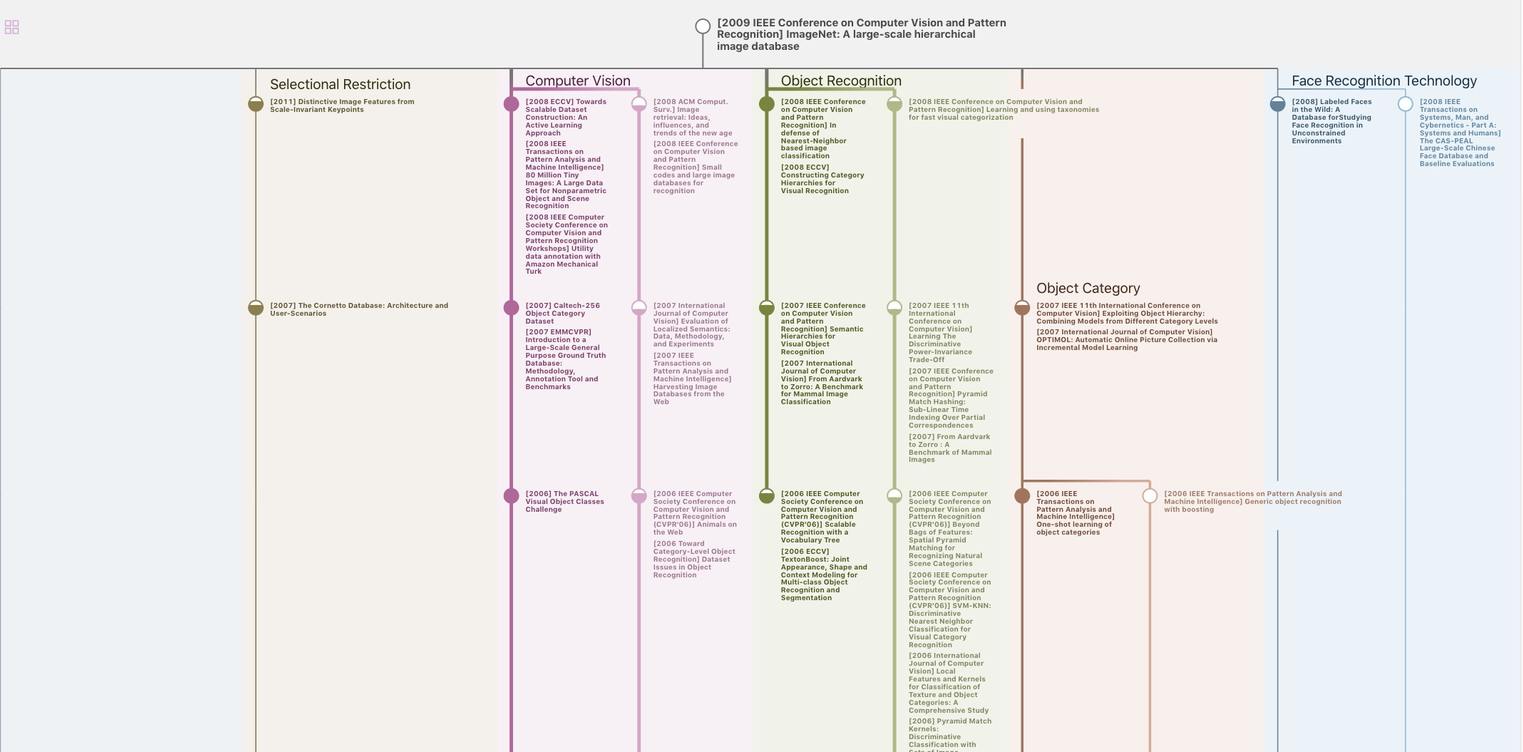
生成溯源树,研究论文发展脉络
Chat Paper
正在生成论文摘要