Scale Dependencies and Self-Similarity Through Wavelet Scattering Covariance
arxiv(2022)
摘要
We introduce a scattering covariance matrix which provides non-Gaussian models of time-series having stationary increments. A complex wavelet transform computes signal variations at each scale. Dependencies across scales are captured by the joint covariance across time and scales of complex wavelet coefficients and their modulus. This covariance is nearly diagonalized by a second wavelet transform, which defines the scattering covariance. We show that this set of moments characterizes a wide range of non-Gaussian properties of multi-scale processes. This is analyzed for a variety of processes, including fractional Brownian motions, Poisson, multifractal random walks and Hawkes processes. We prove that self-similar processes have a scattering covariance matrix which is scale invariant. This property can be estimated numerically and defines a class of wide-sense self-similar processes. We build maximum entropy models conditioned by scattering covariance coefficients, and generate new time-series with a microcanonical sampling algorithm. Applications are shown for highly non-Gaussian financial and turbulence time-series.
更多查看译文
关键词
wavelet,scattering,scale,self-similarity
AI 理解论文
溯源树
样例
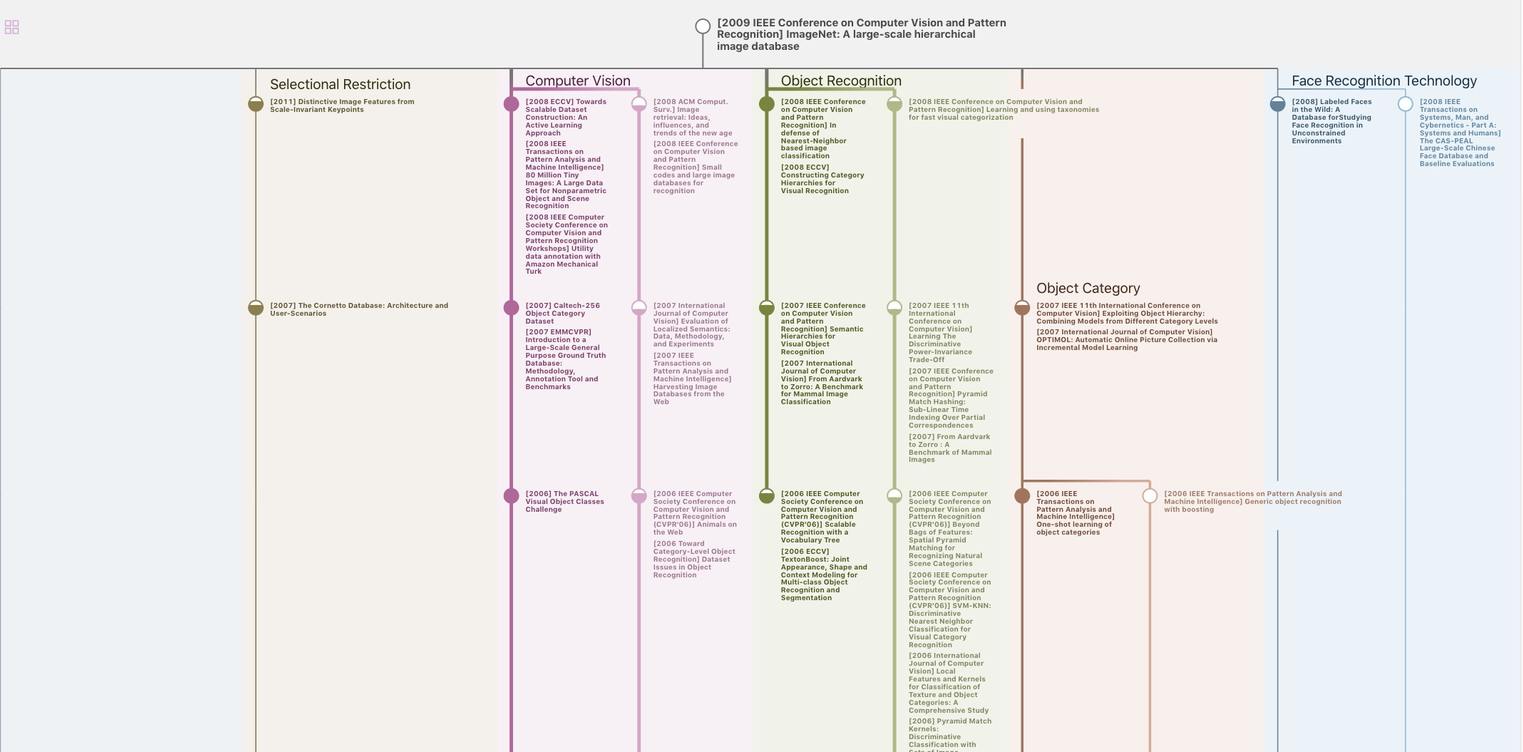
生成溯源树,研究论文发展脉络
Chat Paper
正在生成论文摘要