fairDMS: Rapid Model Training by Data and Model Reuse
2022 IEEE International Conference on Cluster Computing (CLUSTER)(2022)
摘要
Extracting actionable information rapidly from data produced by instruments such as the Linac Coherent Light Source (LCLS-II) and Advanced Photon Source Upgrade (APS-U) is becoming ever more challenging due to high (up to TB/s) data rates. Conventional physics-based information retrieval methods are hard-pressed to detect interesting events fast enough to enable timely focusing on a rare event or correction of an error. Machine learning (ML) methods that learn cheap surrogate classifiers present a promising alternative, but can fail catastrophically when changes in instrument or sample result in degradation in ML performance. To overcome such difficulties, we present a new data storage and ML model training architecture designed to organize large volumes of data and models so that when model degradation is detected, prior models and/or data can be queried rapidly and a more suitable model retrieved and fine-tuned for new conditions. We show that our approach can achieve up to 100x data labelling speedup compared to the current state-of-the-art, 200x improvement in training speed, and 92x speedup in-terms of end-to-end model updating time.
更多查看译文
关键词
rapid model training,model reuse,linac coherent light source,LCLS-II,advanced photon source upgrade,machine learning methods,ML performance,data storage,model degradation,end-to-end model updating time,physics-based information retrieval methods,surrogate classifiers
AI 理解论文
溯源树
样例
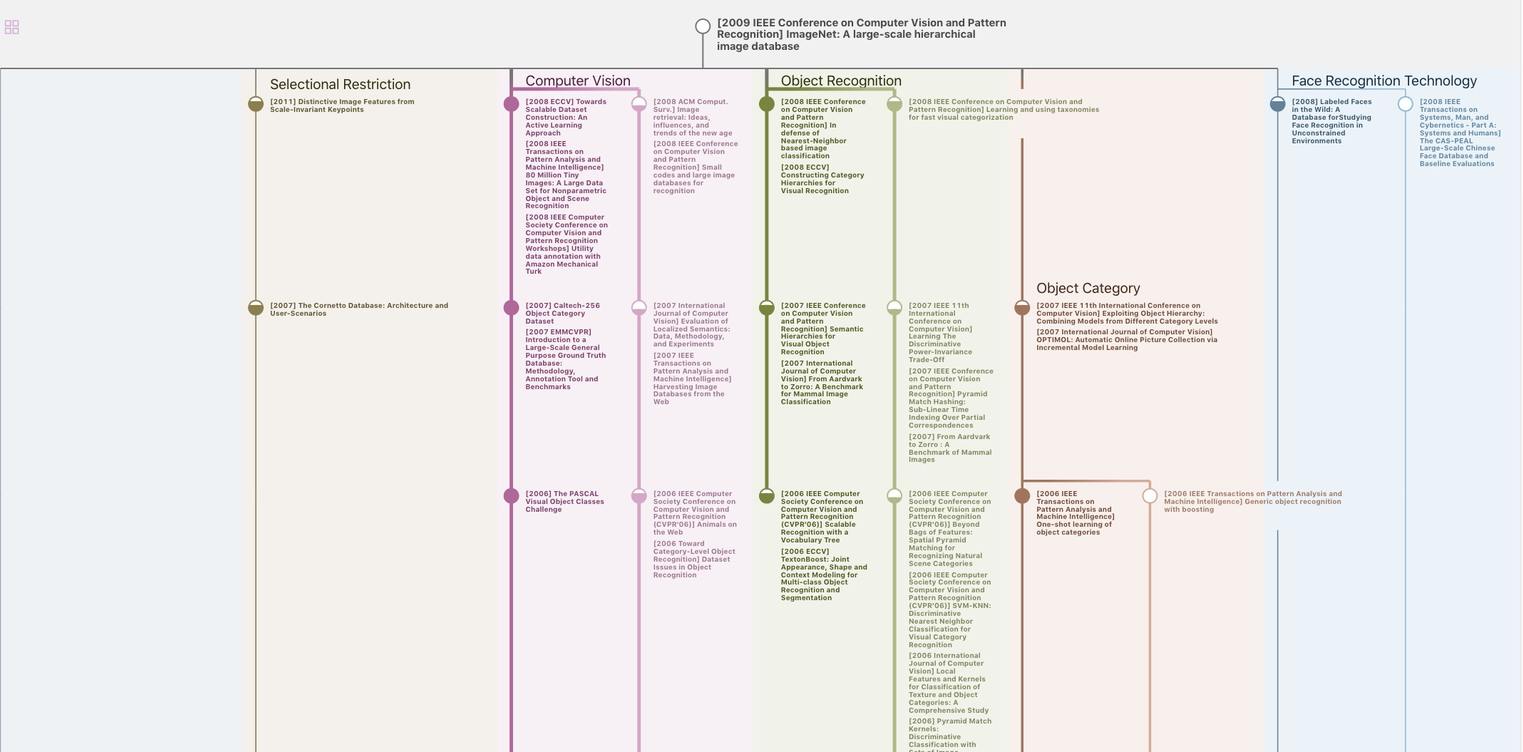
生成溯源树,研究论文发展脉络
Chat Paper
正在生成论文摘要