Development and validation of predictive models for COVID-19 outcomes in a safety-net hospital population
JOURNAL OF THE AMERICAN MEDICAL INFORMATICS ASSOCIATION(2022)
摘要
Objective To develop predictive models of coronavirus disease 2019 (COVID-19) outcomes, elucidate the influence of socioeconomic factors, and assess algorithmic racial fairness using a racially diverse patient population with high social needs. Materials and Methods Data included 7,102 patients with positive (RT-PCR) severe acute respiratory syndrome coronavirus 2 test at a safety-net system in Massachusetts. Linear and nonlinear classification methods were applied. A score based on a recurrent neural network and a transformer architecture was developed to capture the dynamic evolution of vital signs. Combined with patient characteristics, clinical variables, and hospital occupancy measures, this dynamic vital score was used to train predictive models. Results Hospitalizations can be predicted with an area under the receiver-operating characteristic curve (AUC) of 92% using symptoms, hospital occupancy, and patient characteristics, including social determinants of health. Parsimonious models to predict intensive care, mechanical ventilation, and mortality that used the most recent labs and vitals exhibited AUCs of 92.7%, 91.2%, and 94%, respectively. Early predictive models, using labs and vital signs closer to admission had AUCs of 81.1%, 84.9%, and 92%, respectively. Discussion The most accurate models exhibit racial bias, being more likely to falsely predict that Black patients will be hospitalized. Models that are only based on the dynamic vital score exhibited accuracies close to the best parsimonious models, although the latter also used laboratories. Conclusions This large study demonstrates that COVID-19 severity may accurately be predicted using a score that accounts for the dynamic evolution of vital signs. Further, race, social determinants of health, and hospital occupancy play an important role.
更多查看译文
关键词
COVID-19, AI, predictive modeling, racial bias, social determinants of health
AI 理解论文
溯源树
样例
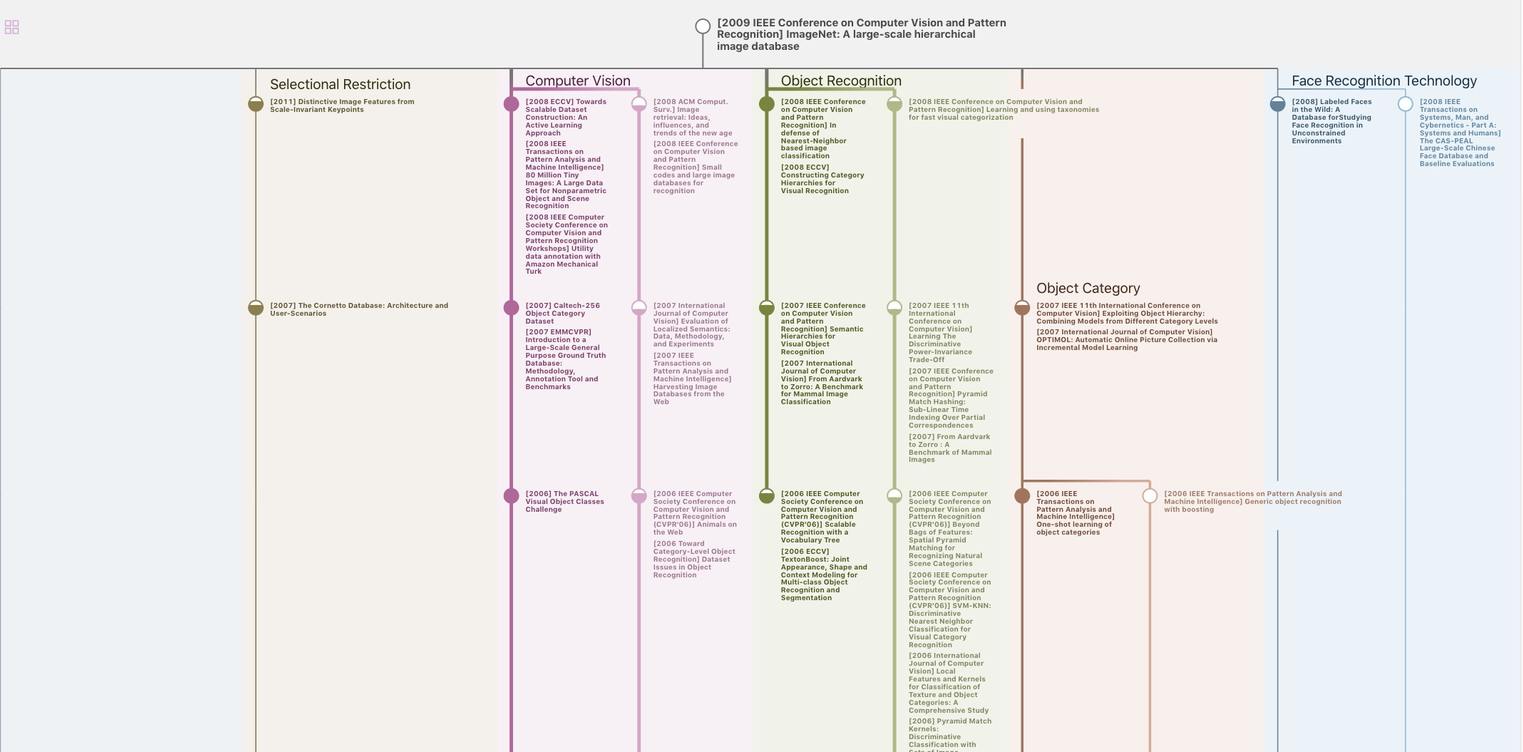
生成溯源树,研究论文发展脉络
Chat Paper
正在生成论文摘要