Physics-Informed Bayesian learning of electrohydrodynamic polymer jet printing dynamics
arxiv(2023)
摘要
Calibration of highly dynamic multi-physics manufacturing processes such as electrohydrodynamics-based additive manufacturing (AM) technologies (E-jet printing) is still performed by labor-intensive trial-and-error practices. Such practices have hindered the broad adoption of these technologies, demanding a new paradigm of self-calibrating E-jet printing machines. Here we develop an end-to-end physics-informed Bayesian learning framework (GPJet) which can learn the jet process dynamics with minimum experimental cost. GPJet consists of three modules: the machine vision module, the physics-based modeling module, and the machine learning (ML) module. GPJet was tested on a virtual E-jet printing machine with in-process jet monitoring capabilities. Our results show that the Machine Vision module can extract high-fidelity jet features in real-time from video data using an automated parallelized computer vision workflow. The Machine Vision module, combined with the Physics-based modeling module, can also act as closed-loop sensory feedback to the Machine Learning module of high- and low-fidelity data. This work extends the application of intelligent AM machines to more complex working conditions while reducing cost and increasing computational efficiency.
更多查看译文
关键词
electrohydrodynamic polymer jet
AI 理解论文
溯源树
样例
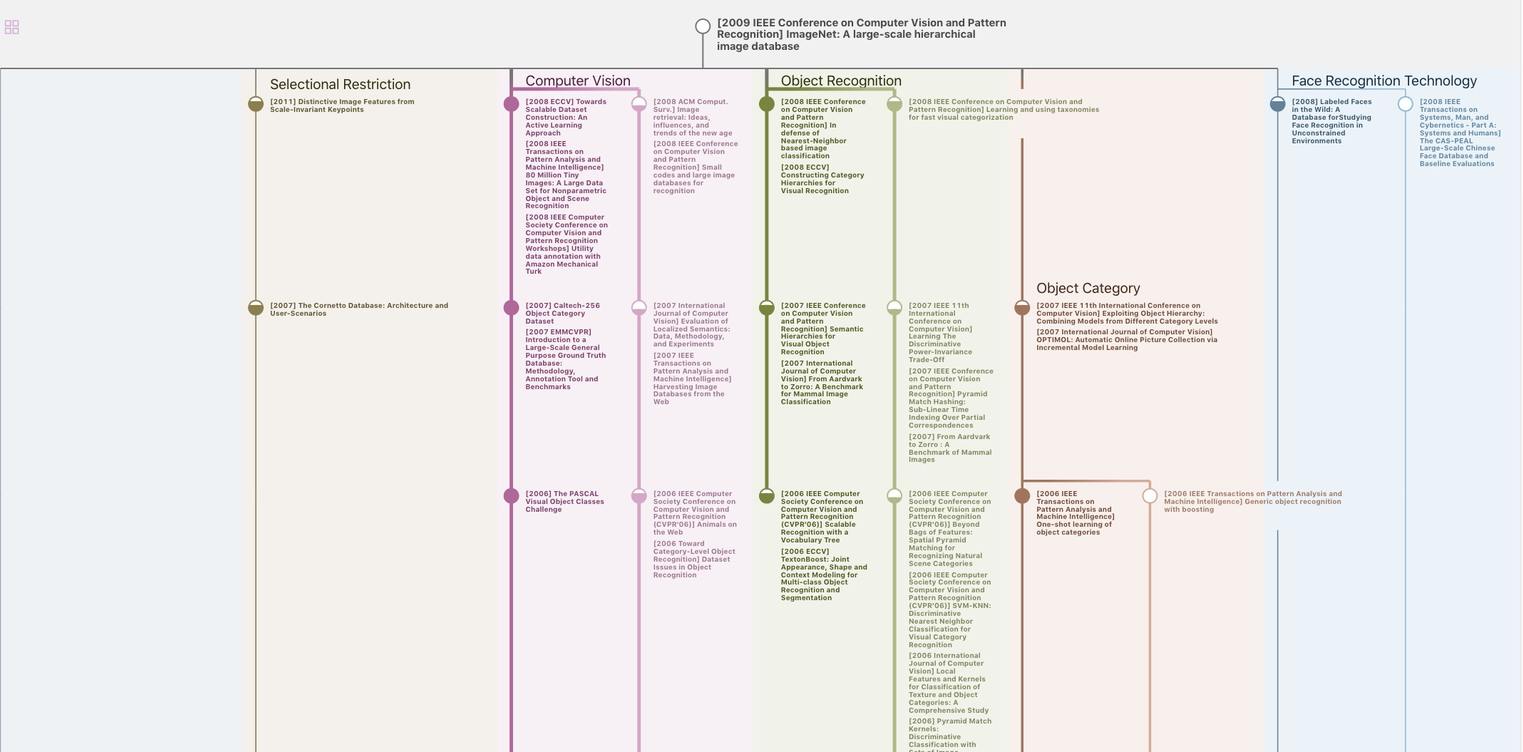
生成溯源树,研究论文发展脉络
Chat Paper
正在生成论文摘要