Active Few-Shot Learning with FASL
NATURAL LANGUAGE PROCESSING AND INFORMATION SYSTEMS (NLDB 2022)(2022)
摘要
Recent advances in natural language processing (NLP) have led to strong text classification models for many tasks. However, still often thousands of examples are needed to train models with good quality. This makes it challenging to quickly develop and deploy new models for real world problems and business needs. Few-shot learning and active learning are two lines of research, aimed at tackling this problem. In this work, we combine both lines into FASL, a platform that allows training text classification models using an iterative and fast process. We investigate which active learning methods work best in our few-shot setup. Additionally, we develop a model to predict when to stop annotating. This is relevant as in a few-shot setup we do not have access to a large validation set.
更多查看译文
关键词
Few-shot learning, Active learning, Siamese networks
AI 理解论文
溯源树
样例
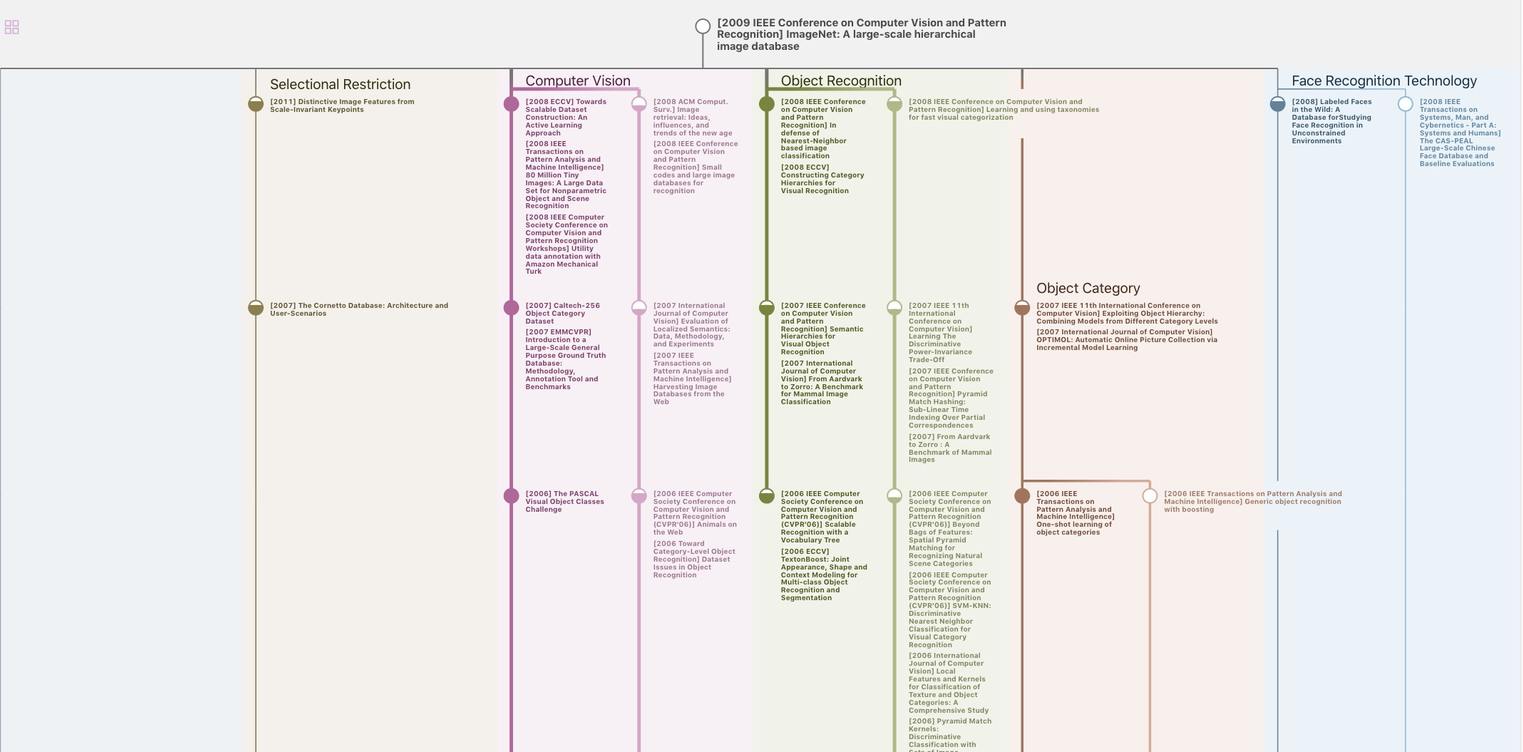
生成溯源树,研究论文发展脉络
Chat Paper
正在生成论文摘要