Learning Theory of Mind via Dynamic Traits Attribution.
International Joint Conference on Autonomous Agents and Multi-agent Systems(2022)
摘要
Machine learning of Theory of Mind (ToM) is essential to build social agents that co-live with humans and other agents. This capacity, once acquired, will help machines infer the mental states of others from observed contextual action trajectories, enabling future prediction of goals, intention, actions and successor representations. The underlying mechanism for such a prediction remains unclear, however. Inspired by the observation that humans often infer the character traits of others, then use it to explain behaviour, we propose a new neural ToM architecture that learns to generate a latent trait vector of an actor from the past trajectories. This trait vector then multiplicatively modulates the prediction mechanism via a `fast weights' scheme in the prediction neural network, which reads the current context and predicts the behaviour. We empirically show that the fast weights provide a good inductive bias to model the character traits of agents and hence improves mindreading ability. On the indirect assessment of false-belief understanding, the new ToM model enables more efficient helping behaviours.
更多查看译文
关键词
attribution,learning,mind,theory
AI 理解论文
溯源树
样例
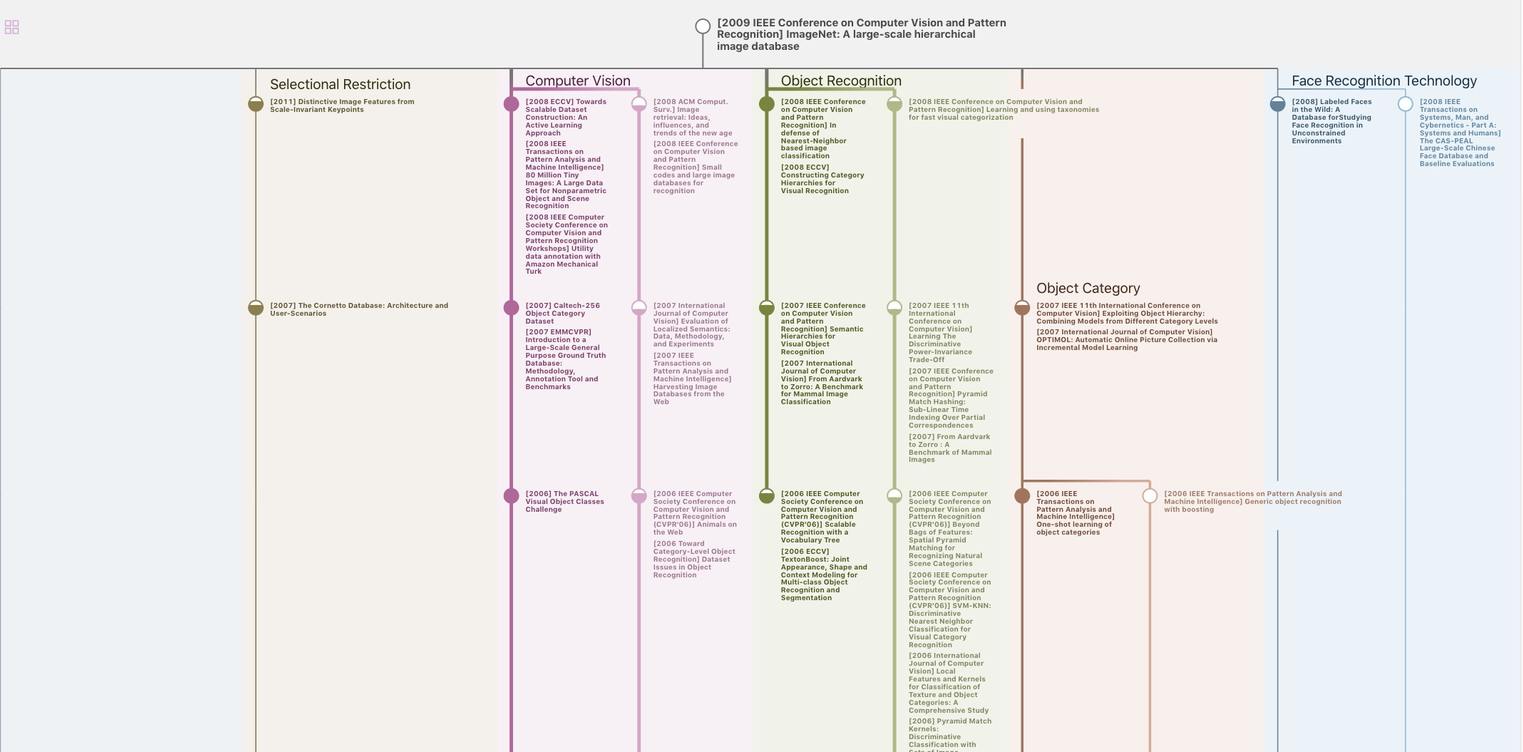
生成溯源树,研究论文发展脉络
Chat Paper
正在生成论文摘要