Motion-compensated online object tracking for activity detection and crowd behavior analysis
The Visual computer(2022)
摘要
It is a nontrivial task to manage crowds in public places and recognize unacceptable behavior (such as violating social distancing norms during the COVID-19 pandemic). In such situations, people should avoid loitering (unnecessary moving out in public places without apparent purpose) and maintain a sufficient physical distance. In this study, a multi-object tracking algorithm has been introduced to improve short-term object occlusion, detection errors, and identity switches. The objects are tracked through bounding box detection and with linear velocity estimation of the object using the Kalman filter frame by frame. The predicted tracks are kept alive for some time, handling the missing detections and short-term object occlusion. ID switches (mainly due to crossing trajectories) are managed by explicitly considering the motion direction of the objects in real time. Furthermore, a novel approach to detect unusual behavior of loitering with a severity level is proposed based on the tracking information. An adaptive algorithm is also proposed to detect physical distance violation based on the object dimensions for the entire length of the track. At last, a mathematical approach to calculate actual physical distance is proposed by using the height of a human as a reference object which adheres more specific distancing norms. The proposed approach is evaluated in traffic and pedestrian movement scenarios. The experimental results demonstrate a significant improvement in the results.
更多查看译文
关键词
Activity recognition,Loitering,Object tracking,Pedestrian movement,Physical distancing
AI 理解论文
溯源树
样例
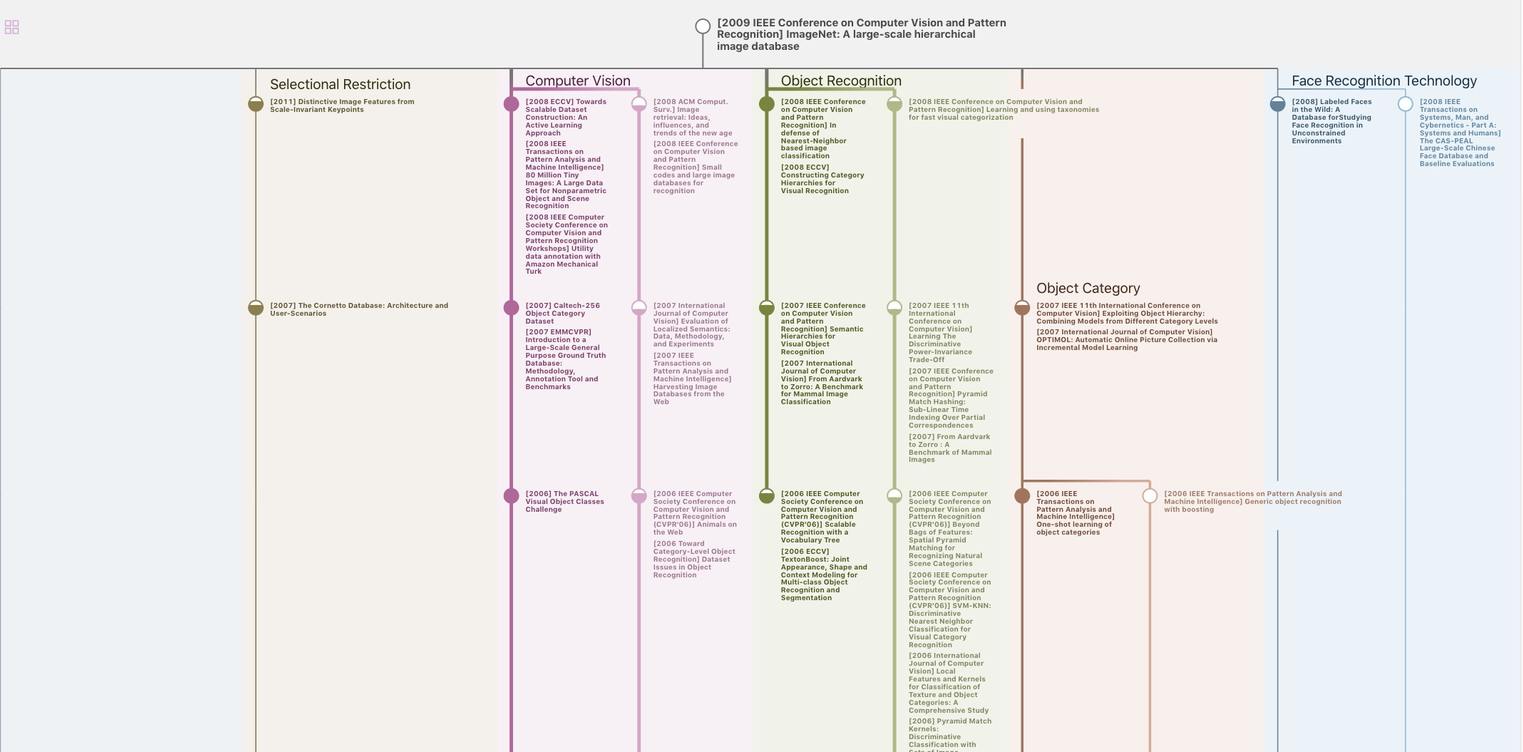
生成溯源树,研究论文发展脉络
Chat Paper
正在生成论文摘要