An Investigation of Monotonic Transducers for Large-Scale Automatic Speech Recognition
2022 IEEE Spoken Language Technology Workshop (SLT)(2023)
摘要
The two most popular loss functions for streaming end-to-end automatic speech recognition (ASR) are RNN-Transducer (RNN-T) and connectionist temporal classification (CTC). Between these two loss types we can classify the monotonic RNN-T (MonoRNN-T) and the recently proposed CTC-like Transducer (CTC-T). Monotonic transducers have a few advantages. First, RNN-T can suffer from runaway hallucination, where a model keeps emitting non-blank symbols without advancing in time. Secondly, monotonic transducers consume exactly one model score per time step and are therefore more compatible with traditional FST-based ASR decoders. However, the MonoRNN-T so far has been found to have worse accuracy than RNN-T. It does not have to be that way: By regularizing the training via joint LAS training or parameter initialization from RNN-T, both MonoRNN-T and CTC-T perform as well or better than RNN-T. This is demonstrated for LibriSpeech and for a large-scale in-house data set.
更多查看译文
关键词
speech recognition,monotonic transducer,GTC-T,RNN-T
AI 理解论文
溯源树
样例
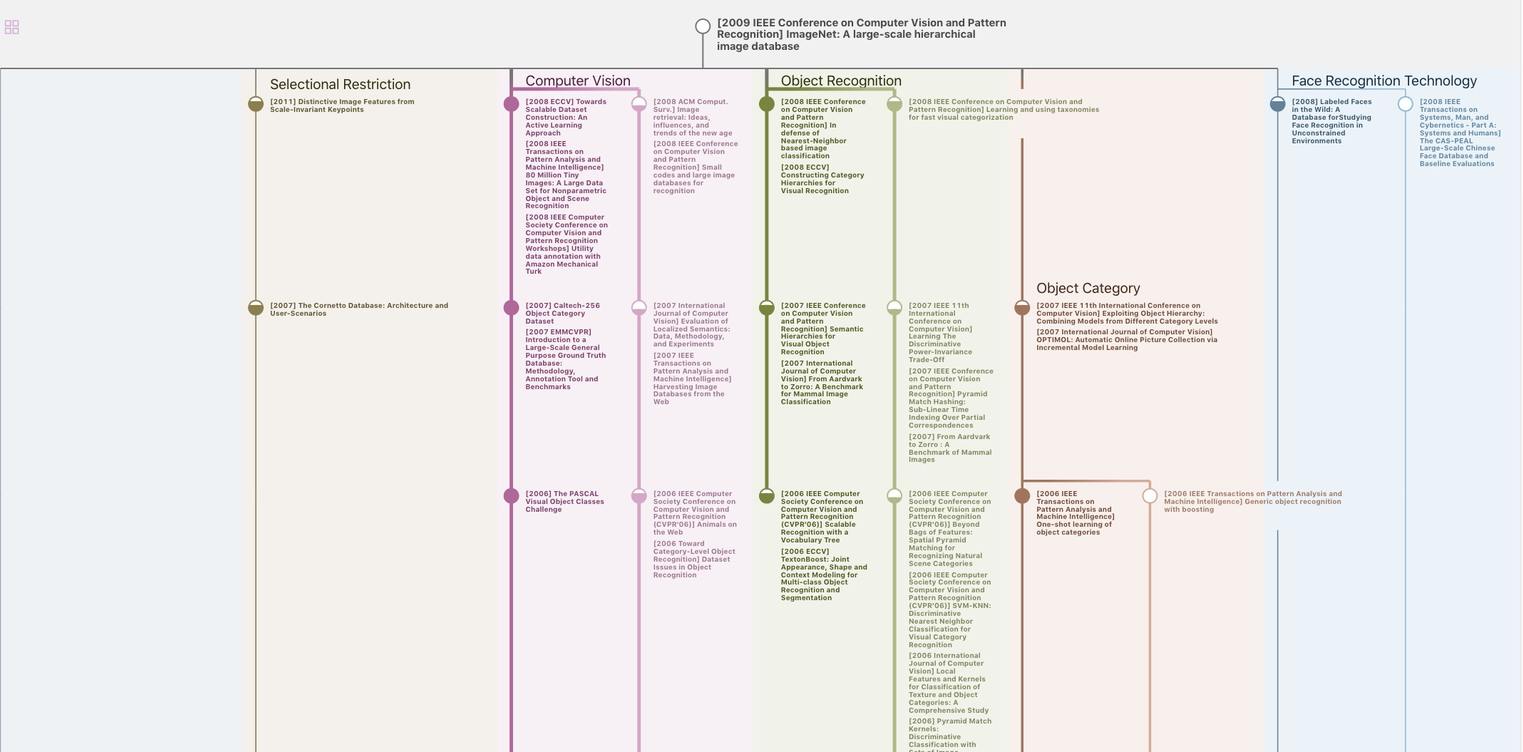
生成溯源树,研究论文发展脉络
Chat Paper
正在生成论文摘要