Independence Testing for Bounded Degree Bayesian Network
NeurIPS 2022(2022)
摘要
We study the following independence testing problem: given access to samples from a distribution $P$ over $\{0,1\}^n$, decide whether $P$ is a product distribution or whether it is $\varepsilon$-far in total variation distance from any product distribution. For arbitrary distributions, this problem requires $\exp(n)$ samples. We show in this work that if $P$ has a sparse structure, then in fact only linearly many samples are required. Specifically, if $P$ is Markov with respect to a Bayesian network whose underlying DAG has in-degree bounded by $d$, then $\tilde{\Theta}(2^{d/2}\cdot n/\varepsilon^2)$ samples are necessary and sufficient for independence testing.
更多查看译文
关键词
Distribution testing,Bayesian Network,Probabilistic Graphical Model
AI 理解论文
溯源树
样例
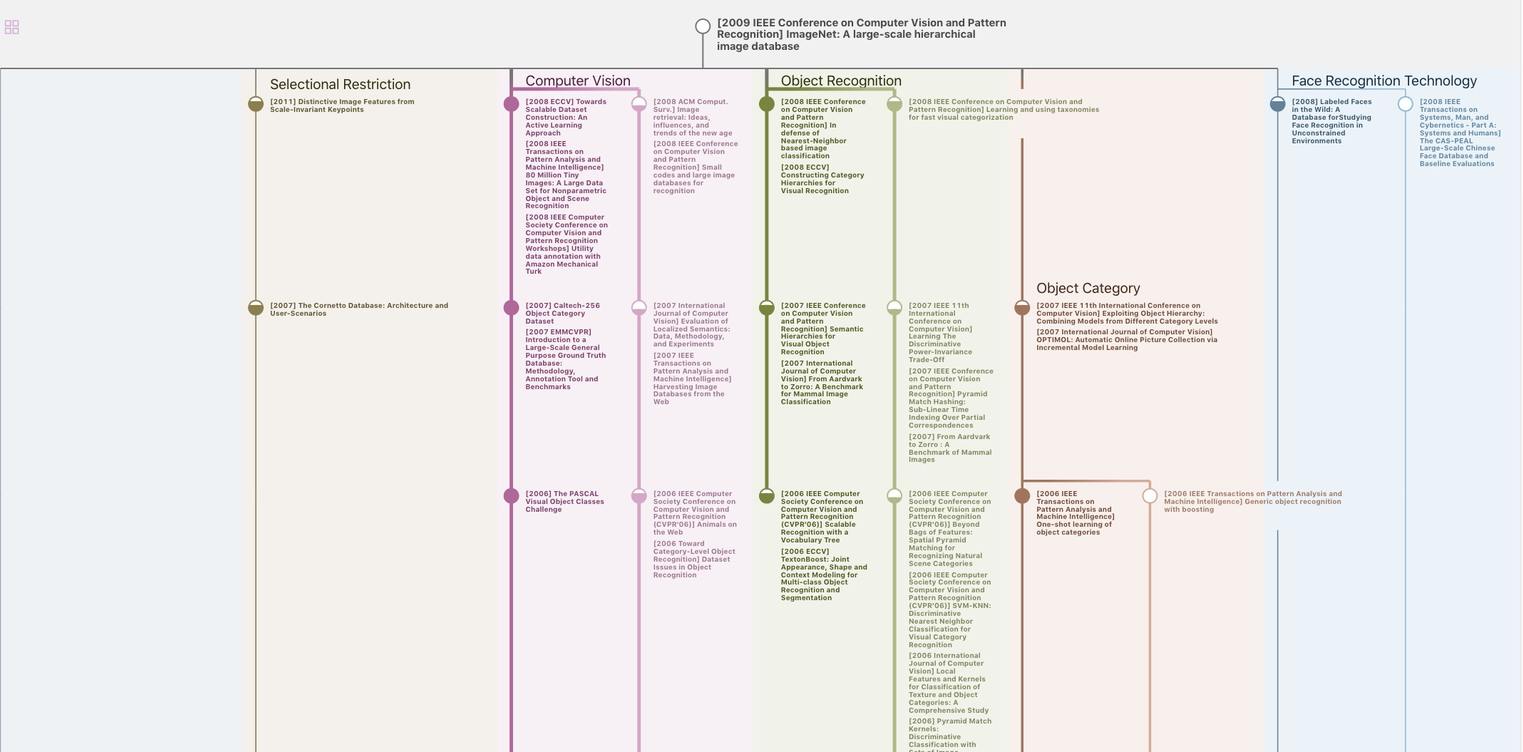
生成溯源树,研究论文发展脉络
Chat Paper
正在生成论文摘要