Role of intra-tumoral vasculature imaging features on susceptibility weighted imaging in differentiating primary central nervous system lymphoma from glioblastoma: a multiparametric comparison with pathological validation
Neuroradiology(2022)
摘要
Purpose Primary objective of this study was to retrospectively evaluate the potential of a range of qualitative and quantitative multiparametric features assessed on T 2 , post-contrast T 1 , DWI, DCE-MRI, and susceptibility-weighted-imaging (SWI) in differentiating evenly sampled cohort of primary-central-nervous-system-lymphoma (PCNSL) vs glioblastoma (GB) with pathological validation. Methods The study included MRI-data of histopathologically confirmed ninety-five GB and PCNSL patients scanned at 3.0 T MRI. A total of six qualitative features (three from T 2 and post-contrast T 1 , three from SWI: thin-linear-uninterrupted-intra-tumoral-vasculature, broken-intra-tumoral-microvasculature, hemorrhage) were analyzed by three independent radiologists. Ten quantitative features from DWI and DCE-MRI were computed using in-house-developed algorithms. For qualitative features, Cohen’s Kappa-interrater-variability-analysis was performed. Z -test and independent t -tests were performed to find significant qualitative and quantitative features respectively. Logistic-regression (LR) classifiers were implemented for evaluating performance of individual and various combinations of features in differentiating PCNSL vs GB. Performance evaluation was done via ROC-analysis. Pathological validation was performed to verify disintegration of vessel walls in GB and rim of viable neoplastic lymphoid cells with angiocentric-pattern in PCNSL. Results Three qualitative SWI features and four quantitative DCE-MRI features (rCBVcorr, K ep , V e , and necrosis-volume-percentage) were significantly different ( p < 0.05) between PCNSL and GB. Best diagnostic performance was observed with LR classifier using SWI features (AUC-0.99). The inclusion of quantitative features with SWI feature did not improve the differentiation accuracy. Conclusions The combination of three qualitative SWI features using LR provided the highest accuracy in differentiating PCNSL and GB. Thin-linear-uninterrupted-intra-tumoral-vasculature in PCNSL and broken-intra-tumoral-microvasculature with hemorrhage in GB are the major contributors to the differentiation.
更多查看译文
关键词
Brain MRI, PCNSL, SWI, DCE-MRI, GB
AI 理解论文
溯源树
样例
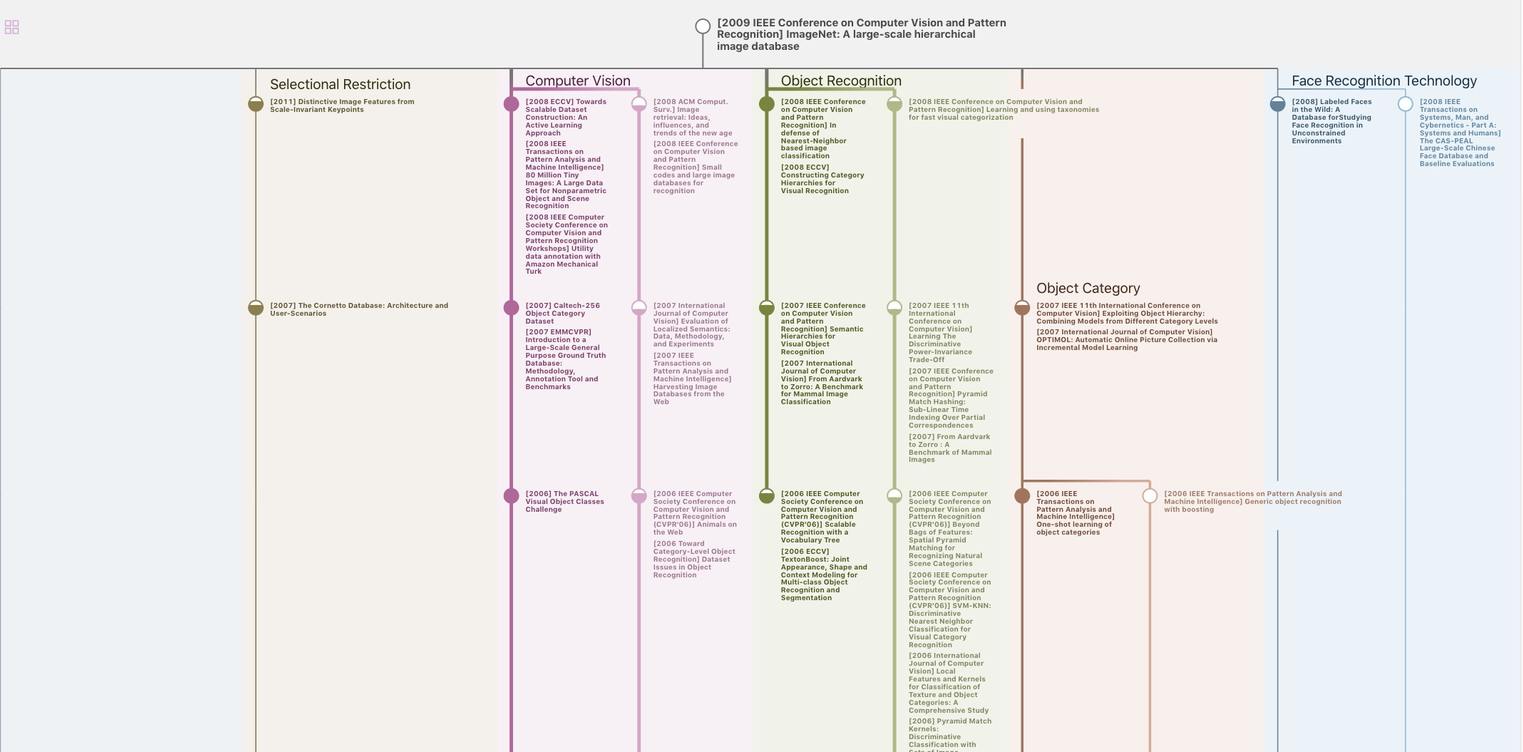
生成溯源树,研究论文发展脉络
Chat Paper
正在生成论文摘要