Prediction, Knowledge, and Explainability: Examining the Use of General Value Functions in Machine Knowledge
Frontiers in Artificial Intelligence(2022)
摘要
Within computational reinforcement learning, a growing body of work seeks to express an agent's knowledge of its world through large collections of predictions. While systems that encode predictions as General Value Functions (GVFs) have seen numerous developments in both theory and application, whether such approaches are explainable is unexplored. In this perspective piece, we explore GVFs as a form of explainable AI. To do so, we articulate a subjective agent-centric approach to explainability in sequential decision-making tasks. We propose that prior to explaining its decisions to others, an self-supervised agent must be able to introspectively explain decisions to itself. To clarify this point, we review prior applications of GVFs that involve human-agent collaboration. In doing so, we demonstrate that by making their subjective explanations public, predictive knowledge agents can improve the clarity of their operation in collaborative tasks.
更多查看译文
关键词
General Value Functions,explainability,knowledge,prediction,reinforcement learning
AI 理解论文
溯源树
样例
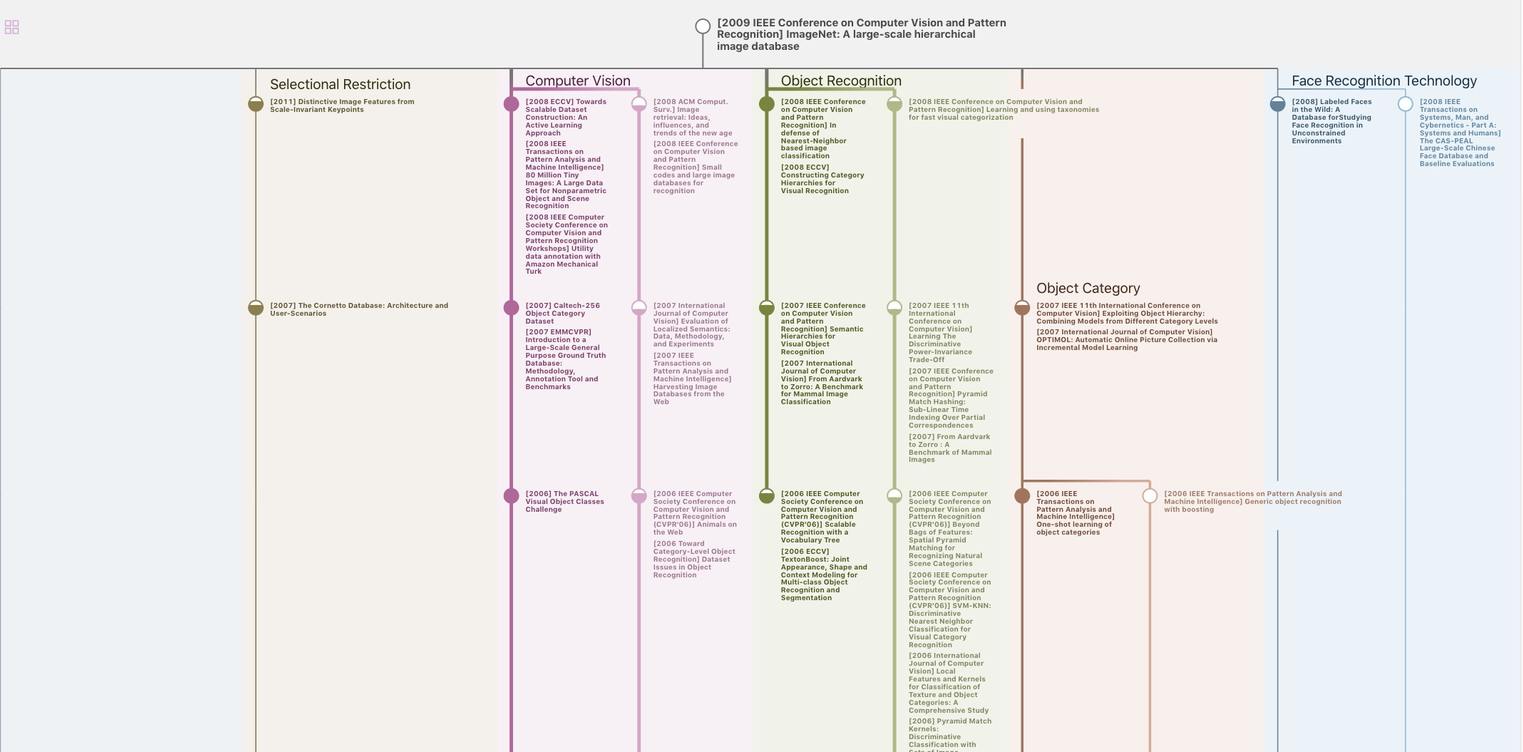
生成溯源树,研究论文发展脉络
Chat Paper
正在生成论文摘要