MP2: A Momentum Contrast Approach for Recommendation with Pointwise and Pairwise Learning
SIGIR '22: Proceedings of the 45th International ACM SIGIR Conference on Research and Development in Information Retrieval(2022)
摘要
Binary pointwise labels (aka implicit feedback) are heavily leveraged by deep learning based recommendation algorithms nowadays. In this paper we discuss the limited expressiveness of these labels may fail to accommodate varying degrees of user preference, and thus lead to conflicts during model training, which we call annotation bias. To solve this issue, we find the soft-labeling property of pairwise labels could be utilized to alleviate the bias of pointwise labels. To this end, we propose a momentum contrast framework (\method ) that combines pointwise and pairwise learning for recommendation. \method has a three-tower network structure: one user network and two item networks. The two item networks are used for computing pointwise and pairwise loss respectively. To alleviate the influence of the annotation bias, we perform a momentum update to ensure a consistent item representation. Extensive experiments on real-world datasets demonstrate the superiority of our method against state-of-the-art recommendation algorithms.
更多查看译文
关键词
Recommendation, Momentum update, Pointwise learning, Pairwise learning
AI 理解论文
溯源树
样例
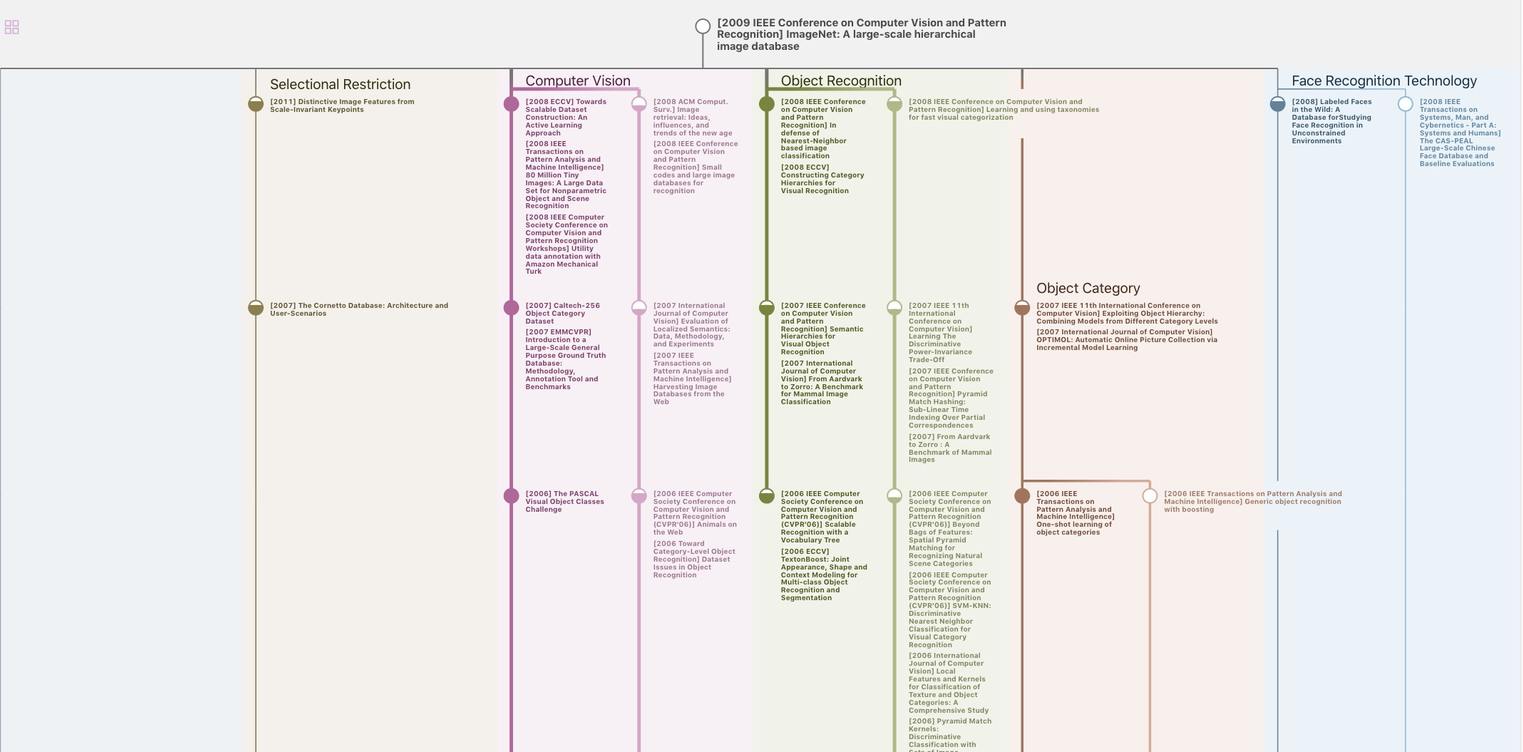
生成溯源树,研究论文发展脉络
Chat Paper
正在生成论文摘要