Super Resolution for Turbulent Flows in 2D: Stabilized Physics Informed Neural Networks
arxiv(2022)
摘要
We propose a new design of a neural network for solving a zero shot super resolution problem for turbulent flows. We embed Luenberger-type observer into the network's architecture to inform the network of the physics of the process, and to provide error correction and stabilization mechanisms. In addition, to compensate for decrease of observer's performance due to the presence of unknown destabilizing forcing, the network is designed to estimate the contribution of the unknown forcing implicitly from the data over the course of training. By running a set of numerical experiments, we demonstrate that the proposed network does recover unknown forcing from data and is capable of predicting turbulent flows in high resolution from low resolution noisy observations.
更多查看译文
关键词
error correction,low resolution noisy observations,Luenberger-type observer,neural network,physics informed neural networks,stabilization mechanisms,turbulent flows,unknown destabilizing forcing,zero shot super resolution problem
AI 理解论文
溯源树
样例
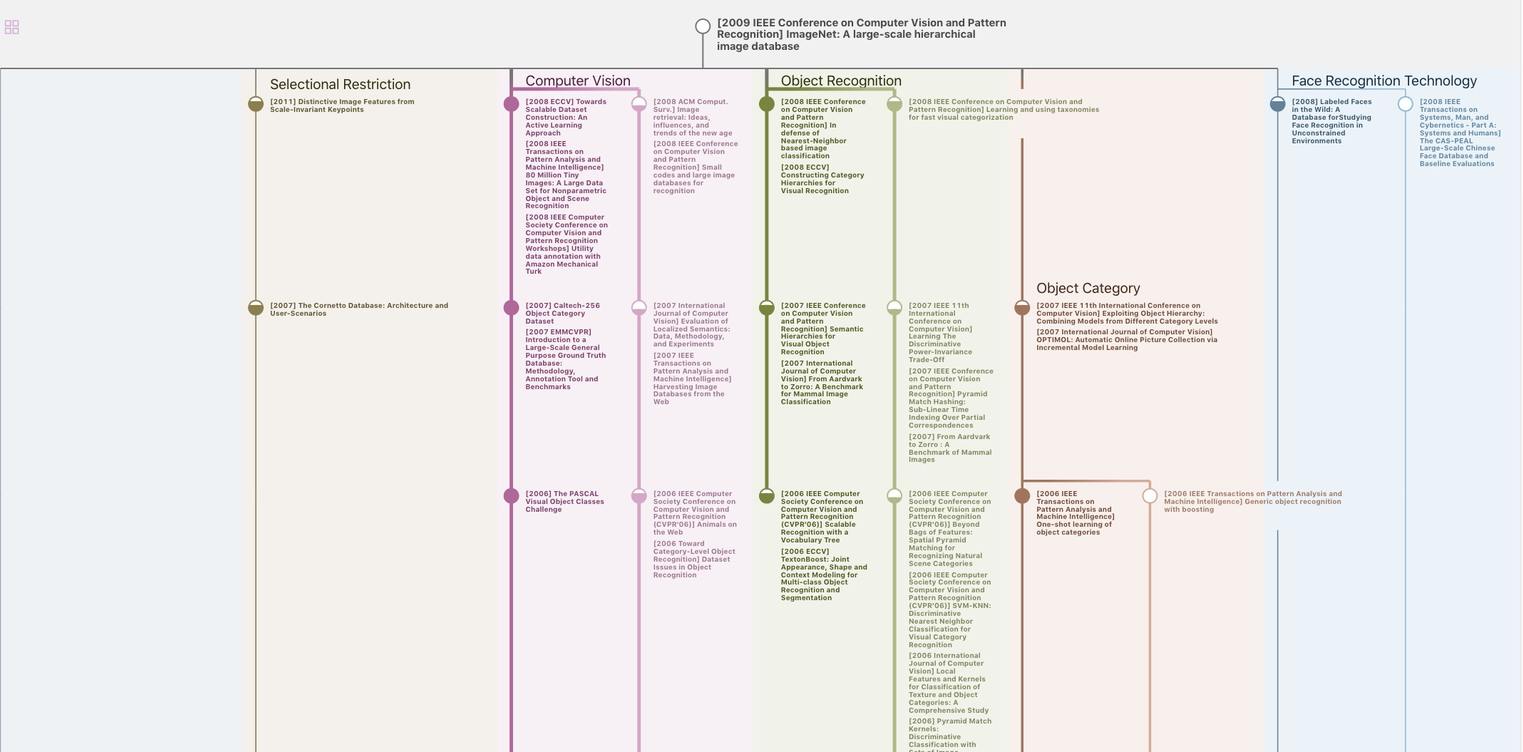
生成溯源树,研究论文发展脉络
Chat Paper
正在生成论文摘要