Ridge-Regression-Induced Robust Graph Relational Network
IEEE transactions on cybernetics(2023)
摘要
Graph convolutional networks (GCNs) have attracted increasing research attention, which merits in its strong ability to handle graph data, such as the citation network or social network. Existing models typically use first-order neighborhood information to design specific convolution operations, which aggregate the features of all adjacent nodes. However, such models ignore the high-order spatial relationship among neighboring nodes in noisy data due to its modeling complexity. In this article, we propose a novel robust graph relational network to address this issue toward modeling high-order relationships in noisy data for graph convolution. Our key innovation lies in designing a generic relation network layer, which is used to infer the underlying relations among adjacent noisy nodes. Specifically, a fixed number of adjacent nodes for each node is chosen by solving the ridge regression problem, in which the regression coefficients are used to rank the adjacent nodes of each node in a graph. Furthermore, to mine the rich features, we extract high-order information from the nodes to significantly enhance the representation ability of the GCNs for extensive applications. We conduct extensive semisupervised node classification experiments on the noisy benchmark datasets, which clearly show that our model is superior to the existing methods and can achieve state-of-the-art performance.
更多查看译文
关键词
Noise measurement,Computational modeling,Cognition,Neural networks,Data models,Convolution,Aggregates,Graph,relational network (RN),ridge regression,robustness
AI 理解论文
溯源树
样例
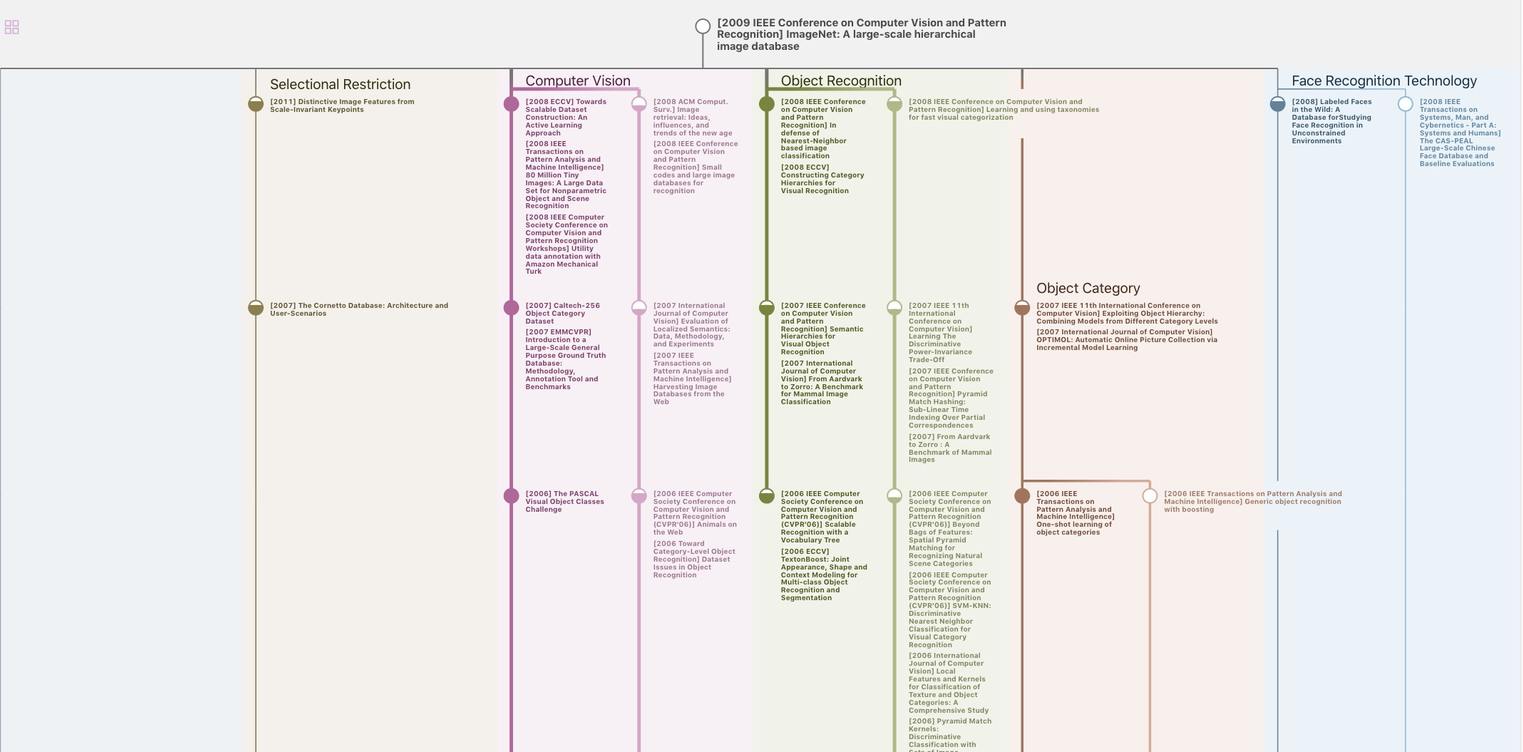
生成溯源树,研究论文发展脉络
Chat Paper
正在生成论文摘要