Automated Registration-Based Temporal Bone Computed Tomography Segmentation for Applications in Neurotologic Surgery
OTOLARYNGOLOGY-HEAD AND NECK SURGERY(2022)
摘要
Objective This study investigates the accuracy of an automated method to rapidly segment relevant temporal bone anatomy from cone beam computed tomography (CT) images. Implementation of this segmentation pipeline has potential to improve surgical safety and decrease operative time by augmenting preoperative planning and interfacing with image-guided robotic surgical systems. Study Design Descriptive study of predicted segmentations. Setting Academic institution. Methods We have developed a computational pipeline based on the symmetric normalization registration method that predicts segmentations of anatomic structures in temporal bone CT scans using a labeled atlas. To evaluate accuracy, we created a data set by manually labeling relevant anatomic structures (eg, ossicles, labyrinth, facial nerve, external auditory canal, dura) for 16 deidentified high-resolution cone beam temporal bone CT images. Automated segmentations from this pipeline were compared against ground-truth manual segmentations by using modified Hausdorff distances and Dice scores. Runtimes were documented to determine the computational requirements of this method. Results Modified Hausdorff distances and Dice scores between predicted and ground-truth labels were as follows: malleus (0.100 +/- 0.054 mm; Dice, 0.827 +/- 0.068), incus (0.100 +/- 0.033 mm; Dice, 0.837 +/- 0.068), stapes (0.157 +/- 0.048 mm; Dice, 0.358 +/- 0.100), labyrinth (0.169 +/- 0.100 mm; Dice, 0.838 +/- 0.060), and facial nerve (0.522 +/- 0.278 mm; Dice, 0.567 +/- 0.130). A quad-core 16GB RAM workstation completed this segmentation pipeline in 10 minutes. Conclusions We demonstrated submillimeter accuracy for automated segmentation of temporal bone anatomy when compared against hand-segmented ground truth using our template registration pipeline. This method is not dependent on the training data volume that plagues many complex deep learning models. Favorable runtime and low computational requirements underscore this method's translational potential.
更多查看译文
关键词
temporal bone, automated segmentation, atlas, data set curation
AI 理解论文
溯源树
样例
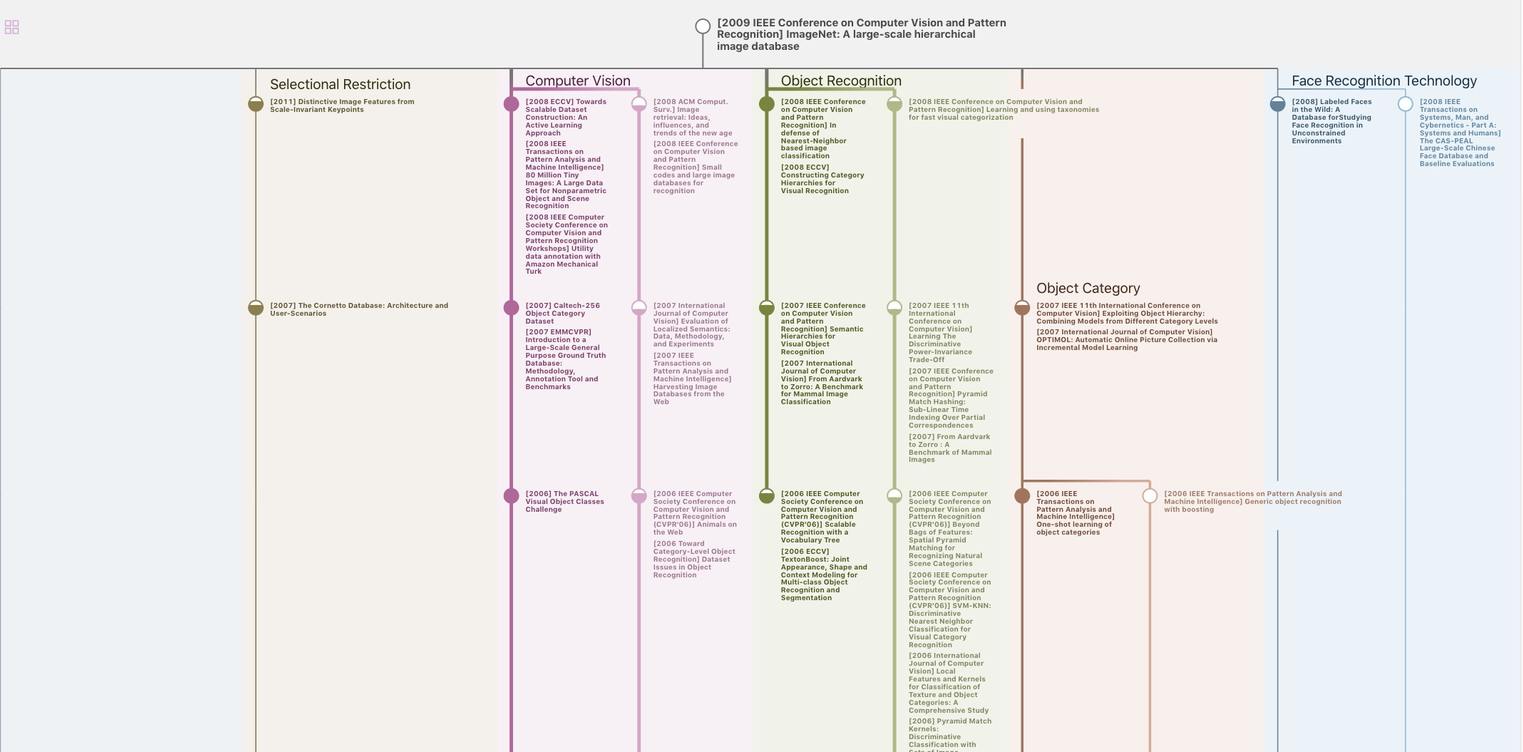
生成溯源树,研究论文发展脉络
Chat Paper
正在生成论文摘要