A Machine Learning Approach to Automatic Classification of Eight Sleep Disorders
arXiv (Cornell University)(2022)
摘要
In this research, we attempt to answer the following basic research questions: Is a machine learning model able to classify all types of sleep disorders with high accuracy? Among the different modalities of sleep disorder signals, are some more important than others? Do raw signals improve the performance of a deep learning model when they are used as inputs? Prior research showed that most sleep disorders belong to eight categories. To study the performance of machine learning models in classifying polysomnography recordings into the eight categories of sleep pathologies, we selected the Cyclic Alternating Pattern Sleep Database. We developed a multi-channel Deep Learning model where a set of Convolutional Neural Networks were applied to six channels of raw signals of different modalities, including three channels of EEG signals and one channel each of EMG, ECG , and EOG signals. To compare the performance of the DL model with other models, we designed a model that took spectral features, instead of raw signals, as its inputs. We first studied the "importance" issue of signal modalities using the RF algorithm. We found that ECG contributed most to the important features and EMG second, among the four signal modalities. We then studied the accuracy performance of the proposed machine learning models. We verified that the multi-channel DL-R model, which took raw signals as its inputs, outperformed all other models, with its sensitivity and specificity scores both being above 95 %. This accuracy performance is on a par with those published results which dealt with fewer types of sleep disorders. We adopted two popular heatmap-generating techniques, with which we confirmed that the DL model's superior performance was owing to the CNN network's ability to extract potent features from raw signals.
更多查看译文
关键词
eight sleep
AI 理解论文
溯源树
样例
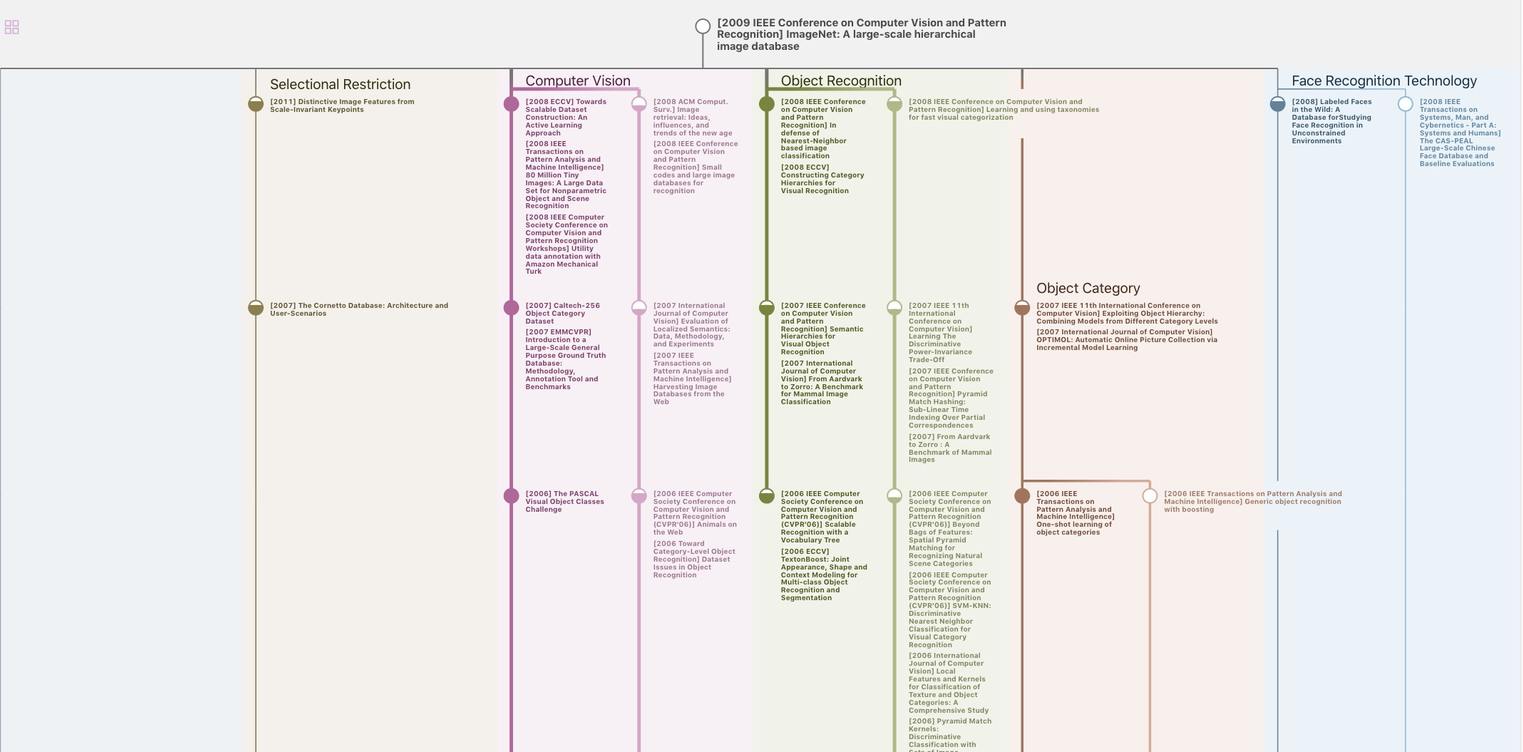
生成溯源树,研究论文发展脉络
Chat Paper
正在生成论文摘要