ULF: Unsupervised Labeling Function Correction using Cross-Validation for Weak Supervision
arxiv(2022)
摘要
A cost-effective alternative to manual data labeling is weak supervision
(WS), where data samples are automatically annotated using a predefined set of
labeling functions (LFs), rule-based mechanisms that generate artificial labels
for the associated classes. In this work, we investigate noise reduction
techniques for WS based on the principle of k-fold cross-validation. We
introduce a new algorithm ULF for Unsupervised Labeling Function correction,
which denoises WS data by leveraging models trained on all but some LFs to
identify and correct biases specific to the held-out LFs. Specifically, ULF
refines the allocation of LFs to classes by re-estimating this assignment on
highly reliable cross-validated samples. Evaluation on multiple datasets
confirms ULF's effectiveness in enhancing WS learning without the need for
manual labeling.
更多查看译文
关键词
nlp,weak supervision,text classification,sentiment analysis
AI 理解论文
溯源树
样例
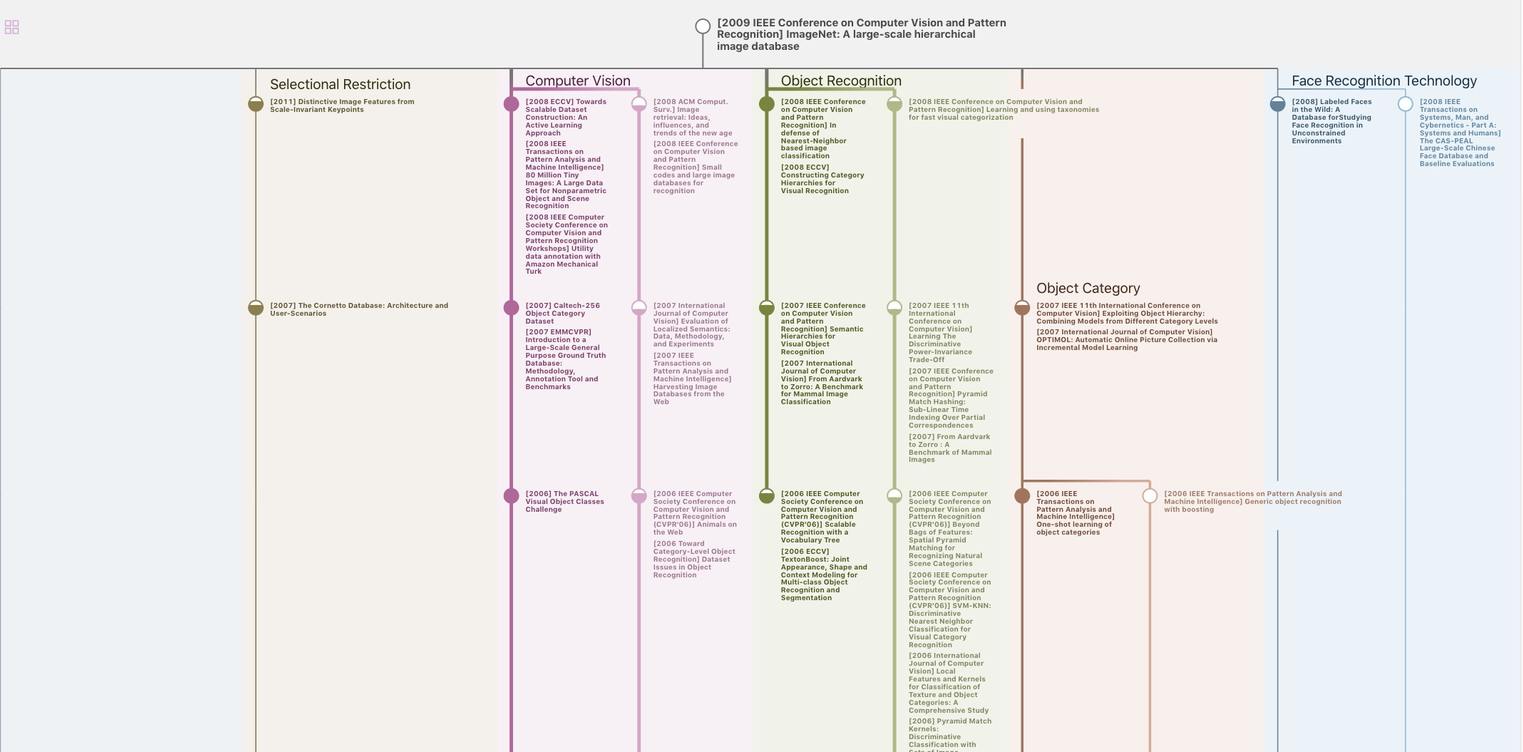
生成溯源树,研究论文发展脉络
Chat Paper
正在生成论文摘要