LSTM-Autoencoder based Anomaly Detection for Indoor Air Quality Time Series Data
arxiv(2022)
摘要
Anomaly detection for indoor air quality (IAQ) data has become an important area of research as the quality of air is closely related to human health and well-being. However, traditional statistics and shallow machine learning-based approaches in anomaly detection in the IAQ area could not detect anomalies involving the observation of correlations across several data points (i.e., often referred to as long-term dependences). We propose a hybrid deep learning model that combines LSTM with Autoencoder for anomaly detection tasks in IAQ to address this issue. In our approach, the LSTM network is comprised of multiple LSTM cells that work with each other to learn the long-term dependences of the data in a time-series sequence. Autoencoder identifies the optimal threshold based on the reconstruction loss rates evaluated on every data across all time-series sequences. Our experimental results, based on the Dunedin CO2 time-series dataset obtained through a real-world deployment of the schools in New Zealand, demonstrate a very high and robust accuracy rate (99.50%) that outperforms other similar models.
更多查看译文
关键词
Anomaly detection,autoencoder (AE),CO₂,indoor air quality (IAQ),long short-term memory (LSTM),time series
AI 理解论文
溯源树
样例
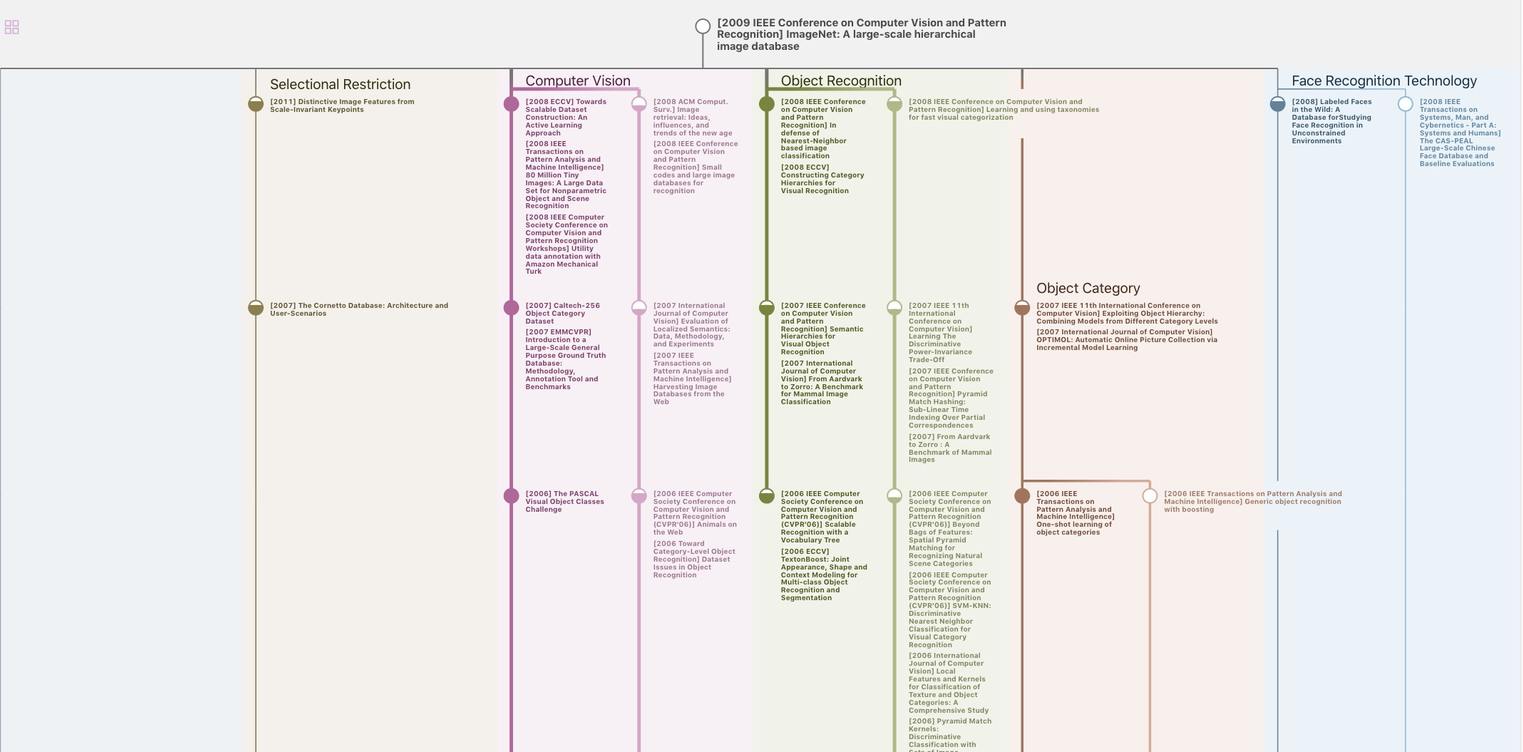
生成溯源树,研究论文发展脉络
Chat Paper
正在生成论文摘要