A Distant Supervision Corpus for Extracting Biomedical Relationships Between Chemicals, Diseases and Genes.
International Conference on Language Resources and Evaluation (LREC)(2022)
摘要
We introduce ChemDisGene, a new dataset for training and evaluating multi-class multi-label document-level biomedical relation extraction models. Our dataset contains 80k biomedical research abstracts labeled with mentions of chemicals, diseases, and genes, portions of which human experts labeled with 18 types of biomedical relationships between these entities (intended for evaluation), and the remainder of which (intended for training) has been distantly labeled via the CTD database with approximately 78\% accuracy. In comparison to similar preexisting datasets, ours is both substantially larger and cleaner; it also includes annotations linking mentions to their entities. We also provide three baseline deep neural network relation extraction models trained and evaluated on our new dataset.
更多查看译文
关键词
Corpus, Information Extraction, Linked Data, Weakly-supervised Learning, Relation Extraction
AI 理解论文
溯源树
样例
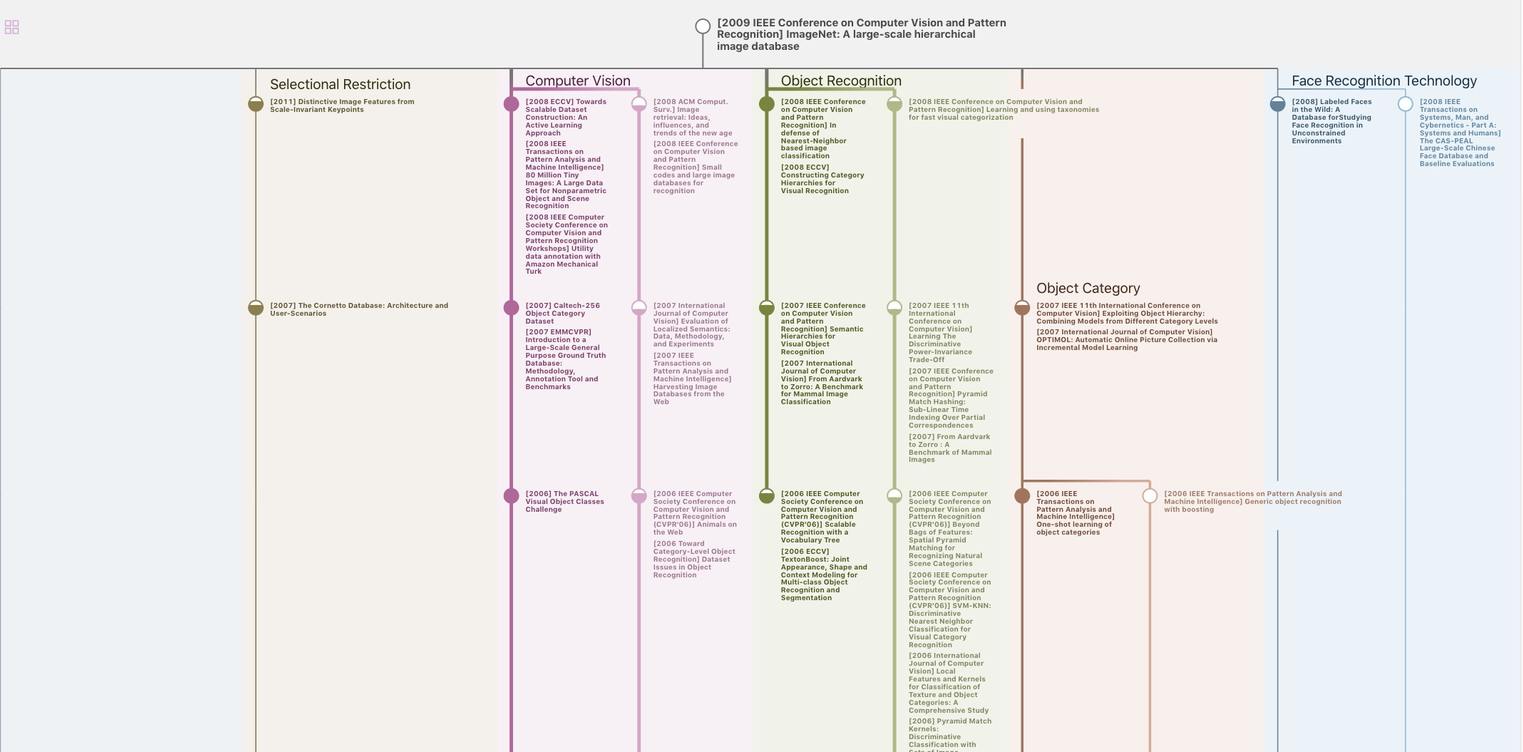
生成溯源树,研究论文发展脉络
Chat Paper
正在生成论文摘要