A visual deep learning model to predict abnormal versus normal parathyroid glands using intraoperative autofluorescence signals
JOURNAL OF SURGICAL ONCOLOGY(2022)
摘要
Background Previous work demonstrated that abnormal versus normal parathyroid glands (PGs) exhibit different patterns of autofluorescence, with former appearing darker and more heterogenous. Our objective was to develop a visual artificial intelligence model using intraoperative autofluorescence signals to predict whether a PG is abnormal (hypersecreting and/or hypercellular) or normal before excision during surgical exploration for primary hyperparathyroidism. Methods A total of 906 intraoperative parathyroid autofluorescence images of 303 patients undergoing parathyroidectomy/thyroidectomy were used to develop model. Autofluorescence image of each PG was uploaded into the visual artificial intelligence platform as abnormal or normal. For deep learning, randomly chosen 80% of data was used for training, 10% for testing, 10% for validation. The area under the receiver operating characteristic (AUROC), area under the precision-recall curve (AUPRC), recall (sensitivity), and precision (positive predictive value) of the model were calculated. Results AUROC and AUPRC of the model to predict normal and abnormal PGs were 0.90 and 0.93, respectively. Recall and precision of the model were 89% each. Conclusion Visual artificial intelligence platforms may be used to compare the autofluorescence signal of a given parathyroid gland against a large database. This may be a new adjunctive tool for intraoperative assessment of parathyroid glands during surgical exploration for primary hyperparathyroidism.
更多查看译文
关键词
artificial intelligence, machine learning, parathyroid neoplasms
AI 理解论文
溯源树
样例
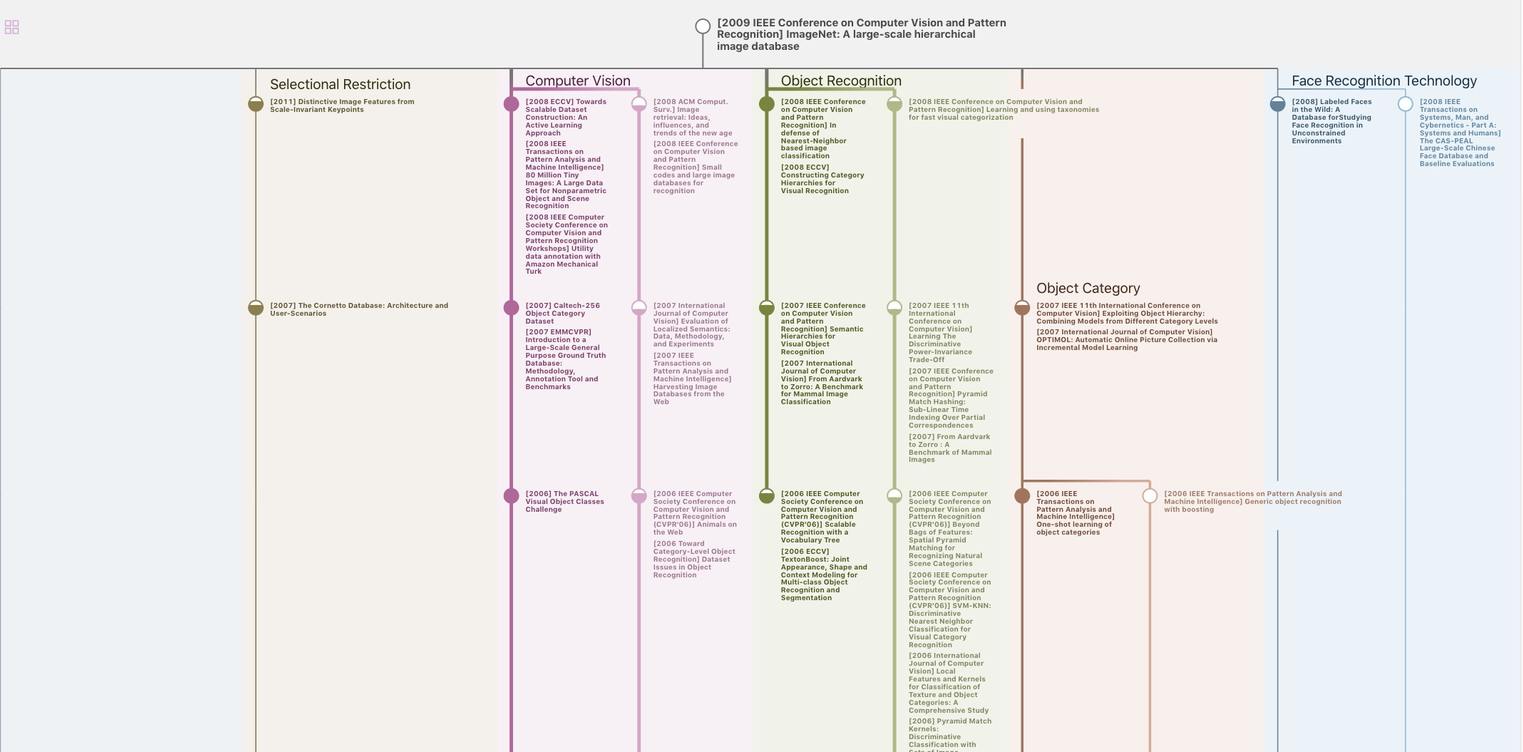
生成溯源树,研究论文发展脉络
Chat Paper
正在生成论文摘要