Conditional Image Generation by Conditioning Variational Auto-Encoders
International Conference on Learning Representations (ICLR)(2022)
摘要
We present a conditional variational auto-encoder (VAE) which, to avoid the substantial cost of training from scratch, uses an architecture and training objective capable of leveraging a foundation model in the form of a pretrained unconditional VAE. To train the conditional VAE, we only need to train an artifact to perform amortized inference over the unconditional VAE's latent variables given a conditioning input. We demonstrate our approach on tasks including image inpainting, for which it outperforms state-of-the-art GAN-based approaches at faithfully representing the inherent uncertainty. We conclude by describing a possible application of our inpainting model, in which it is used to perform Bayesian experimental design for the purpose of guiding a sensor.
更多查看译文
关键词
variational auto-encoders,Bayesian inference,variational inference,amortized inference,image completion
AI 理解论文
溯源树
样例
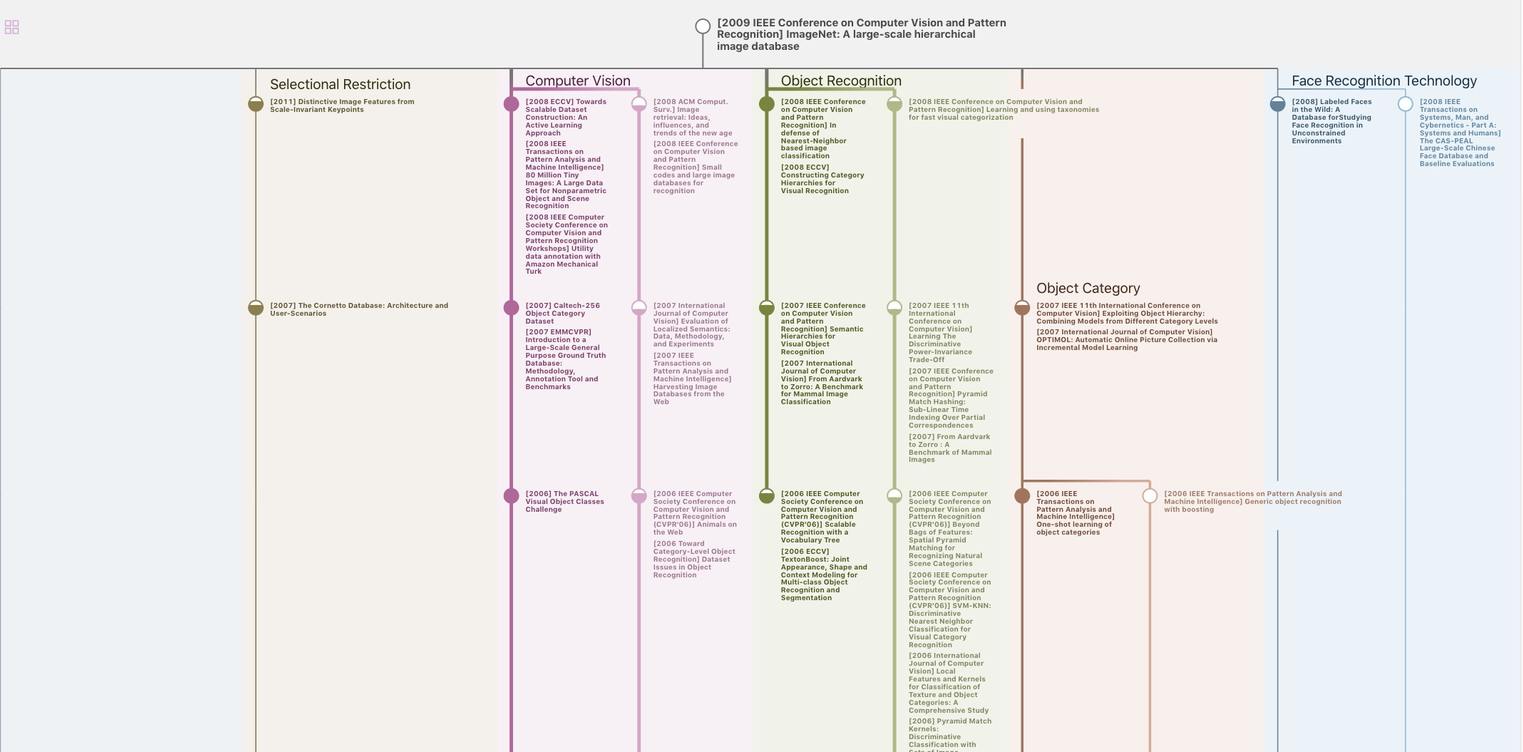
生成溯源树,研究论文发展脉络
Chat Paper
正在生成论文摘要