Tuformer: Data-driven Design of Transformers for Improved Generalization or Efficiency
International Conference on Learning Representations (ICLR)(2022)
摘要
Transformers are neural network architectures that achieve remarkable performance in many areas. However, the core component of Transformers, multi-head self-attention (MHSA), is mainly derived from heuristics, and the interactions across its components are not well understood. To address the problem, we first introduce a mathematically rigorous and yet intuitive tensor diagram representation of MHSA. Guided by tensor diagram representations, we propose a novel design, namely Tunable Transformers (Tuformers), by allowing data-driven weights across heads, whereas MHSA adopts pre-defined and fixed weights across heads, as will be explained in our paper. Tuformers naturally reveal a flexible design space that a user, depending on the needs, can choose a structure that has either improved performance (generalization error) or higher model efficiency. Any pre-trained Transformer can be an initialization of the corresponding Tuformer with trainable number of heads for efficient training and fine-tuning. Tuformers universally outperform Transformers on various tasks across multiple domains under a wide range of model sizes.
更多查看译文
关键词
Attention Modules,Transformers,Data-driven Model Design,Trainable Heads,Expressive Power,Tensor Methods.
AI 理解论文
溯源树
样例
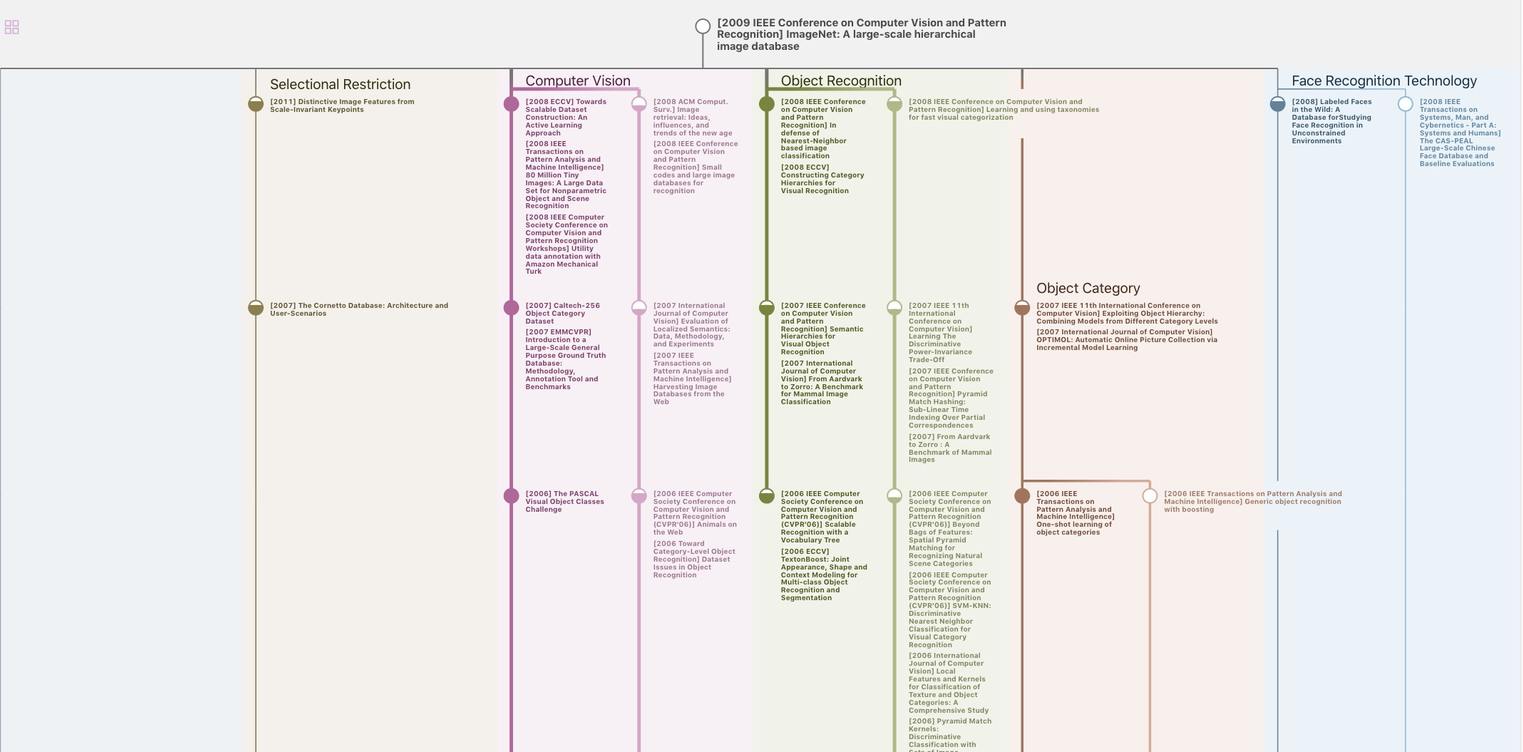
生成溯源树,研究论文发展脉络
Chat Paper
正在生成论文摘要