Communication Efficient Curvature Aided Primal-Dual Algorithms for Decentralized Optimization
IEEE TRANSACTIONS ON AUTOMATIC CONTROL(2023)
摘要
This article presents a family of algorithms for decentralized convex composite problems. We consider the setting of a network of agents that cooperatively minimize a global objective function composed of a sum of local functions plus a regularizer. Through the use of intermediate consensus variables, we remove the need for inner communication loops between agents when computing curvature-guided updates. A general scheme is presented, which unifies the analysis for a plethora of computing choices, including gradient descent, Newton updates, and Broyden, Fletcher, Goldfarb, and Shanno updates. Our analysis establishes sublinear convergence rates under convex objective functions with Lipschitz continuous gradients, as well as linear convergence rates when the local functions are further assumed to be strongly convex. Moreover, we explicitly characterize the acceleration due to curvature information. Last but not the least, we present an asynchronous implementation for the proposed algorithms, which removes the need for a central clock, with linear convergence rates established in expectation under strongly convex objectives. We ascertain the effectiveness of the proposed methods with numerical experiments on benchmark datasets.
更多查看译文
关键词
Asynchronous algorithms,control,decentralized optimization,network analysis,primal-dual algorithms.
AI 理解论文
溯源树
样例
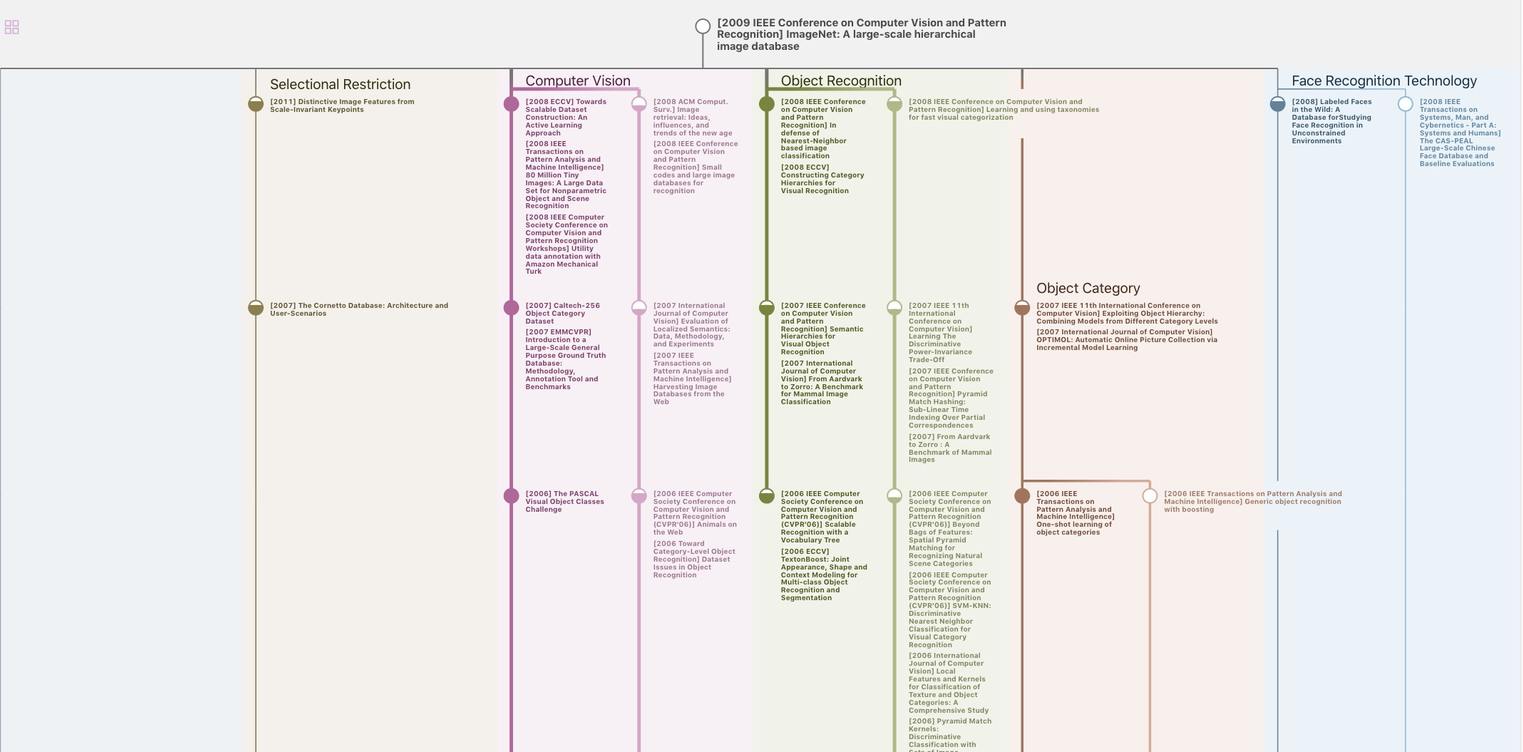
生成溯源树,研究论文发展脉络
Chat Paper
正在生成论文摘要