Probing for Constituency Structure in Neural Language Models
arxiv(2022)
摘要
In this paper, we investigate to which extent contextual neural language models (LMs) implicitly learn syntactic structure. More concretely, we focus on constituent structure as represented in the Penn Treebank (PTB). Using standard probing techniques based on diagnostic classifiers, we assess the accuracy of representing constituents of different categories within the neuron activations of a LM such as RoBERTa. In order to make sure that our probe focuses on syntactic knowledge and not on implicit semantic generalizations, we also experiment on a PTB version that is obtained by randomly replacing constituents with each other while keeping syntactic structure, i.e., a semantically ill-formed but syntactically well-formed version of the PTB. We find that 4 pretrained transfomer LMs obtain high performance on our probing tasks even on manipulated data, suggesting that semantic and syntactic knowledge in their representations can be separated and that constituency information is in fact learned by the LM. Moreover, we show that a complete constituency tree can be linearly separated from LM representations.
更多查看译文
关键词
constituency structure,language,models
AI 理解论文
溯源树
样例
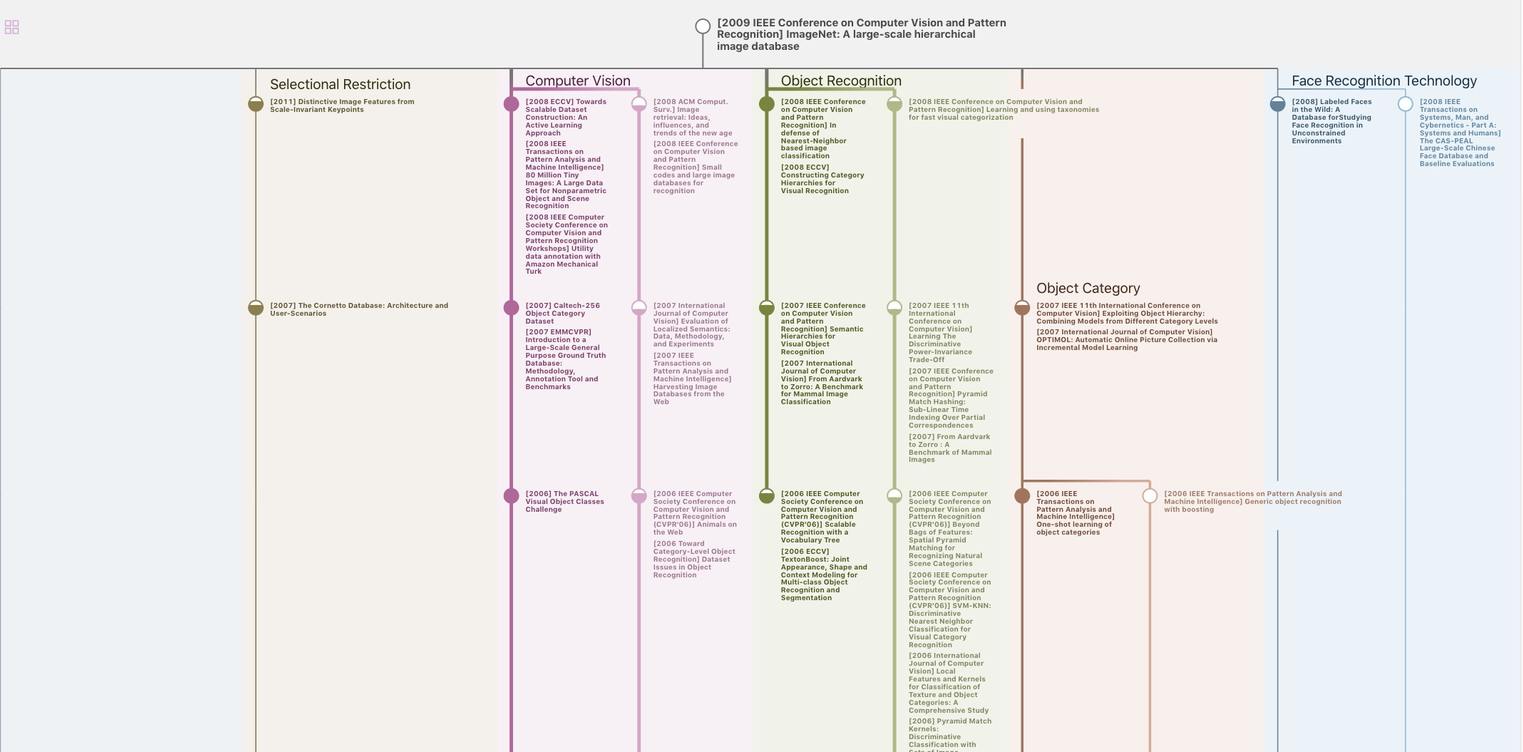
生成溯源树,研究论文发展脉络
Chat Paper
正在生成论文摘要