SRMD: Sparse Random Mode Decomposition
arxiv(2023)
摘要
Signal decomposition and multiscale signal analysis provide many useful tools for time-frequency analysis. We proposed a random feature method for analyzing time-series data by constructing a sparse approximation to the spectrogram. The randomization is both in the time window locations and the frequency sampling, which lowers the overall sampling and computational cost. The sparsification of the spectrogram leads to a sharp separation between time-frequency clusters which makes it easier to identify intrinsic modes, and thus leads to a new data-driven mode decomposition. The applications include signal representation, outlier removal, and mode decomposition. On benchmark tests, we show that our approach outperforms other state-of-the-art decomposition methods.
更多查看译文
关键词
mode,decomposition
AI 理解论文
溯源树
样例
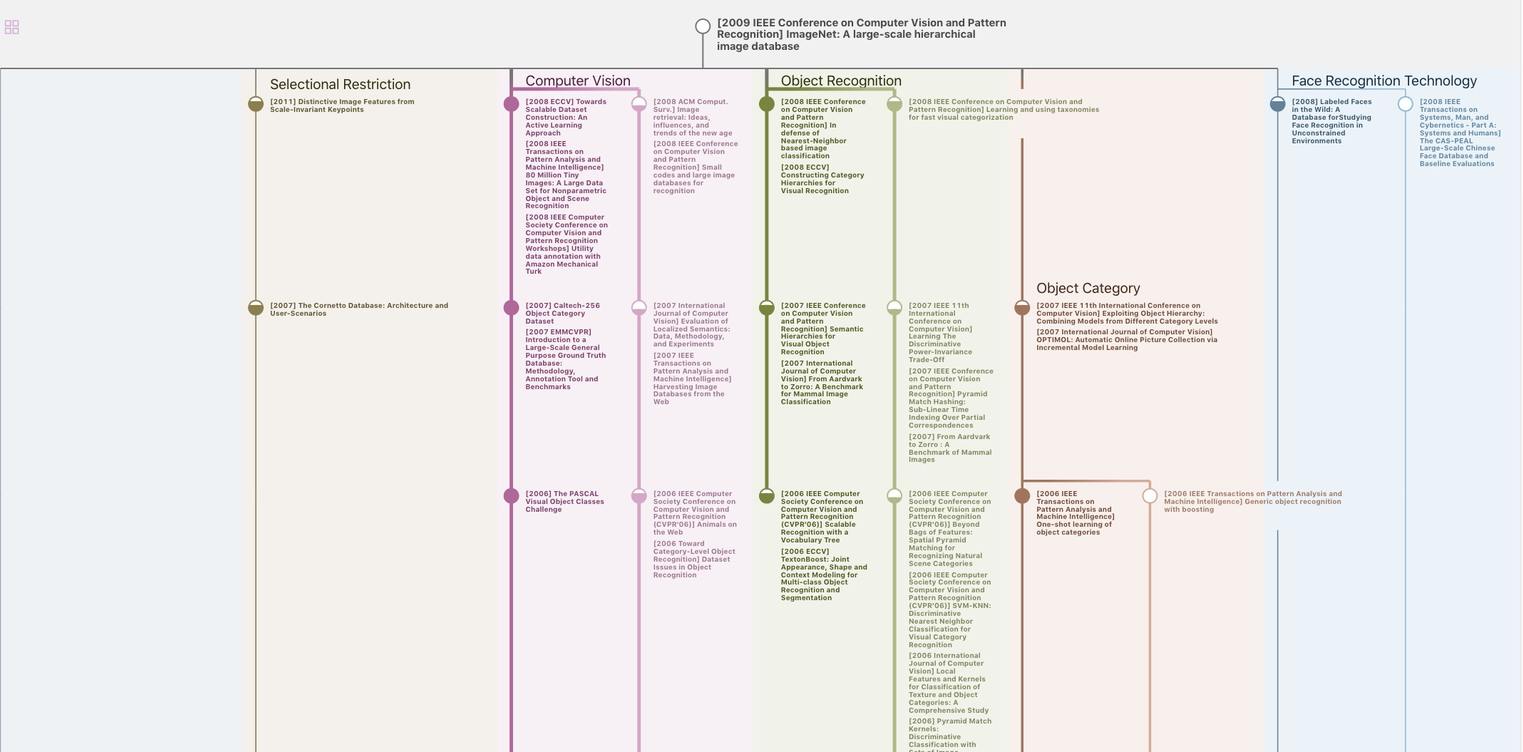
生成溯源树,研究论文发展脉络
Chat Paper
正在生成论文摘要