Enhanced Conformational Sampling with an Adaptive Coarse-Grained Elastic Network Model Using Short-Time All-Atom Molecular Dynamics br
JOURNAL OF CHEMICAL THEORY AND COMPUTATION(2022)
摘要
Compared to all-atom molecular dynamics (AA-MD) simulations, coarse-grained (CG) MD simulations can significantly reduce calculation costs. However, existing CG-MDmethods are unsuitable for sampling structures that departsignificantly from the initial structure without any biased force.In this study, we developed a new adaptive CG elastic networkmodel (ENM), in which the dynamic cross-correlation coefficientbased on short-time AA-MD of at most ns order is considered. Byapplying Bayesian optimization to search for a suitable parameteramong the vast parameter space of adaptive CG-ENM, wesucceeded in reducing the searching cost to approximately 10% of those for random sampling and exhaustive sampling. Toevaluate the performance of adaptive CG-ENM, we applied the new methodology to adenylate kinase (ADK) and glutamine bindingprotein (GBP) in the apo state. The results showed that the structural ensembles explored by adaptive CG-ENM could beconsiderably more diverse than those by conventional ENMs with enhanced sampling such as temperature replica exchange MD andlong-time AA-MD of 1 mu s. In particular, some of the structures sampled by adaptive ENM are relatively close to the holo-types tructures of ADK and GBP. Furthermore, as a challenging task, to demonstrate the advantages of the CG model with lower calculation cost, we applied our new methodology to a larger biomolecule, integrin (alpha V) in the inactive state. Then, we sampled various structural ensembles, including extended structures that are apparently different from inactive ones.
更多查看译文
AI 理解论文
溯源树
样例
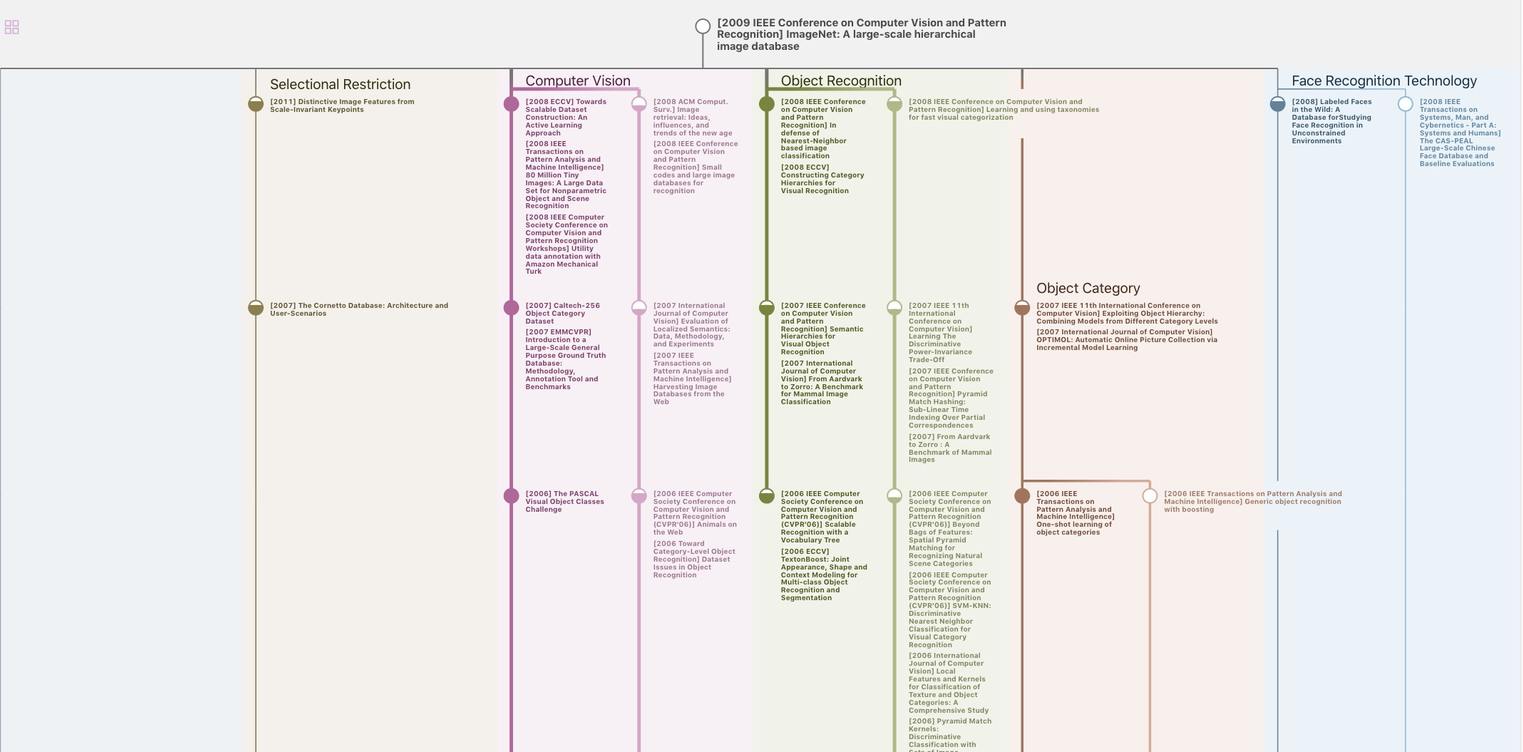
生成溯源树,研究论文发展脉络
Chat Paper
正在生成论文摘要