An attention-constrained neural network with overall cognition for landslide spatial prediction
Landslides(2022)
摘要
The occurrence of landslides is affected by various environmental factors. When predicting landslides, conventional neural networks optimize parameters using global connectivity, which limits their efficiency in extracting features of contributing factors. In this study, we developed an attention-constrained neural network with overall cognition (OC-ACNN) to focus on important features from the complex data. The method has four steps: (1) extract the overall cognition as the prior input based on historical landslide distribution and contributing factors, (2) embed an attention mechanism in hidden layers to allocate more weight to noteworthy features, (3) update weights and fit the nonlinear relationship by the back-propagation neural network (BPNN), and (4) generate prediction results using a classifier. This model was applied to the Sichuan-Tibet Highway, considering 10 predisposing factors and 1449 historical landslides. The evaluation results indicate that OC-ACNN (0.822) had a higher predictive capability than multiple linear regression (MLR, 0.734) and BPNN (0.789) in terms of the area under the receiver operating characteristic curve (AUC). Further, we compared different attention patterns and score functions for use with the proposed model. The results show that OC-ACNN offered greater predictive performance than Self-ACNN (without OC, 0.803) and that the improved cosine (0.822) score function had better results and stability than others (0.819 highest).
更多查看译文
关键词
Landslide spatial prediction,Attention model,Machine learning,Sichuan-Tibet Highway
AI 理解论文
溯源树
样例
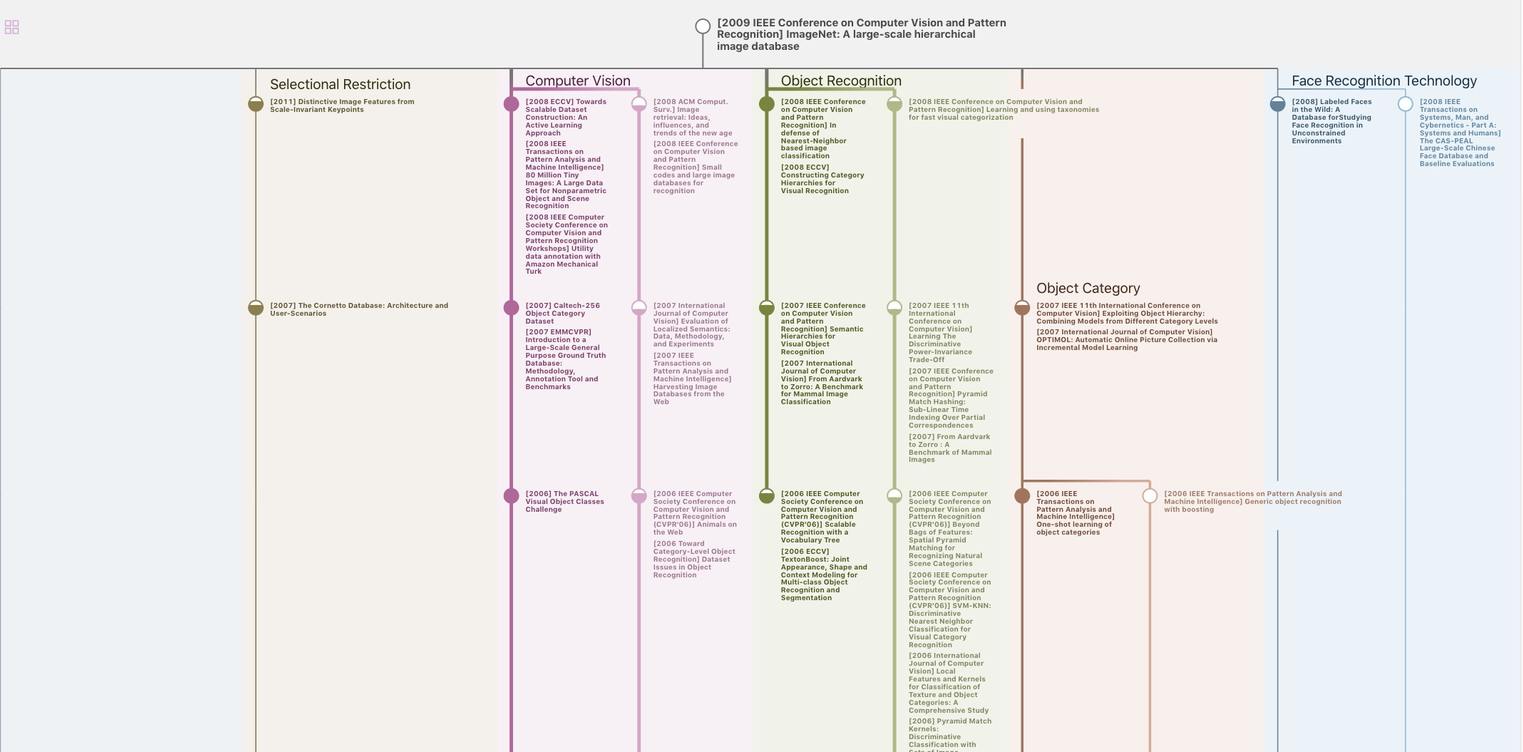
生成溯源树,研究论文发展脉络
Chat Paper
正在生成论文摘要