Self-Supervised Losses for One-Class Textual Anomaly Detection
arxiv(2022)
摘要
Current deep learning methods for anomaly detection in text rely on supervisory signals in inliers that may be unobtainable or bespoke architectures that are difficult to tune. We study a simpler alternative: fine-tuning Transformers on the inlier data with self-supervised objectives and using the losses as an anomaly score. Overall, the self-supervision approach outperforms other methods under various anomaly detection scenarios, improving the AUROC score on semantic anomalies by 11.6% and on syntactic anomalies by 22.8% on average. Additionally, the optimal objective and resultant learnt representation depend on the type of downstream anomaly. The separability of anomalies and inliers signals that a representation is more effective for detecting semantic anomalies, whilst the presence of narrow feature directions signals a representation that is effective for detecting syntactic anomalies.
更多查看译文
关键词
anomaly,textual,detection,self-supervised,one-class
AI 理解论文
溯源树
样例
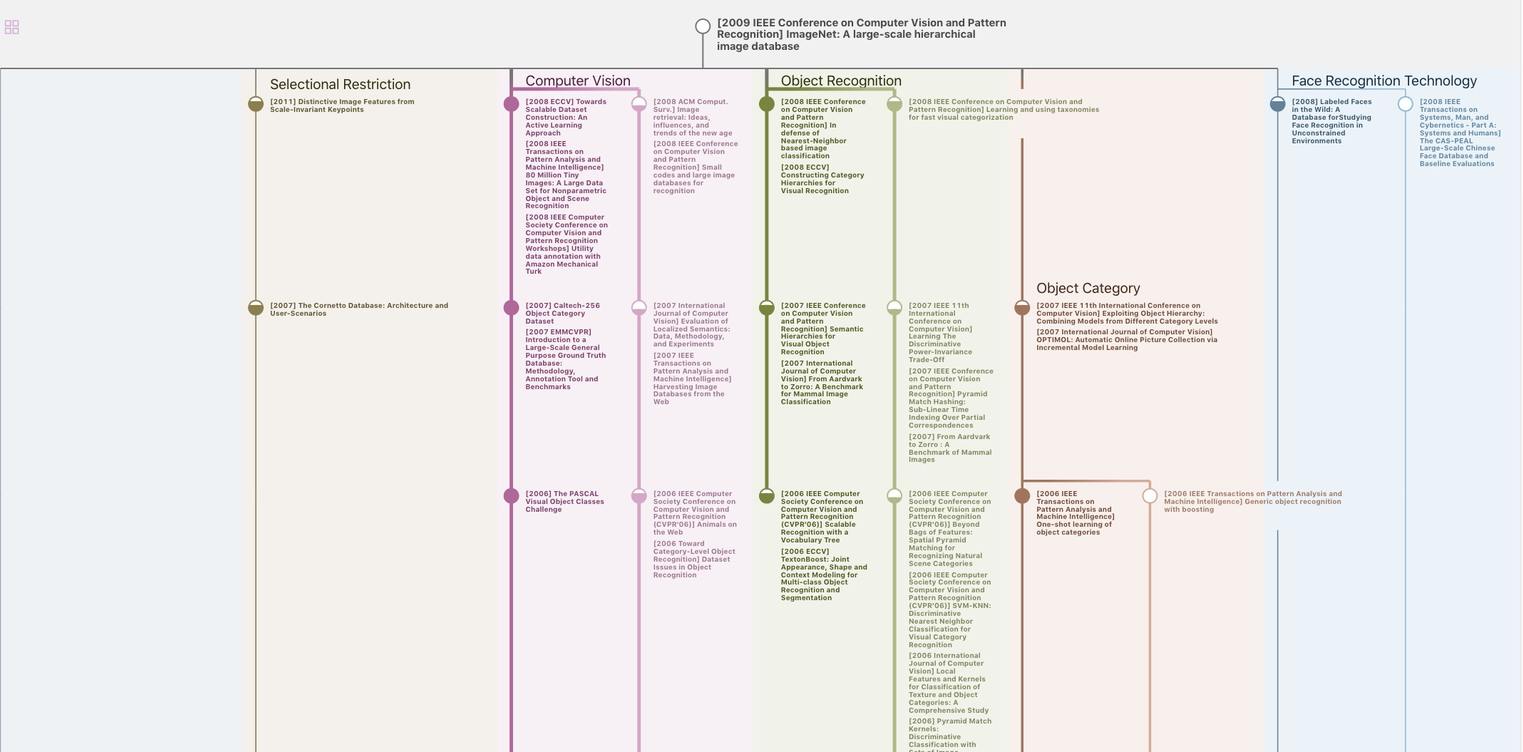
生成溯源树,研究论文发展脉络
Chat Paper
正在生成论文摘要