medXGAN: Visual Explanations for Medical Classifiers through a Generative Latent Space.
IEEE Conference on Computer Vision and Pattern Recognition(2022)
摘要
Despite the surge of deep learning in the past decade, some users are skeptical to deploy these models in practice due to their black-box nature. Specifically, in the medical space where there are severe potential repercussions, we need to develop methods to gain confidence in the models' decisions. To this end, we propose a novel medical imaging generative adversarial framework, medXGAN (medical eXplanation GAN), to visually explain what a medical classifier focuses on in its binary predictions. By encoding domain knowledge of medical images, we are able to disentangle anatomical structure and pathology, leading to fine-grained visualization through latent interpolation. Furthermore, we optimize the latent space such that interpolation explains how the features contribute to the classifier's output. Our method outperforms baselines such as Gradient-Weighted Class Activation Mapping (Grad-CAM) and Integrated Gradients in localization and explanatory ability. Additionally, a combination of the medXGAN with Integrated Gradients can yield explanations more robust to noise. The code is available at: https://github.com/avdravid/medXGAN_explanations.
更多查看译文
关键词
visual explanations,medical classifiers,latent space
AI 理解论文
溯源树
样例
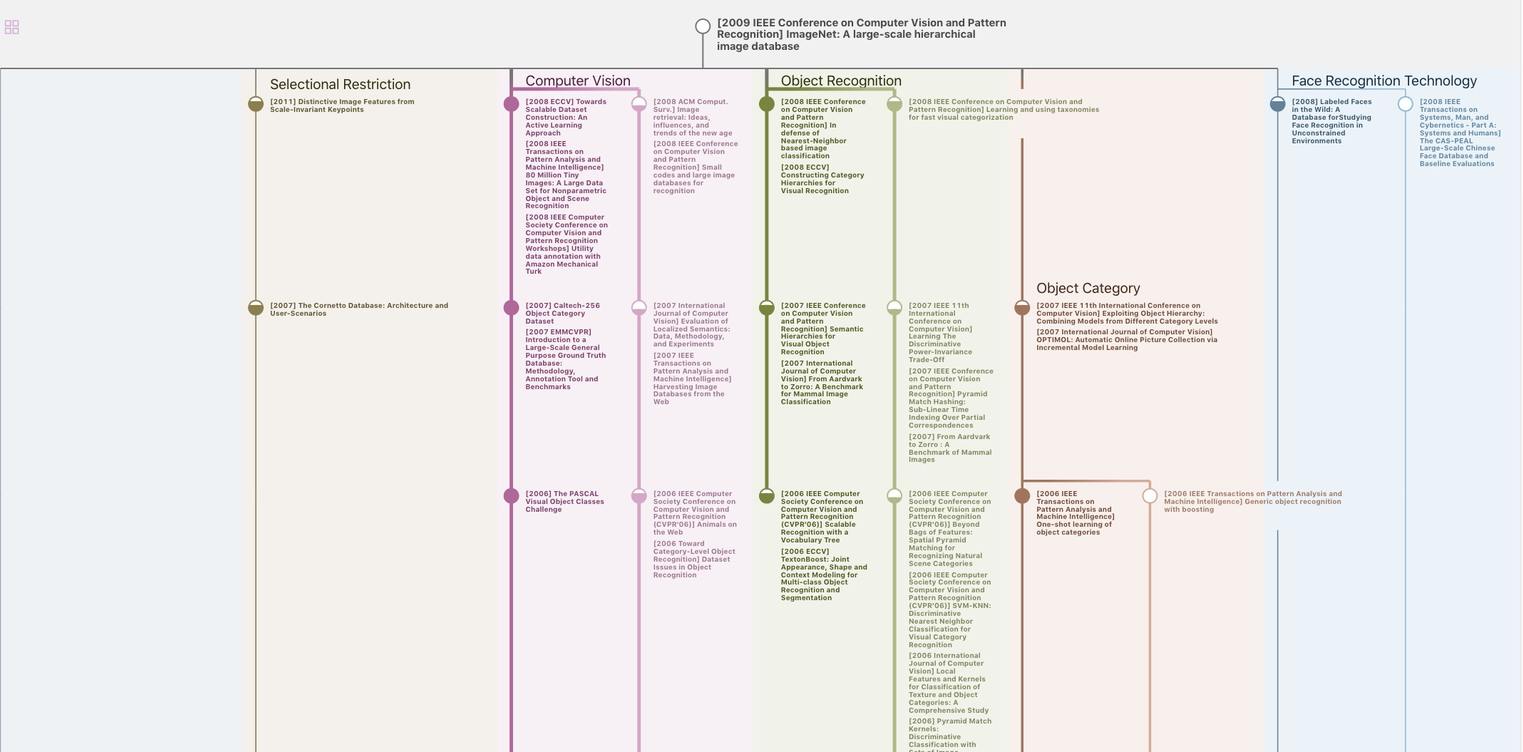
生成溯源树,研究论文发展脉络
Chat Paper
正在生成论文摘要