Segmentation-Consistent Probabilistic Lesion Counting
arxiv(2022)
摘要
Lesion counts are important indicators of disease severity, patient prognosis, and treatment efficacy, yet counting as a task in medical imaging is often overlooked in favor of segmentation. This work introduces a novel continuously differentiable function that maps lesion segmentation predictions to lesion count probability distributions in a consistent manner. The proposed end-to-end approach--which consists of voxel clustering, lesion-level voxel probability aggregation, and Poisson-binomial counting--is non-parametric and thus offers a robust and consistent way to augment lesion segmentation models with post hoc counting capabilities. Experiments on Gadolinium-enhancing lesion counting demonstrate that our method outputs accurate and well-calibrated count distributions that capture meaningful uncertainty information. They also reveal that our model is suitable for multi-task learning of lesion segmentation, is efficient in low data regimes, and is robust to adversarial attacks.
更多查看译文
关键词
lesion,counting,segmentation-consistent
AI 理解论文
溯源树
样例
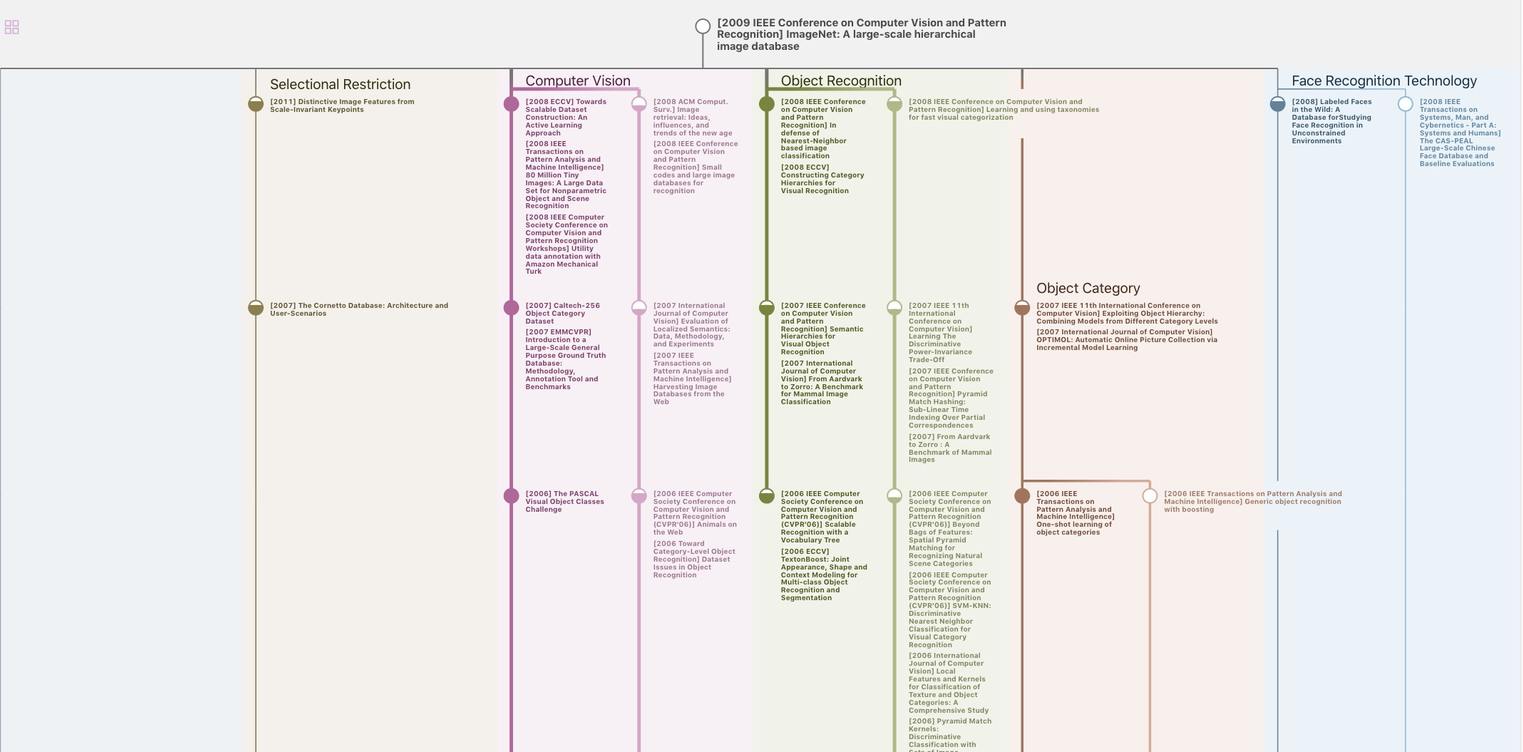
生成溯源树,研究论文发展脉络
Chat Paper
正在生成论文摘要