NeuS: Neutral Multi-News Summarization for Mitigating Framing Bias
North American Chapter of the Association for Computational Linguistics (NAACL)(2022)
摘要
Media framing bias can lead to increased political polarization, and thus, the need for automatic mitigation methods is growing. We propose a new task, a neutral summary generation from multiple news headlines of the varying political spectrum, to facilitate balanced and unbiased news reading. In this paper, we first collect a new dataset, obtain some insights about framing bias through a case study, and propose a new effective metric and models for the task. Lastly, we conduct experimental analyses to provide insights about remaining challenges and future directions. One of the most interesting observations is that generation models can hallucinate not only factually inaccurate or unverifiable content, but also politically biased content.
更多查看译文
AI 理解论文
溯源树
样例
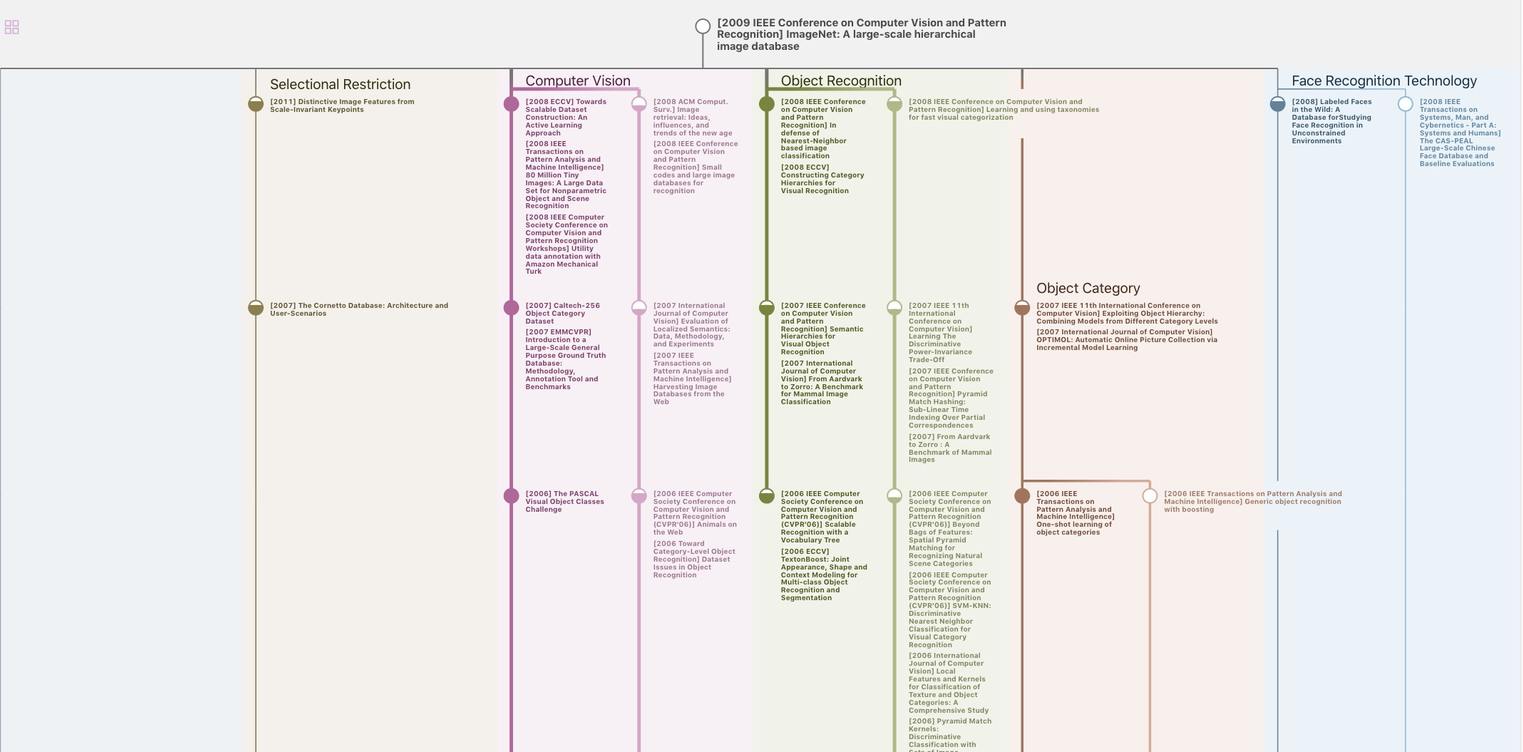
生成溯源树,研究论文发展脉络
Chat Paper
正在生成论文摘要