SOS! Self-supervised Learning over Sets of Handled Objects in Egocentric Action Recognition.
European Conference on Computer Vision(2022)
摘要
Learning an egocentric action recognition model from video data is challenging due to distractors in the background, e.g., irrelevant objects. Further integrating object information into an action model is hence beneficial. Existing methods often leverage a generic object detector to identify and represent the objects in the scene. However, several important issues remain. Object class annotations of good quality for the target domain (dataset) are still required for learning good object representation. Moreover, previous methods deeply couple existing action models with object representations, and thus need to retrain them jointly, leading to costly and inflexible integration. To overcome both limitations, we introduce Self-Supervised Learning Over Sets (SOS), an approach to pre-train a generic Objects In Contact (OIC) representation model from video object regions detected by an off-the-shelf hand-object contact detector. Instead of augmenting object regions individually as in conventional self-supervised learning, we view the action process as a means of natural data transformations with unique spatiotemporal continuity and exploit the inherent relationships among per-video object sets. Extensive experiments on two datasets, EPIC-KITCHENS-100 and EGTEA, show that our OIC significantly boosts the performance of multiple state-of-the-art video classification models.
更多查看译文
关键词
Handled objects,Egocentric action recognition,Self-supervised pre-training over sets,Long-tail setup
AI 理解论文
溯源树
样例
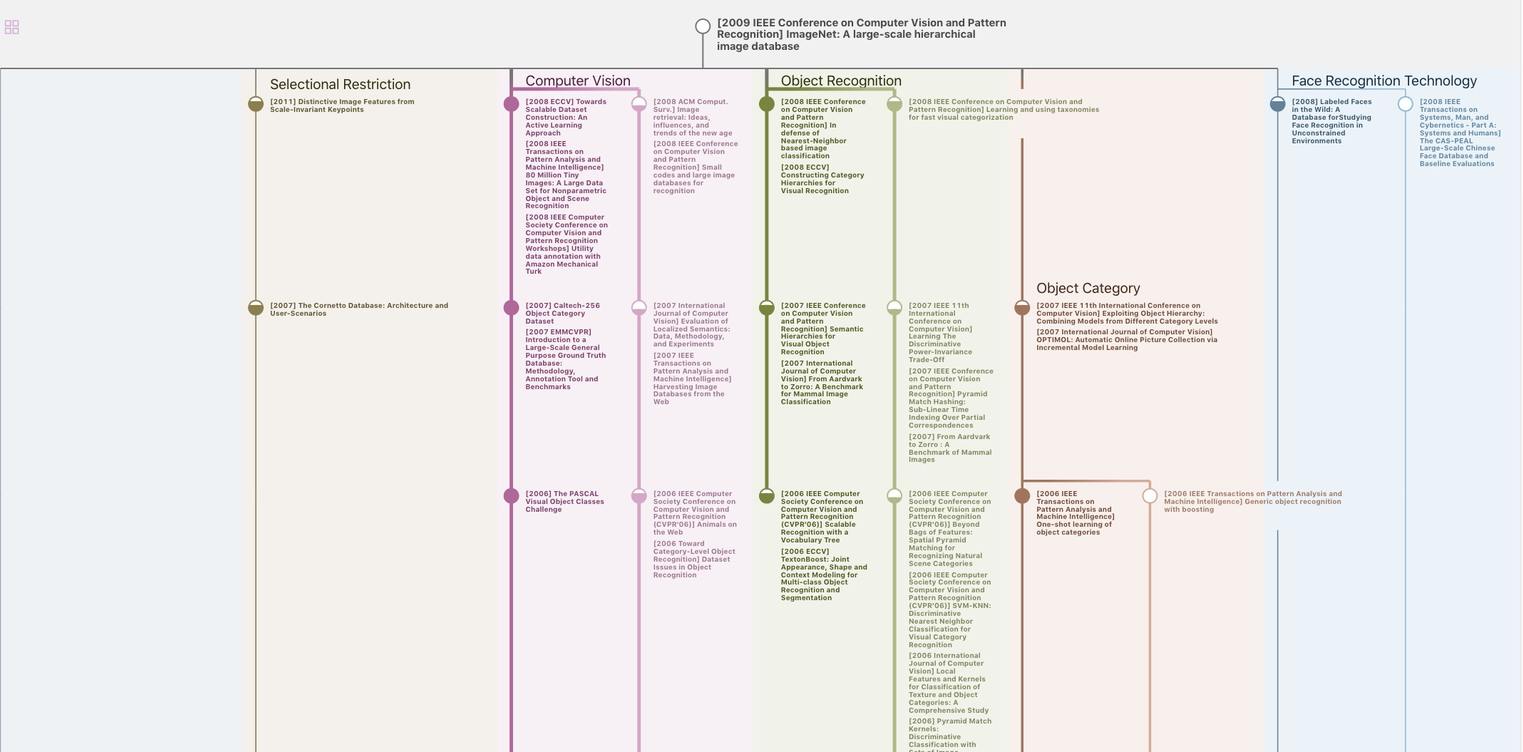
生成溯源树,研究论文发展脉络
Chat Paper
正在生成论文摘要