Information-theoretic Online Memory Selection for Continual Learning
International Conference on Learning Representations (ICLR)(2022)
摘要
A challenging problem in task-free continual learning is the online selection of a representative replay memory from data streams. In this work, we investigate the online memory selection problem from an information-theoretic perspective. To gather the most information, we propose the \textit{surprise} and the \textit{learnability} criteria to pick informative points and to avoid outliers. We present a Bayesian model to compute the criteria efficiently by exploiting rank-one matrix structures. We demonstrate that these criteria encourage selecting informative points in a greedy algorithm for online memory selection. Furthermore, by identifying the importance of \textit{the timing to update the memory}, we introduce a stochastic information-theoretic reservoir sampler (InfoRS), which conducts sampling among selective points with high information. Compared to reservoir sampling, InfoRS demonstrates improved robustness against data imbalance. Finally, empirical performances over continual learning benchmarks manifest its efficiency and efficacy.
更多查看译文
关键词
Task-free continual learning,replay memory,information theoretic,reservoir sampling
AI 理解论文
溯源树
样例
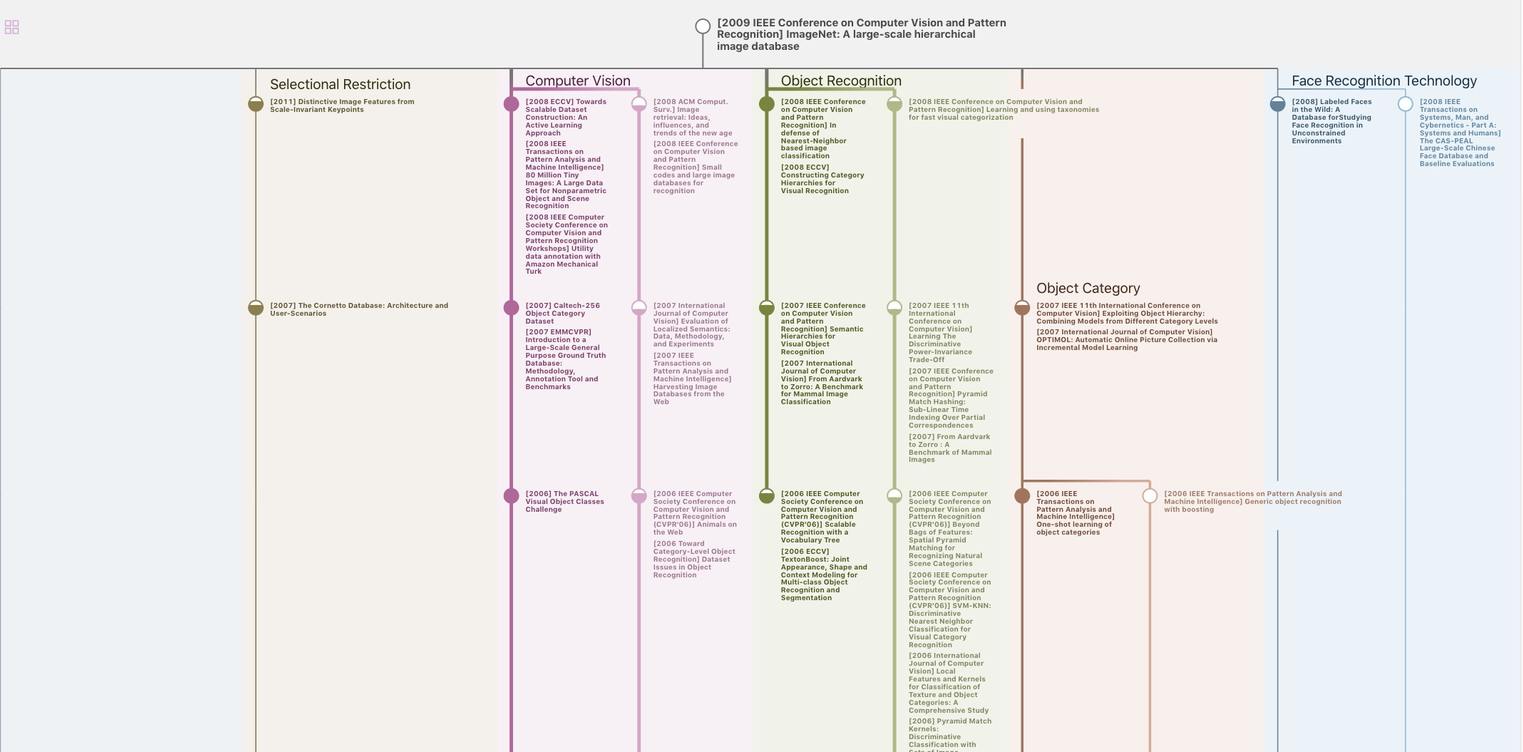
生成溯源树,研究论文发展脉络
Chat Paper
正在生成论文摘要